Topic-aware Intention Network for Explainable Recommendation with Knowledge Enhancement
ACM transactions on office information systems(2023)
摘要
Recently, recommender systems based on knowledge graphs (KGs) have become a popular research direction. Graph neural network (GNN) is the key technology of KG-based recommendation systems. However, existing GNNs have a significant flaw: They cannot explicitly model users’ intent in recommendations. Intent plays an essential role in users’ behaviors. For example, users may first generate an intent to purchase a certain group of items and then select a specific item from the group based on their preferences. Therefore, explicitly modeling intent has a positive significance for improving recommendation performance and providing explanations for recommendations. In this article, we propose a new model called Topic-aware Intention Network (TIN) for explainable recommendations with KGs. TIN models user representations from both preference and intent views. Specifically, we design a relational attention graph neural network to selectively aggregate information in KG to learn user preferences, and we propose a knowledge-enhanced topic model to learn user intent, which is viewed as topics hidden in user behavior sequences. Finally, we obtain the user representation by fusing user preference and intent through an attention network. The experimental results show that our proposed model outperforms the state-of-the-art methods and can generate reasonable explanations for the recommendation results.
更多查看译文
关键词
Knowledge graph,recommender system,topic model
AI 理解论文
溯源树
样例
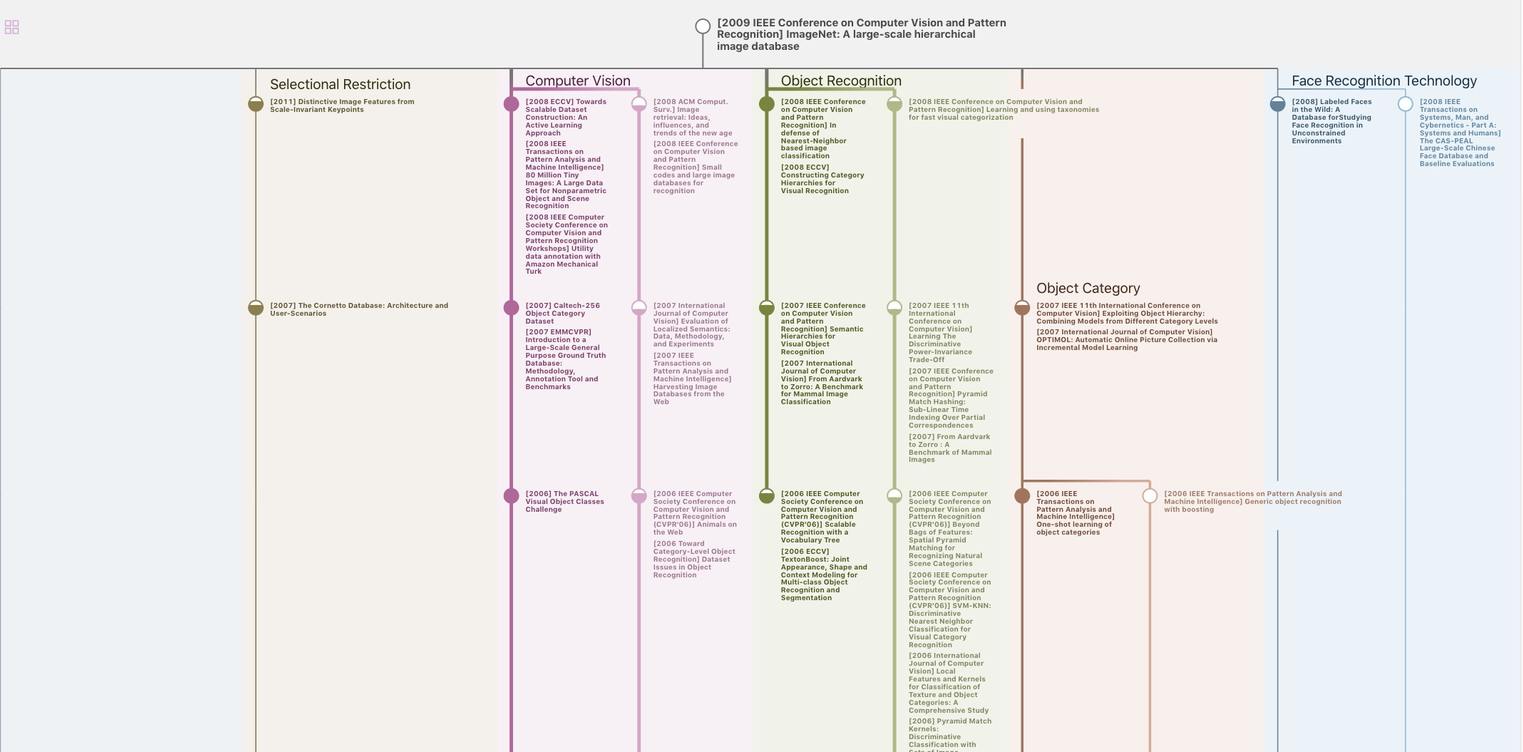
生成溯源树,研究论文发展脉络
Chat Paper
正在生成论文摘要