Replay to Remember: Continual Layer-Specific Fine-Tuning for German Speech Recognition
ARTIFICIAL NEURAL NETWORKS AND MACHINE LEARNING, ICANN 2023, PT VII(2023)
摘要
While Automatic Speech Recognition (ASR) models have shown significant advances with the introduction of unsupervised or self-supervised training techniques, these improvements are still only limited to a subsection of languages and speakers. Transfer learning enables the adaptation of large-scale multilingual models to not only low-resource languages but also to more specific speaker groups. However, fine-tuning on data from new domains is usually accompanied by a decrease in performance on the original domain. Therefore, in our experiments, we examine how well the performance of large-scale ASR models can be approximated for smaller domains, with our own dataset of German Senior Voice Commands (SVC-de), and how much of the general speech recognition performance can be preserved by selectively freezing parts of the model during training. To further increase the robustness of the ASR model to vocabulary and speakers outside of the fine-tuned domain, we apply Experience Replay [20] for continual learning. By adding only a fraction of data from the original domain, we are able to reach Word-Error-Rates (WERs) below 5% on the new domain, while stabilizing performance for general speech recognition at acceptable WERs.
更多查看译文
关键词
Automatic Speech Recognition,Transfer Learning,Continual Learning,Domain Adaptation
AI 理解论文
溯源树
样例
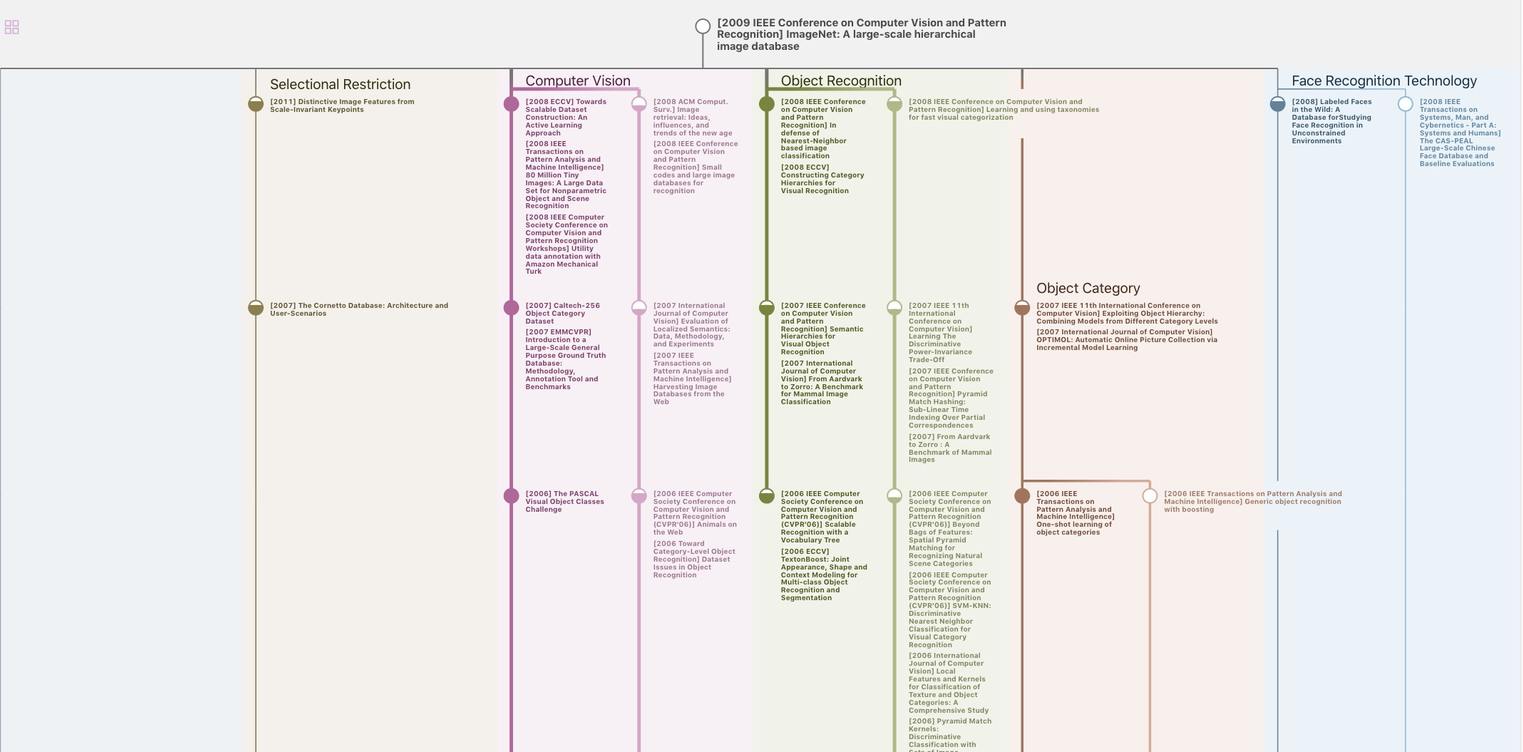
生成溯源树,研究论文发展脉络
Chat Paper
正在生成论文摘要