iEnhancer-DCSA: identifying enhancers via dual-scale convolution and spatial attention
BMC genomics(2023)
摘要
Background Due to the dynamic nature of enhancers, identifying enhancers and their strength are major bioinformatics challenges. With the development of deep learning, several models have facilitated enhancers detection in recent years. However, existing studies either neglect different length motifs information or treat the features at all spatial locations equally. How to effectively use multi-scale motifs information while ignoring irrelevant information is a question worthy of serious consideration. In this paper, we propose an accurate and stable predictor iEnhancer-DCSA, mainly composed of dual-scale fusion and spatial attention, automatically extracting features of different length motifs and selectively focusing on the important features. Results Our experimental results demonstrate that iEnhancer-DCSA is remarkably superior to existing state-of-the-art methods on the test dataset. Especially, the accuracy and MCC of enhancer identification are improved by 3.45% and 9.41%, respectively. Meanwhile, the accuracy and MCC of enhancer classification are improved by 7.65% and 18.1%, respectively. Furthermore, we conduct ablation studies to demonstrate the effectiveness of dual-scale fusion and spatial attention. Conclusions iEnhancer-DCSA will be a valuable computational tool in identifying and classifying enhancers, especially for those not included in the training dataset.
更多查看译文
关键词
Enhancers,Dual-scale convolution,Spatial attention,Word embedding
AI 理解论文
溯源树
样例
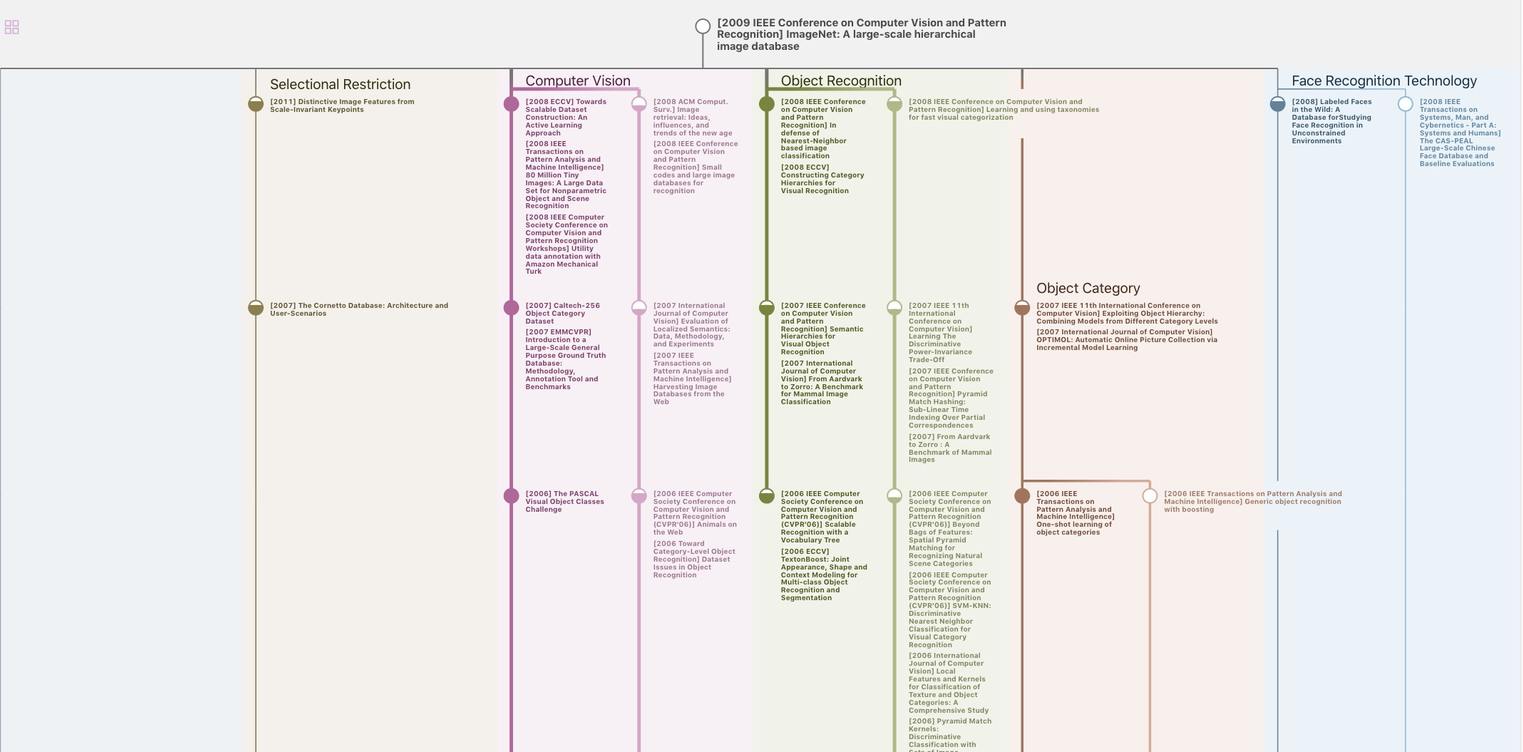
生成溯源树,研究论文发展脉络
Chat Paper
正在生成论文摘要