Contrastive Cross-scale Graph Knowledge Synergy.
KDD(2023)
Abstract
Graph representation learning via Contrastive Learning (GCL) has drawn considerable attention recently. Efforts are mainly focused on gathering more global information via contrasting on a single high-level graph view, which, however, underestimates the inherent complex and hierarchical properties in many real-world networks, leading to sub-optimal embeddings. To incorporate these properties of a complex graph, we propose Cross-Scale Contrastive Graph Knowledge Synergy (CGKS), a generic feature learning framework, to advance graph contrastive learning with enhanced generalization ability and the awareness of latent anatomies. Specifically, to maintain the hierarchical information, we create a so-call graph pyramid (GP) consisting of coarse-grained graph views. Each graph view is obtained via the careful design topology-aware graph coarsening layer that extends the Laplacian Eigenmaps with negative sampling. To promote cross-scale information sharing and knowledge interactions among GP, we propose a novel joint optimization formula that contains a pairwise contrastive loss between any two coarse-grained graph views. This synergy loss not only promotes knowledge sharing that yields informative representations, but also stabilizes the training process. Experiments on various downstream tasks demonstrate the substantial improvements of the proposed method over its counterparts.
MoreTranslated text
Key words
Graph Contrastive Learning,Graph Neural Networks
AI Read Science
Must-Reading Tree
Example
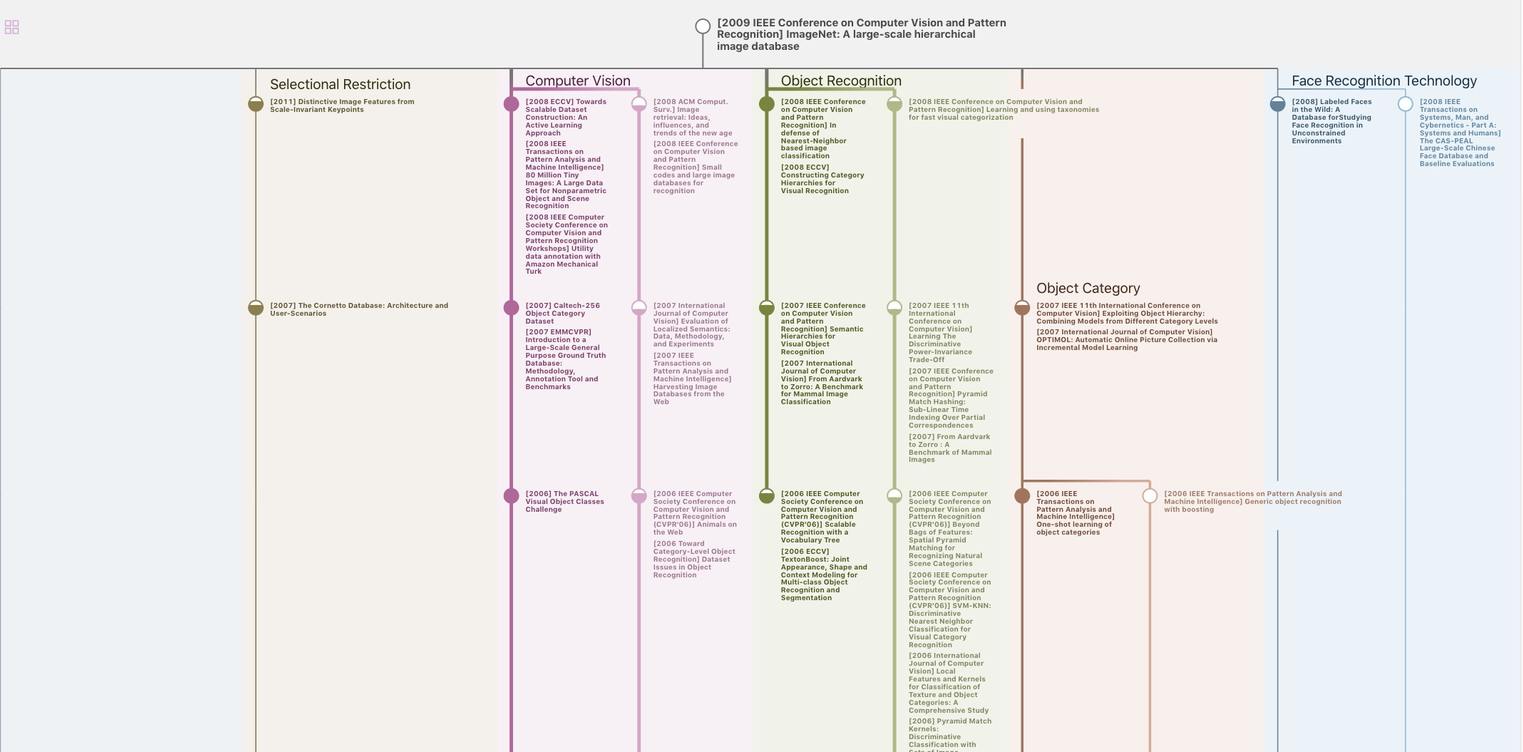
Generate MRT to find the research sequence of this paper
Chat Paper
Summary is being generated by the instructions you defined