[Revealing Driving Factors of Urban O 3 Based on Explainable Machine Learning].
Huan jing ke xue= Huanjing kexue(2023)
摘要
Driven by precursor emissions, meteorological conditions, and other factors, atmospheric ozone (O) has become the main pollutant affecting urban air quality in summer. The current deductive models driven by physical and chemical mechanisms require a large number of parameters for the analysis of O pollution, and the calculation timeliness is poor. The data-driven inductive models are efficient but have problems such as poor explanation. In this study, an explainable model of data-driven Correlation-ML-SHAP was established to reveal the strongly correlated influencing factors of O concentration. Additionally, the machine learning ML module coupled with the explainable SHAP module was used to calculate the contributions of driving factors to O concentration, so as to realize the quantitative analysis of driving factors. The O pollution process in the summer of 2021 in Jincheng City was used as an example to carry out the application research. The results showed that the Correlation-ML-SHAP model could reveal and use strong driving factors to simulate O concentration and quantify influence contribution, and the ML module used the XGBoost model to achieve the best simulation accuracy. Air temperature, solar radiation, relative humidity, and precursor emission level were the strong driving factors of O pollution in Jincheng City in summer 2021, and the contribution weights were 32.1%, 21.3%, 16.5%, and 15.6%. The contribution weights of air temperature, solar radiation, and precursor emission level increased by 3.4%, 1.2%, and 1.2% on polluted days, respectively, and the contribution weights of precursor emission level rose to third place on polluted days. Each driving factor had a nonlinear interaction effect on O concentration. When the air temperature exceeded 24℃, or the relative humidity was lower than 70%, there was a 94.9% and 94.1% probability of positive contribution to O pollution, respectively. Under such meteorological conditions, (NO) exceeded 9 μg·m, or (CO) exceeded 0.7 mg·m, and there was a 94.9% and 99.3% probability of positive contribution to O pollution, respectively. The southeast wind speed was lower than 5.8 m·s, or the south wind speed was lower than 5.3 m·s, both of which contributed positively to O pollution. The model quantitatively analyzed the influence contribution of various driving factors on urban O concentration, which could provide a basis for the prevention and control of urban atmospheric O pollution in summer.
更多查看译文
关键词
urban o3,explainable machine learning,machine learning
AI 理解论文
溯源树
样例
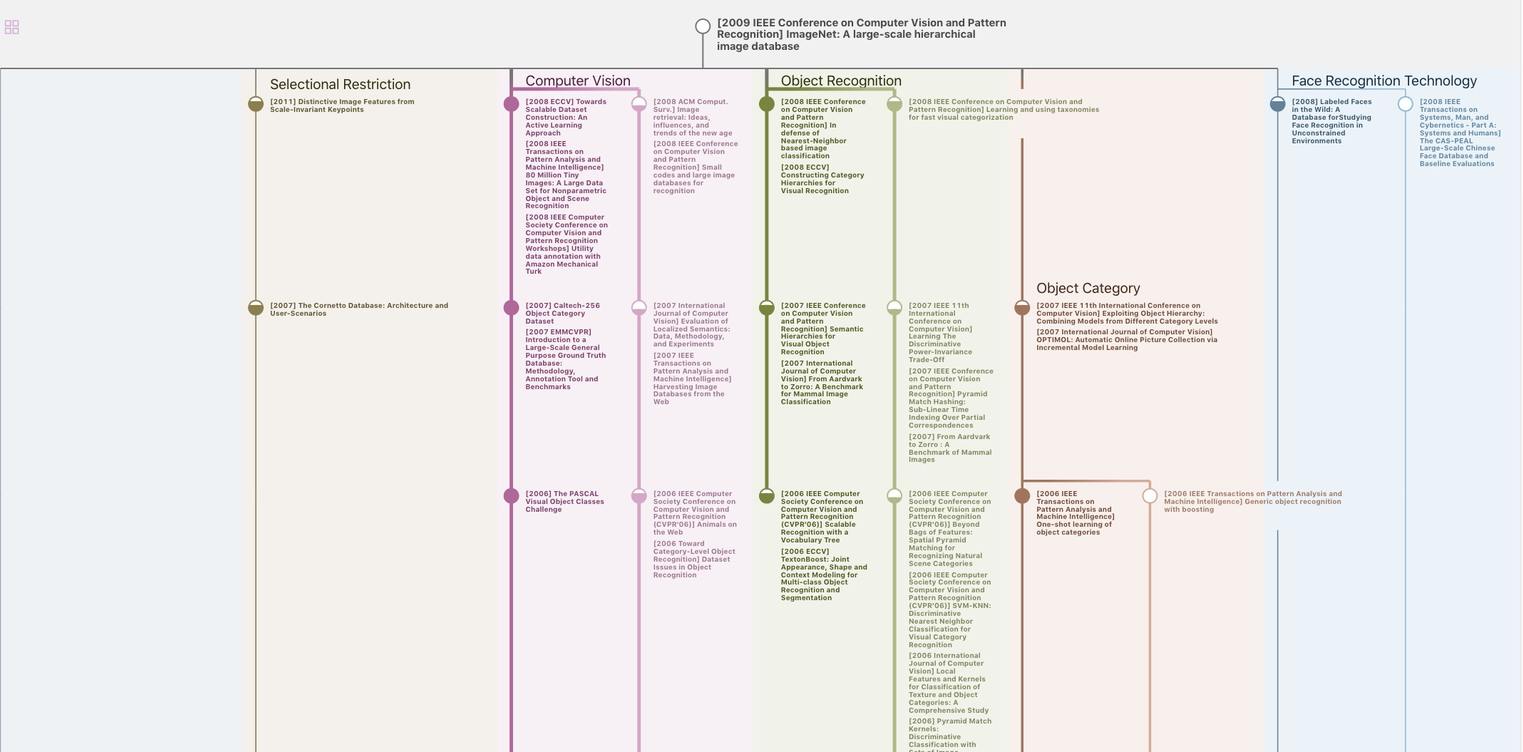
生成溯源树,研究论文发展脉络
Chat Paper
正在生成论文摘要