Generating Efficient Training Data via LLM-based Attribute Manipulation
CoRR(2023)
摘要
In this paper, we propose a novel method, Chain-of-Thoughts Attribute Manipulation (CoTAM), to guide few-shot learning by carefully crafted data from Large Language Models (LLMs). The main idea is to create data with changes only in the attribute targeted by the task. Inspired by facial attribute manipulation, our approach generates label-switched data by leveraging LLMs to manipulate task-specific attributes and reconstruct new sentences in a controlled manner. Instead of conventional latent representation controlling, we implement chain-of-thoughts decomposition and reconstruction to adapt the procedure to LLMs. Extensive results on text classification and other tasks verify the advantage of CoTAM over other LLM-based text generation methods with the same number of training examples. Analysis visualizes the attribute manipulation effectiveness of CoTAM and presents the potential of LLM-guided learning with even less supervision.
更多查看译文
关键词
attribute manipulation,data,training,efficient,llm-based
AI 理解论文
溯源树
样例
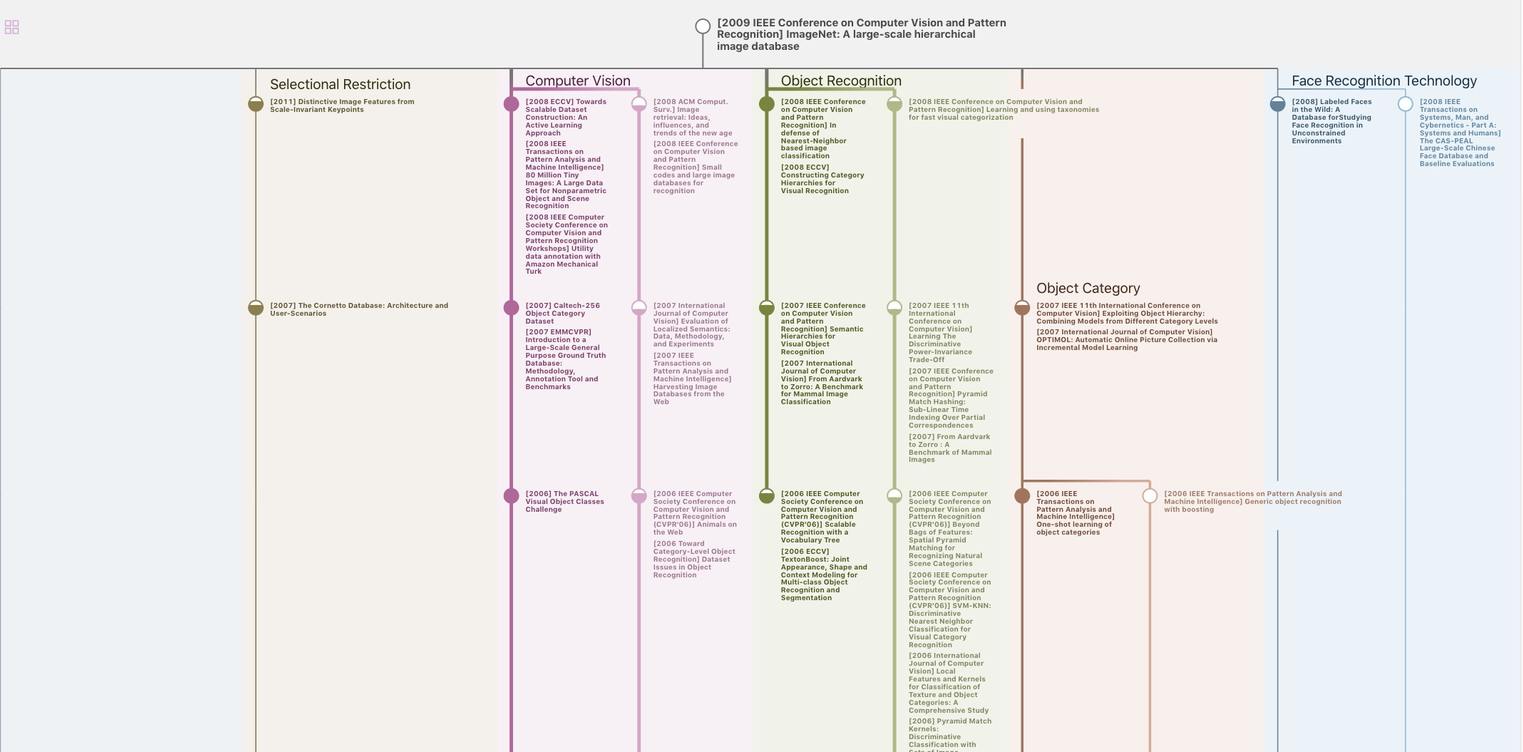
生成溯源树,研究论文发展脉络
Chat Paper
正在生成论文摘要