Semantic Consistent Embedding for Domain Adaptive Zero-Shot Learning.
IEEE transactions on image processing : a publication of the IEEE Signal Processing Society(2023)
摘要
Unsupervised domain adaptation has limitations when encountering label discrepancy between the source and target domains. While open-set domain adaptation approaches can address situations when the target domain has additional categories, these methods can only detect them but not further classify them. In this paper, we focus on a more challenging setting dubbed Domain Adaptive Zero-Shot Learning (DAZSL), which uses semantic embeddings of class tags as the bridge between seen and unseen classes to learn the classifier for recognizing all categories in the target domain when only the supervision of seen categories in the source domain is available. The main challenge of DAZSL is to perform knowledge transfer across categories and domain styles simultaneously. To this end, we propose a novel end-to-end learning mechanism dubbed Three-way Semantic Consistent Embedding (TSCE) to embed the source domain, target domain, and semantic space into a shared space. Specifically, TSCE learns domain-irrelevant categorical prototypes from the semantic embedding of class tags and uses them as the pivots of the shared space. The source domain features are aligned with the prototypes via their supervised information. On the other hand, the mutual information maximization mechanism is introduced to push the target domain features and prototypes towards each other. By this way, our approach can align domain differences between source and target images, as well as promote knowledge transfer towards unseen classes. Moreover, as there is no supervision in the target domain, the shared space may suffer from the catastrophic forgetting problem. Hence, we further propose a ranking-based embedding alignment mechanism to maintain the consistency between the semantic space and the shared space. Experimental results on both I2AwA and I2WebV clearly validate the effectiveness of our method.
更多查看译文
关键词
Zero-shot learning, unsupervised domain adaptation, transfer learning
AI 理解论文
溯源树
样例
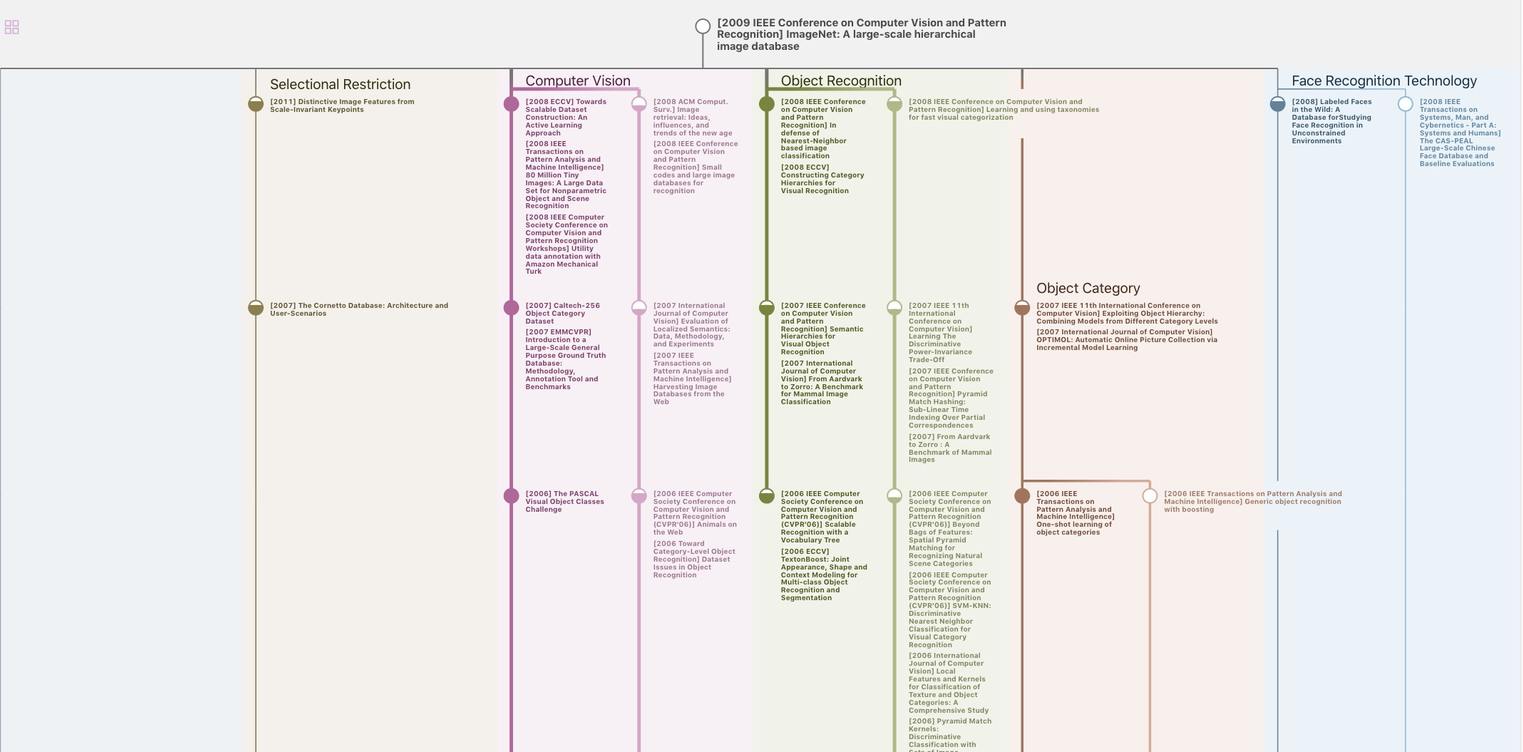
生成溯源树,研究论文发展脉络
Chat Paper
正在生成论文摘要