Network Security Situation Prediction Based on Optimized Clock-Cycle Recurrent Neural Network for Sensor-Enabled Networks.
Sensors (Basel, Switzerland)(2023)
摘要
We propose an optimized Clockwork Recurrent Neural Network (CW-RNN) based approach to address temporal dynamics and nonlinearity in network security situations, improving prediction accuracy and real-time performance. By leveraging the clock-cycle RNN, we enable the model to capture both short-term and long-term temporal features of network security situations. Additionally, we utilize the Grey Wolf Optimization (GWO) algorithm to optimize the hyperparameters of the network, thus constructing an enhanced network security situation prediction model. The introduction of a clock-cycle for hidden units allows the model to learn short-term information from high-frequency update modules while retaining long-term memory from low-frequency update modules, thereby enhancing the model's ability to capture data patterns. Experimental results demonstrate that the optimized clock-cycle RNN outperforms other network models in extracting the temporal and nonlinear features of network security situations, leading to improved prediction accuracy. Furthermore, our approach has low time complexity and excellent real-time performance, ideal for monitoring large-scale network traffic in sensor networks.
更多查看译文
关键词
network security, situation prediction, Grey Wolf Optimization (GWO), Clockwork Recurrent Neural Networks (CW-RNN)
AI 理解论文
溯源树
样例
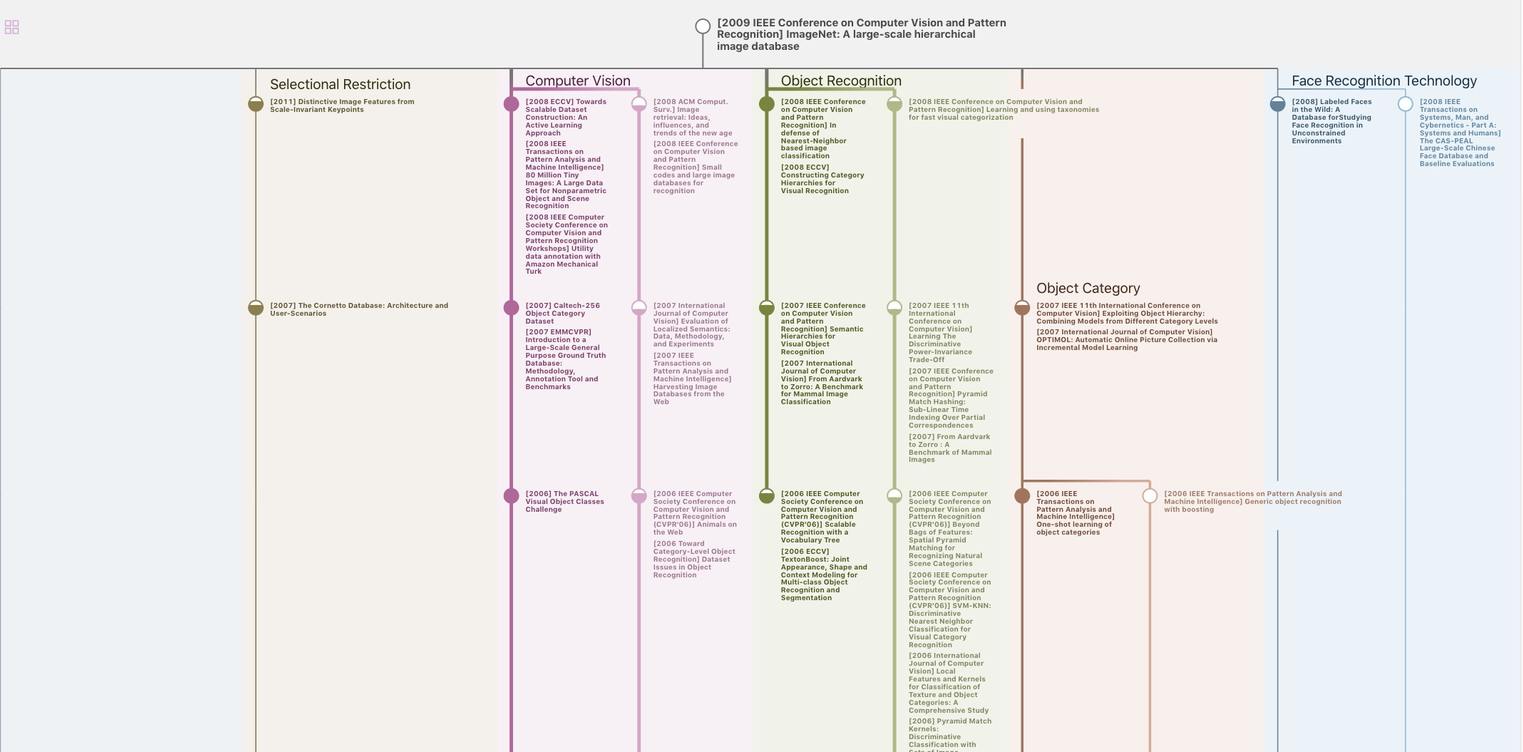
生成溯源树,研究论文发展脉络
Chat Paper
正在生成论文摘要