QTIAH-GNN: Quantity and Topology Imbalance-Aware Heterogeneous Graph Neural Network for Bankruptcy Prediction
KDD 2023(2023)
摘要
The timely prediction of bankruptcy is highly desirable to guarantee an upward spiral for overall societal well-being. By extracting multifaceted information from the business interaction networks, Graph Neural Networks (GNNs) may be able to automatically make more informed predictions for bankruptcy, as compared to methods that rely heavily on abundant manpower to a large extent. Yet in real applications, bankruptcy prediction faces the key issue of quantity-imbalance: data usually comes with a long-tailed distribution wherein bankrupt corporates occupy the least of the data proportion but are our target to be identified. Apart from that, the topology-imbalance issue behind graph-structural data exacerbates prediction deterioration: feature propagation is dominated by non-bankrupt nodes through messages passing between nodes; thus, bankrupt nodes receive highly confusing information and could be easily assimilated by nearby non-bankrupt nodes. Unfortunately, the existing GNN methods are not immune to these two imbalance issues. To tackle the challenging but practically useful scenario, we propose a novel bankruptcy prediction model called the Quantity and Topology Imbalance-Aware Heterogeneous Graph Neural Network (QTIAH-GNN) to boost the final performance. Specifically, QTIAH-GNN employs the multi-hierarchy label-aware neighbor selection to conquer the topology-imbalance issue by using the class-semantic representation and the learnable parameterized similarity metric, and employs the imbalance-oriented loss to obtain the optimal tradeoff between the accuracies of the majority and minority classes. In experiments, we evaluate the proposed QTIAH-GNN on two large-scale, real-world datasets. The results show that QTIAH-GNN outperforms other state-of-the-art baselines in terms of prediction accuracy with superior efficiency and generalization ability, has stronger robustness to data imbalance, and provides meaningful model interpretation.
更多查看译文
关键词
Bankruptcy prediction,Graph Neural Network (GNN),Quantity-imbalance,Topology-imbalance
AI 理解论文
溯源树
样例
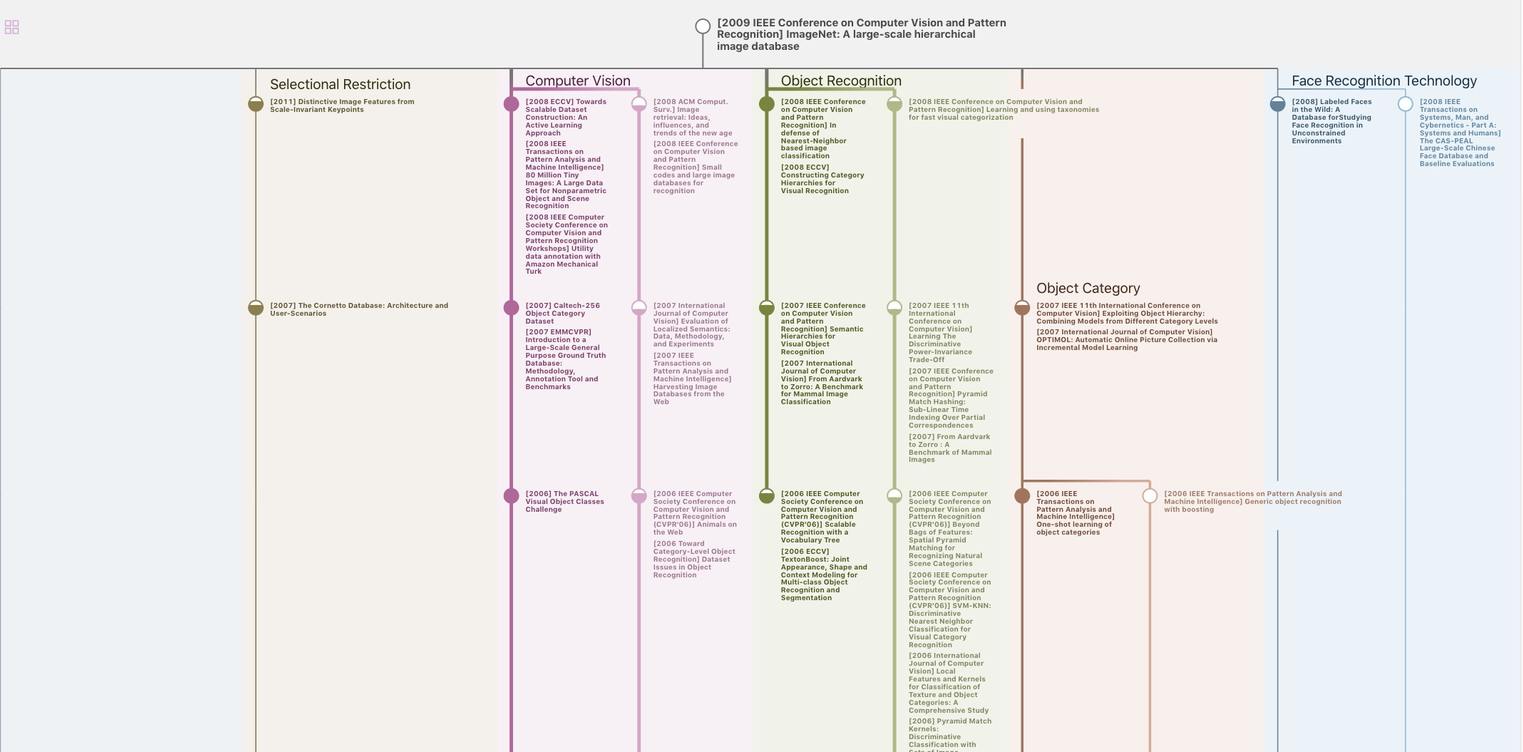
生成溯源树,研究论文发展脉络
Chat Paper
正在生成论文摘要