Learning-Based Ad Auction Design with Externalities: The Framework and A Matching-Based Approach.
KDD(2023)
摘要
Learning-based ad auctions have increasingly been adopted in online advertising. However, existing approaches neglect externalities, such as the interaction between ads and organic items. In this paper, we propose a general framework, namely Score-Weighted VCG, for designing learning-based ad auctions that account for externalities. The framework decomposes the optimal auction design into two parts: designing a monotone score function and an allocation algorithm, which facilitates data-driven implementation. Theoretical results demonstrate that this framework produces the optimal incentive-compatible and individually rational ad auction under various externality-aware CTR models while being data-efficient and robust. Moreover, we present an approach to implement the proposed framework with a matching-based allocation algorithm. Experiment results on both real-world and synthetic data illustrate the effectiveness of the proposed approach.
更多查看译文
关键词
Learning-Based Mechanism Design,Multi-slot Ad Auction,Externality,Online Advertising
AI 理解论文
溯源树
样例
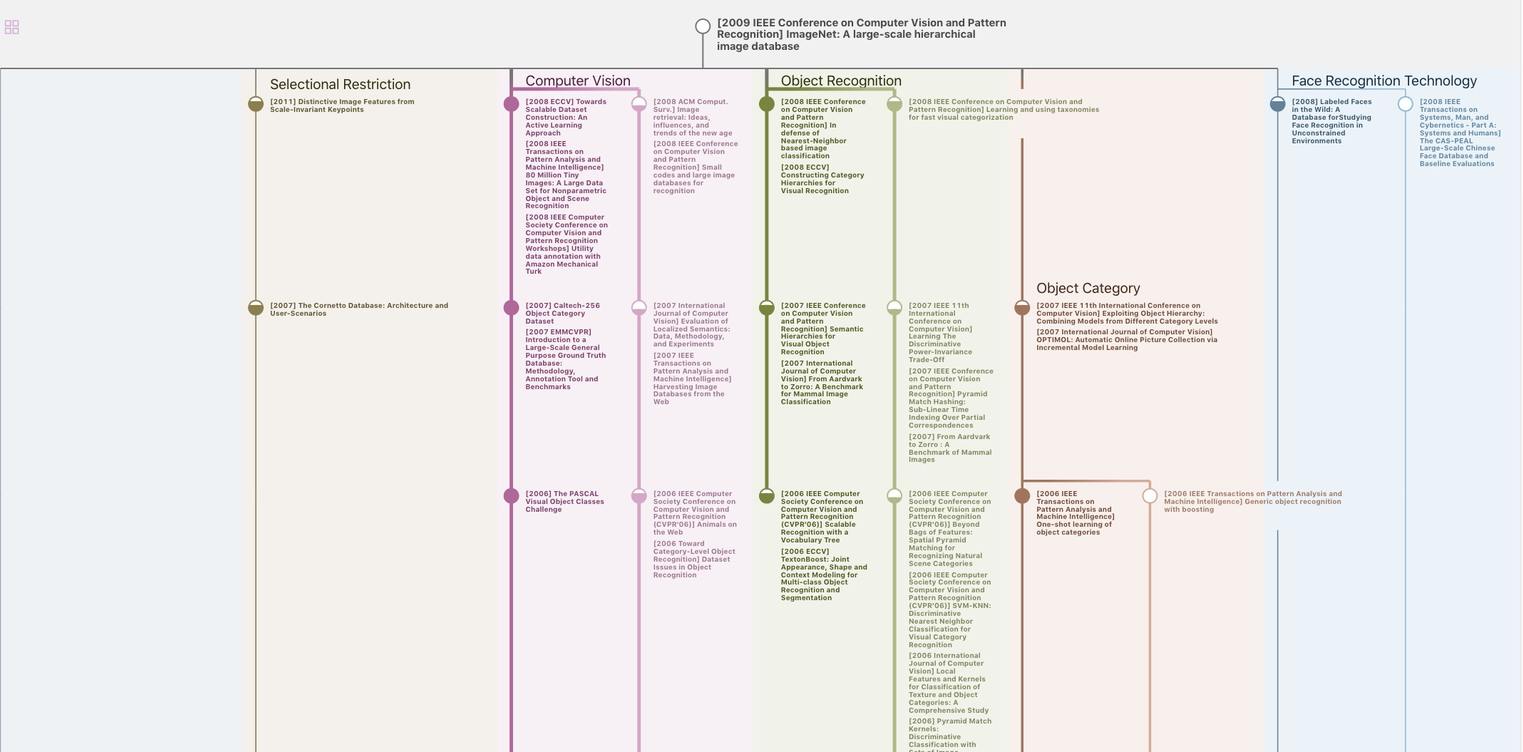
生成溯源树,研究论文发展脉络
Chat Paper
正在生成论文摘要