WinGNN: Dynamic Graph Neural Networks with Random Gradient Aggregation Window.
KDD(2023)
摘要
Modeling the dynamics into graph neural networks (GNNs) contributes to the understanding of evolution in dynamic graphs, which helps optimize temporal-spatial representations for real-world dynamic network problems. Empirically, dynamic GNN embedding requires additional temporal encoders, which inevitably introduces additional learning parameters to make dynamic GNNs oversized and inefficient. Furthermore, previous dynamic GNN models are under the same fixed temporal term, which causes the short-temporal optimum. To address these issues, we propose the WinGNN framework to model dynamic graphs, which is realized by a simple GNN model with the meta-learning strategy and a novel mechanism of random gradient aggregation. WinGNN calculates the frame-wise loss of the current snapshot and passes the loss gradient to the next to model graph dynamics without temporal encoders. Then it introduces the randomized sliding-window to acquire the window-aware gradienton consecutive snapshots, and the calculated two types of gradient are aggregated to update the GNN, thereby reducing the parameter size and improving the robustness. Experiments on six public datasets show the advantage of our WinGNN compared with existing baselines, where it has reached the optimum in twenty-two out of twenty-four performance metrics.
更多查看译文
关键词
Temporal encoder-free GNN,multi gradient aggregation,dynamic graph neural networks,dynamic graph representation learning
AI 理解论文
溯源树
样例
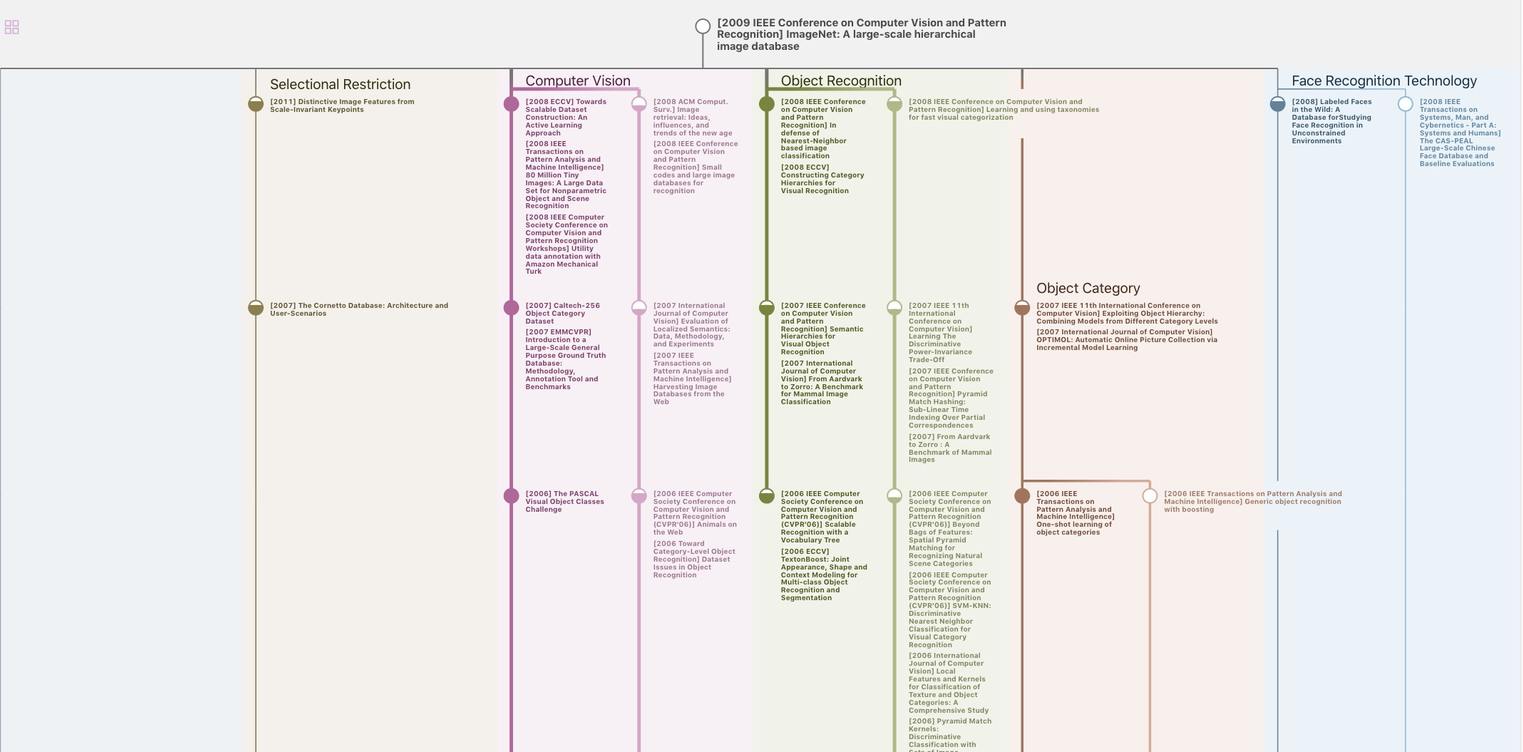
生成溯源树,研究论文发展脉络
Chat Paper
正在生成论文摘要