Comparison of the Strengths and Weaknesses of Machine Learning Algorithms and Feature Selection on KEGG Database Microbial Gene Pathway Annotation and Its Effects on Reconstructed Network Topology.
Journal of computational biology(2023)
摘要
The development of tools for the annotation of genes from newly sequenced species has not evolved much from homologous alignment to prior annotated species. While the quality of gene annotations continues to decline as we sequence and assemble more evolutionary distant gut microbiome species, machine learning presents a high quality alternative to traditional techniques. In this study, we investigate the relative performance of common classical and nonclassical machine learning algorithms in the problem of gene annotation using human microbiome-associated species genes from the KEGG database. The majority of the ensemble, clustering, and deep learning algorithms that we investigated showed higher prediction accuracy than CD-Hit in predicting partial KEGG function. Motif-based, machine-learning methods of annotation in new species were faster and had higher precision-recall than methods of homologous alignment or orthologous gene clustering. Gradient boosted ensemble methods and neural networks also predicted higher connectivity in reconstructed KEGG pathways, finding twice as many new pathway interactions than blast alignment. The use of motif-based, machine-learning algorithms in annotation software will allow researchers to develop powerful tools to interact with bacterial microbiomes in ways previously unachievable through homologous sequence alignment alone.
更多查看译文
关键词
biological databases,functional annotation,machine learning,network biology
AI 理解论文
溯源树
样例
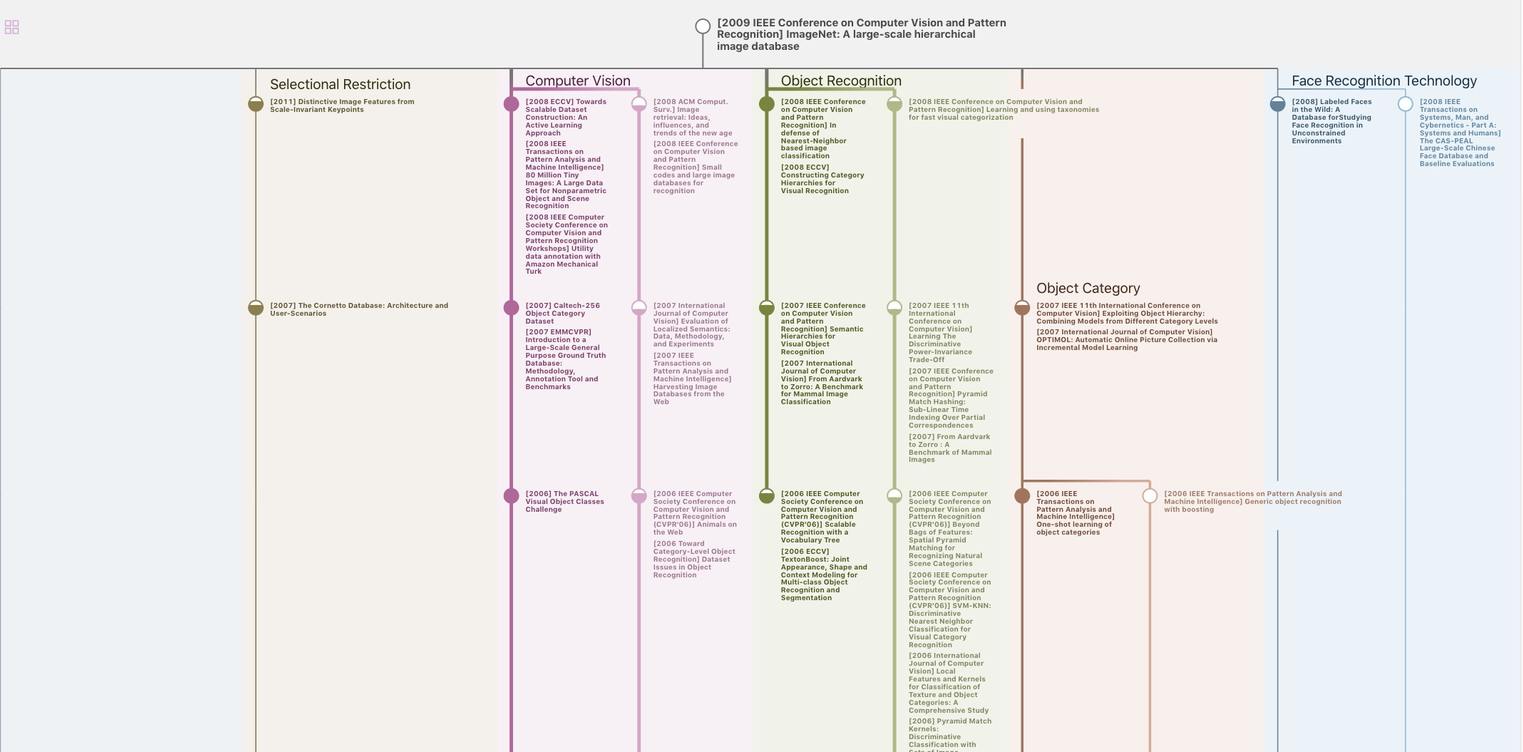
生成溯源树,研究论文发展脉络
Chat Paper
正在生成论文摘要