Deep learning for histopathological segmentation of smooth muscle in the urinary bladder
BMC medical informatics and decision making(2023)
摘要
Background Histological assessment of smooth muscle is a critical step particularly in staging malignant tumors in various internal organs including the urinary bladder. Nonetheless, manual segmentation and classification of muscular tissues by pathologists is often challenging. Therefore, a fully automated and reliable smooth muscle image segmentation system is in high demand. Methods To characterize muscle fibers in the urinary bladder, including muscularis mucosa (MM) and muscularis propria (MP), we assessed 277 histological images from surgical specimens, using two well-known deep learning (DL) model groups, one including VGG16, ResNet18, SqueezeNet, and MobileNetV2, considered as a patch-based approach, and the other including U-Net, MA-Net, DeepLabv3 + , and FPN, considered as a pixel-based approach. All the trained models in both the groups were evaluated at pixel-level for their performance. Results For segmenting MP and non-MP (including MM) regions, MobileNetV2, in the patch-based approach and U-Net, in the pixel-based approach outperformed their peers in the groups with mean Jaccard Index equal to 0.74 and 0.79, and mean Dice co-efficient equal to 0.82 and 0.88, respectively. We also demonstrated the strengths and weaknesses of the models in terms of speed and prediction accuracy. Conclusions This work not only creates a benchmark for future development of tools for the histological segmentation of smooth muscle but also provides an effective DL-based diagnostic system for accurate pathological staging of bladder cancer.
更多查看译文
关键词
Deep learning,Smooth muscle,Bladder cancer,Pathological slides,Segmentation
AI 理解论文
溯源树
样例
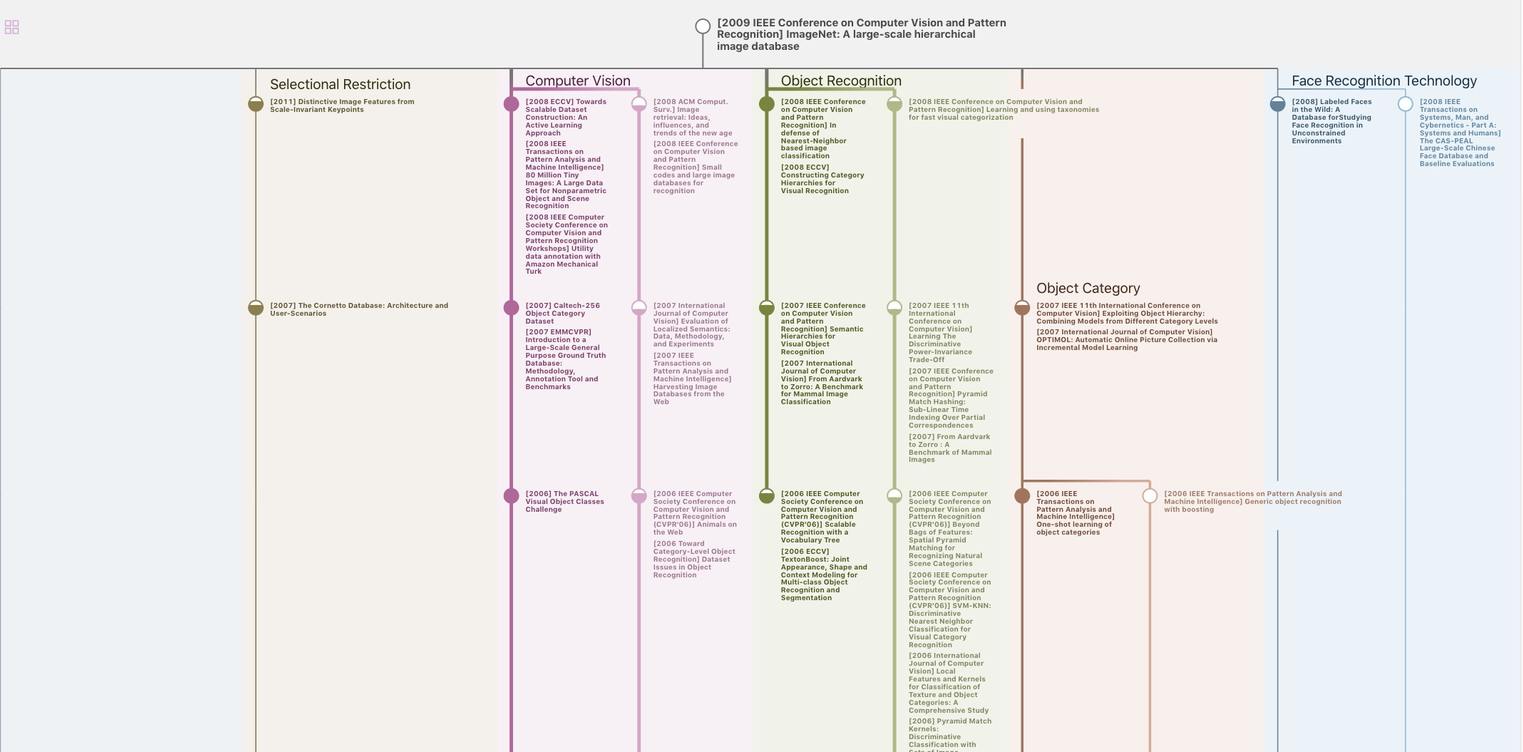
生成溯源树,研究论文发展脉络
Chat Paper
正在生成论文摘要