A Dynamic Points Removal Benchmark in Point Cloud Maps
CoRR(2023)
摘要
In the field of robotics, the point cloud has become an essential map representation. From the perspective of downstream tasks like localization and global path planning, points corresponding to dynamic objects will adversely affect their performance. Existing methods for removing dynamic points in point clouds often lack clarity in comparative evaluations and comprehensive analysis. Therefore, we propose an easy-to-extend unified benchmarking framework for evaluating techniques for removing dynamic points in maps. It includes refactored state-of-art methods and novel metrics to analyze the limitations of these approaches. This enables researchers to dive deep into the underlying reasons behind these limitations. The benchmark makes use of several datasets with different sensor types. All the code and datasets related to our study are publicly available for further development and utilization.
更多查看译文
关键词
Point Cloud,Dynamic Point,Point Cloud Map,Path Planning,Types Of Sensors,Map Points,Dynamic Objects,Refactoring,Pedestrian,Grid Cells,Learning-based Methods,Global Map,Static Function,Point Cloud Data,Representation Of The Environment,Benchmark Results,Simultaneous Localization And Mapping,Raw Point,Ground Points,Robot Operating System,Ray Casting,Query Point,Noise Points,True Point,Occupancy Values,Occupancy Grid,Raw Point Cloud,Point Cloud Processing,Occupancy Map
AI 理解论文
溯源树
样例
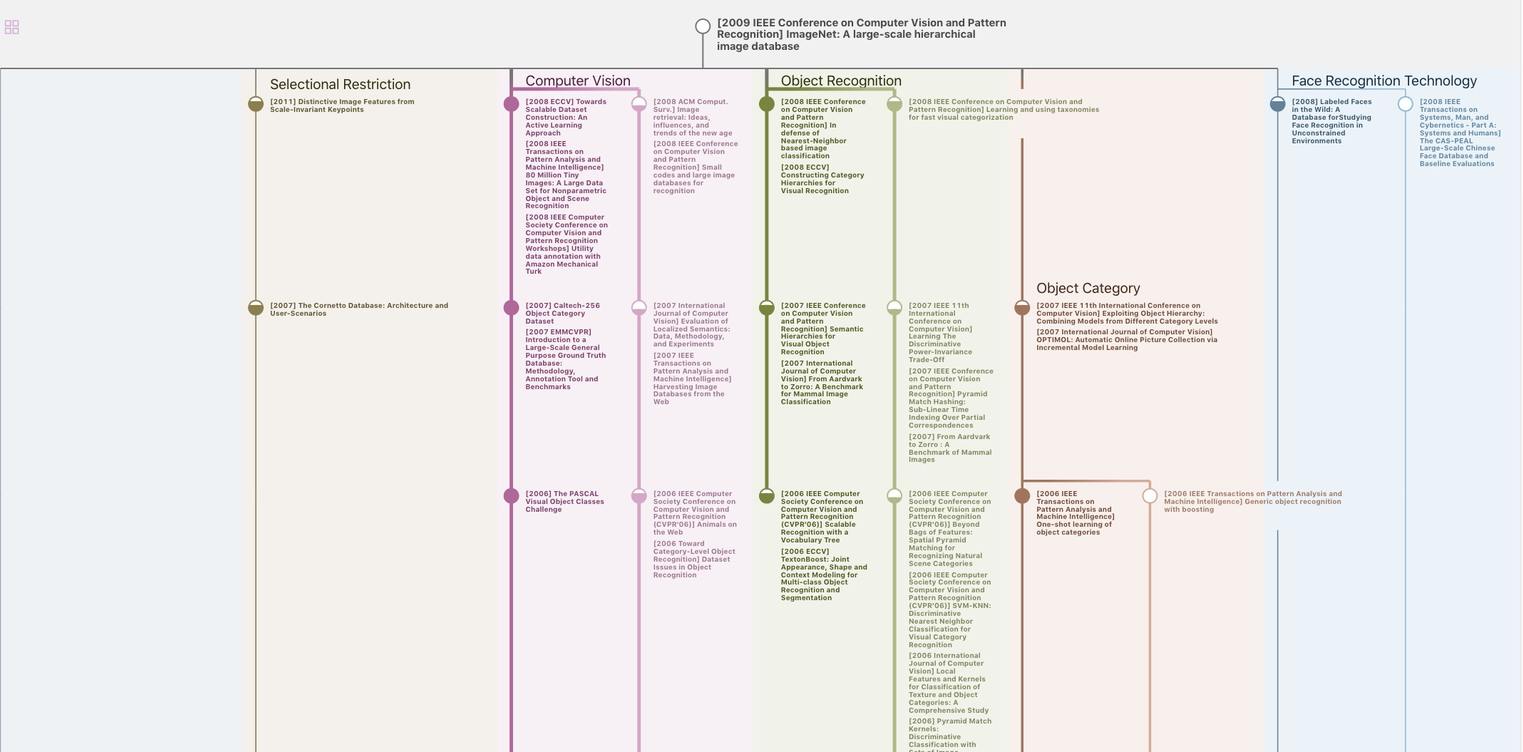
生成溯源树,研究论文发展脉络
Chat Paper
正在生成论文摘要