Mp55-20 a novel machine learning framework for automated detection of prostate cancer lesions confirmed on mri-informed target biopsy
The Journal of Urology(2023)
摘要
You have accessJournal of UrologyCME1 Apr 2023MP55-20 A NOVEL MACHINE LEARNING FRAMEWORK FOR AUTOMATED DETECTION OF PROSTATE CANCER LESIONS CONFIRMED ON MRI-INFORMED TARGET BIOPSY Masatomo Kaneko, Giovanni E. Cacciamani, Vasileios Magoulianitis, Yijing Yang, Jintang Xue, Jiaxin Yang, Jinyuan Liu, Maria Sarah L. Lenon, Passant Mohamed, Darryl H. Hwang, Karan Gill, Manju Aron, Vinay Duddalwar, Suzanne L. Palmer, C.-C. Jay Kuo, Inderbir Gill, Andre Luis Abreu, and Chrysostomos L. Nikias Masatomo KanekoMasatomo Kaneko More articles by this author , Giovanni E. CacciamaniGiovanni E. Cacciamani More articles by this author , Vasileios MagoulianitisVasileios Magoulianitis More articles by this author , Yijing YangYijing Yang More articles by this author , Jintang XueJintang Xue More articles by this author , Jiaxin YangJiaxin Yang More articles by this author , Jinyuan LiuJinyuan Liu More articles by this author , Maria Sarah L. LenonMaria Sarah L. Lenon More articles by this author , Passant MohamedPassant Mohamed More articles by this author , Darryl H. HwangDarryl H. Hwang More articles by this author , Karan GillKaran Gill More articles by this author , Manju AronManju Aron More articles by this author , Vinay DuddalwarVinay Duddalwar More articles by this author , Suzanne L. PalmerSuzanne L. Palmer More articles by this author , C.-C. Jay KuoC.-C. Jay Kuo More articles by this author , Inderbir GillInderbir Gill More articles by this author , Andre Luis AbreuAndre Luis Abreu More articles by this author , and Chrysostomos L. NikiasChrysostomos L. Nikias More articles by this author View All Author Informationhttps://doi.org/10.1097/JU.0000000000003308.20AboutPDF ToolsAdd to favoritesDownload CitationsTrack CitationsPermissionsReprints ShareFacebookLinked InTwitterEmail Abstract INTRODUCTION AND OBJECTIVE: To develop a novel machine learning framework for automated detection of clinically significant prostate cancer (CSPCa) on Prostate Imaging Reporting & Data System (PIRADS) lesions confirmed by magnetic resonance imaging (MRI) informed target biopsy (TB). METHODS: From our prostate biopsy (PBx) database (IRB# HS-13-00663), consecutive men who underwent 3T multiparametric MRI (T2-weighted [T2W], diffusion-weighted images [DWI], apparent diffusion coefficient [ADC] and Dynamic Contrast Enhanced) followed by MRI-informed TB were identified. MRI was acquired and interpreted according to PIRADS v2 or v2.1 by experienced radiologists. The PIRADS 3-5 lesions were manually segmented on T2W, high-b-value (b≥1000) DWI, and ADC. Men with single lesion on MRI (PIRADS≥3) were included in the study. CSPCa was defined as Grade Group≥2 on TB. Independent modules were used for peripheral zones (PZ) and transition zones (TZ) regions of interest (ROI). For feature extraction and ROI classification, we adopt the Green Learning (GL) paradigm, and specifically the IPHOP-II feature extraction process (Figure 1). This offers advantages of lightweight model size and an explainable and transparent feature extraction. The performances of the GL model were assessed by the receiver operating characteristic (ROC) analysis. RESULTS: A total of 205 MRIs were used for training the GL model and it was validated on 54 MRIs. The PIRADS 3, 4 and 5 distribution was 152, 70 and 37 for PZ, and 84, 24, 15 for TZ, respectively. The CSPCa detection for PIRADS 3, 4 and 5 was 16%, 46% and 68% for PZ, and 13%, 29%, and 53%, for TZ, respectively. The area under the ROC curve (AUC) for the PZ on validation set was: 0.82 for overall; 0.71 for PIRADS 3; 0.80 for PIRADS 4; and 0.87 for PIRADS 5, respectively. The error rate was 27% for PIRADS 3; 18% for PIRADS 4; and 12% for PIRADS 5. The AUC for the TZ on validating set were: 0.79 for overall; 0.69 for PIRADS 3; 0.81 for PIRADS 4; and 0.86 for PIRADS 5, respectively. The error rate was 29% for PIRADS 3; 19% for PIRADS 4; and 13% for PIRADS 5. CONCLUSIONS: We developed a novel machine learning framework, the Green Learning, to detect CSPCa according to PIRADS stratification. This new tool assists with the proper prostatic lesions classification with improved accuracy to the current radiology performance. Source of Funding: None. © 2023 by American Urological Association Education and Research, Inc.FiguresReferencesRelatedDetails Volume 209Issue Supplement 4April 2023Page: e771 Advertisement Copyright & Permissions© 2023 by American Urological Association Education and Research, Inc.MetricsAuthor Information Masatomo Kaneko More articles by this author Giovanni E. Cacciamani More articles by this author Vasileios Magoulianitis More articles by this author Yijing Yang More articles by this author Jintang Xue More articles by this author Jiaxin Yang More articles by this author Jinyuan Liu More articles by this author Maria Sarah L. Lenon More articles by this author Passant Mohamed More articles by this author Darryl H. Hwang More articles by this author Karan Gill More articles by this author Manju Aron More articles by this author Vinay Duddalwar More articles by this author Suzanne L. Palmer More articles by this author C.-C. Jay Kuo More articles by this author Inderbir Gill More articles by this author Andre Luis Abreu More articles by this author Chrysostomos L. Nikias More articles by this author Expand All Advertisement PDF downloadLoading ...
更多查看译文
关键词
prostate cancer,novel machine learning framework,machine learning,mri-informed
AI 理解论文
溯源树
样例
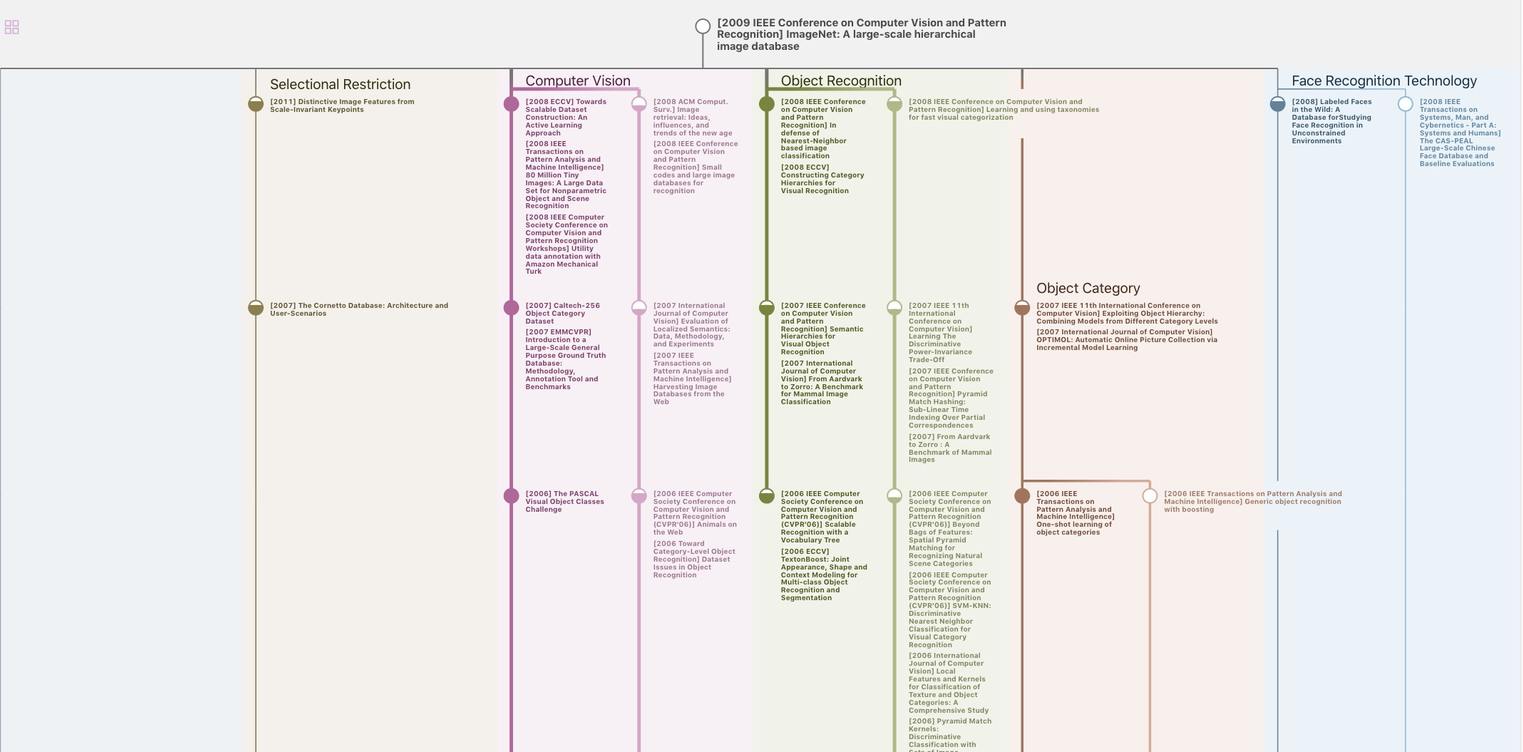
生成溯源树,研究论文发展脉络
Chat Paper
正在生成论文摘要