Dissenting Explanations: Leveraging Disagreement to Reduce Model Overreliance
AAAI 2024(2024)
摘要
While modern explanation methods have been shown to be inconsistent and contradictory, the explainability of black-box models nevertheless remains desirable. When the role of explanations extends from understanding models to aiding decision making, the semantics of explanations is not always fully understood – to what extent do explanations ``explain” a decision and to what extent do they merely advocate for a decision? Can we help humans gain insights from explanations accompanying correct predictions and not over-rely on incorrect predictions advocated for by explanations? With this perspective in mind, we introduce the notion of dissenting explanations: conflicting predictions with accompanying explanations. We first explore the advantage of dissenting explanations in the setting of model multiplicity, where multiple models with similar performance may have different predictions. Through a human study on the task of identifying deceptive reviews, we demonstrate that dissenting explanations reduce overreliance on model predictions, without reducing overall accuracy. Motivated by the utility of dissenting explanations we present both global and local methods for their generation.
更多查看译文
关键词
General
AI 理解论文
溯源树
样例
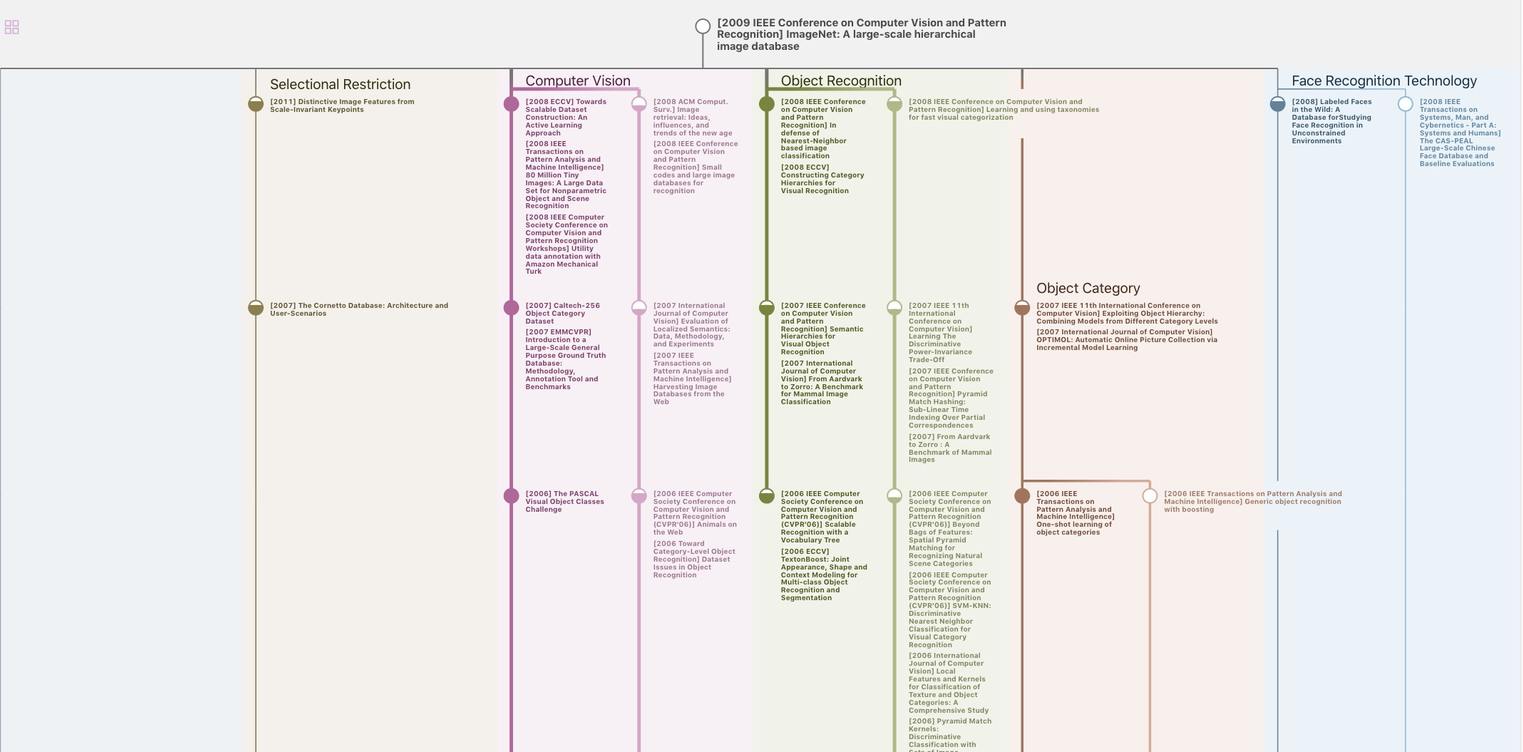
生成溯源树,研究论文发展脉络
Chat Paper
正在生成论文摘要