ML on Chain: The Case and Taxonomy of Machine Learning on Blockchain
2023 IEEE International Conference on Blockchain and Cryptocurrency (ICBC)(2023)
摘要
Blockchain has recently amassed a lot of interest from researchers and practitioners. This is due to its ability to manage data in a decentralized, transparent and accountable manner. However, blockchain applications are limited in terms of compute and cost effectiveness. These limits led to smart contracts—programs that are processed by the blockchain network—being relatively simple, not taking advantage of complex computation methods. In this paper, we shed light on the design space of using machine learning in blockchain applications (we call this ML on Chain for short). Although a lot of work discusses the intersection of blockchain and ML, there is little clarity in mapping, understanding, and evaluating the various approaches in this space. This paper contributes to this new area by the following: (1) we introduce a taxonomy of ML on Chain. This taxonomy considers existing and future potential methods in this area and groups them based on their design characteristics. We consider in the taxonomy five off-chain approaches and a baseline on-chain approach. (2) we perform an extensive experimental evaluation of ML on Chain methods. We compare the different 5 groups of solutions across different settings and three ML model types: logistic regression, k-nearest neighbors and neural networks. (3) Using the taxonomy and experimental study, we provide insights about the current state and challenges of the ML on Chain space; we use this newfound understanding to discuss potential future approaches and provide suggestions to address ML on Chain challenges.
更多查看译文
AI 理解论文
溯源树
样例
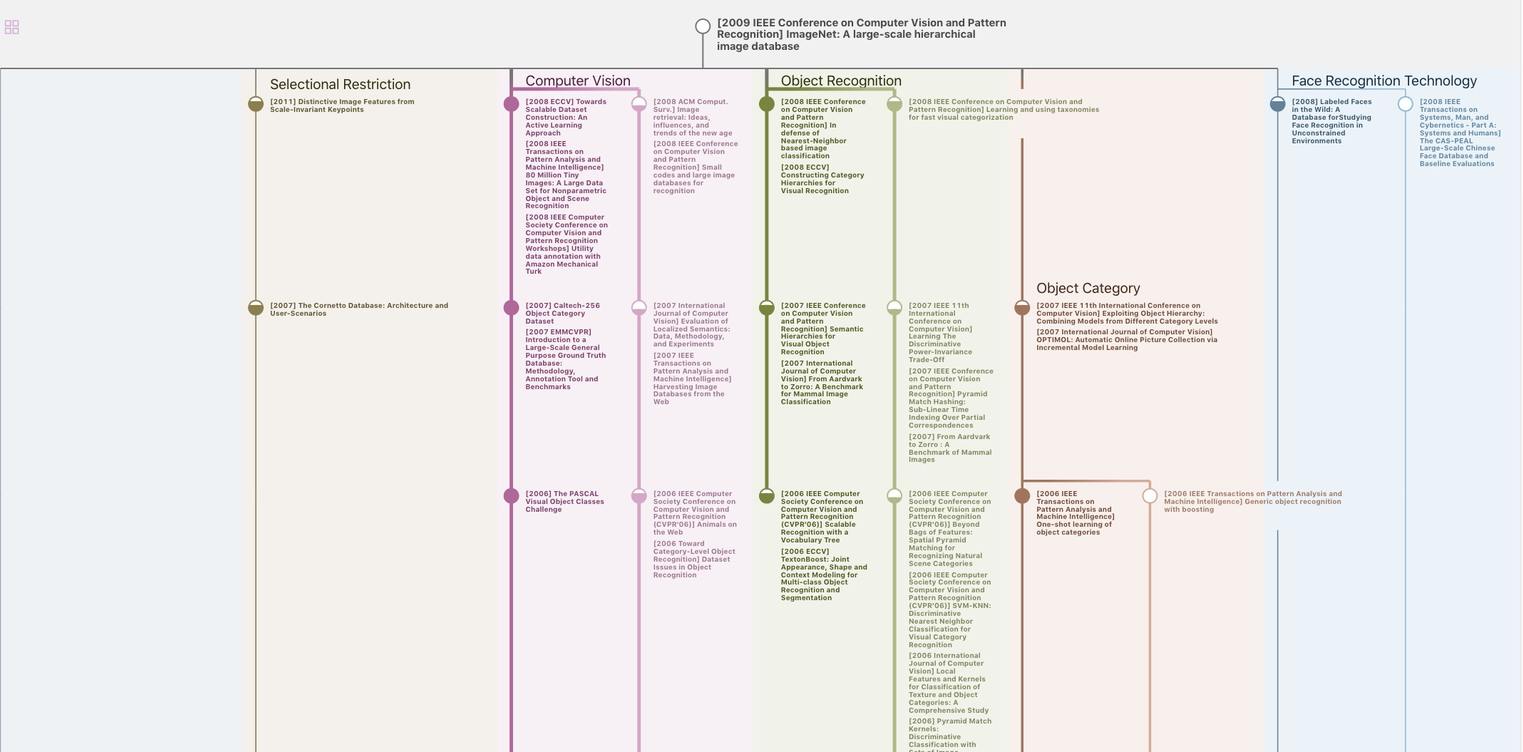
生成溯源树,研究论文发展脉络
Chat Paper
正在生成论文摘要