Rotor dynamics informed deep learning for detection, identification, and localization of shaft crack and unbalance defects
ADVANCED ENGINEERING INFORMATICS(2023)
摘要
This paper proposes a new model, called rotor finite element mimetic neural network (RFEMNN), for diagnosing rotor unbalance and shaft crack faults. RFEMNN uses a convolution long short-term memory networks (CNN-LSTM) architecture to achieve fault recognition and localization in the rotor structure. The term "Mimetic"refers to the topology of the rotor finite element model and solution process by custom designing NN structure and data flow, which is based on the physics informed structure paradigm. The proposed "Mimetic"theory enhances the physics consistency of the RFEMNN model without requiring complete analytic physical knowledge or all parameter values. The proposed model's effectiveness is validated through experimental tests, compared to 9 benchmark models from the literature and one deep learning model with the same depth but no physics embedding. Results showed that RFEMNN outperformed the benchmark models with a comprehensive diagnostics accuracy of 94.7%, indicating its potential for practical industrial applications.
更多查看译文
关键词
shaft crack,deep learning
AI 理解论文
溯源树
样例
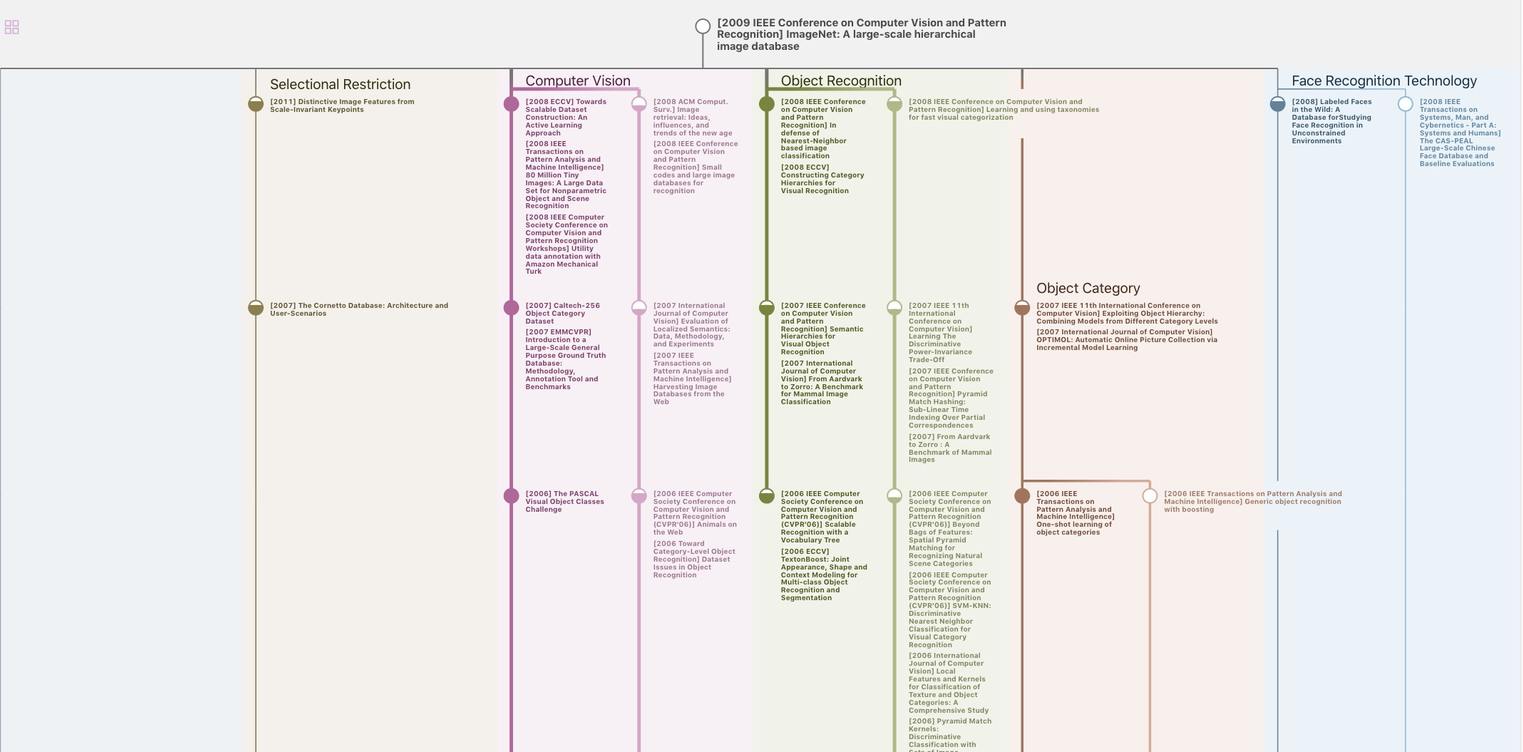
生成溯源树,研究论文发展脉络
Chat Paper
正在生成论文摘要