Corrected kernel principal component analysis for model structural change detection
arXiv (Cornell University)(2023)
摘要
This paper develops a method to detect model structural changes by applying a Corrected Kernel Principal Component Analysis (CKPCA) to construct the so-called central distribution deviation subspaces. This approach can efficiently identify the mean and distribution changes in these dimension reduction subspaces. We derive that the locations and number changes in the dimension reduction data subspaces are identical to those in the original data spaces. Meanwhile, we also explain the necessity of using CKPCA as the classical KPCA fails to identify the central distribution deviation subspaces in these problems. Additionally, we extend this approach to clustering by embedding the original data with nonlinear lower dimensional spaces, providing enhanced capabilities for clustering analysis. The numerical studies on synthetic and real data sets suggest that the dimension reduction versions of existing methods for change point detection and clustering significantly improve the performances of existing approaches in finite sample scenarios.
更多查看译文
关键词
change detection,structural
AI 理解论文
溯源树
样例
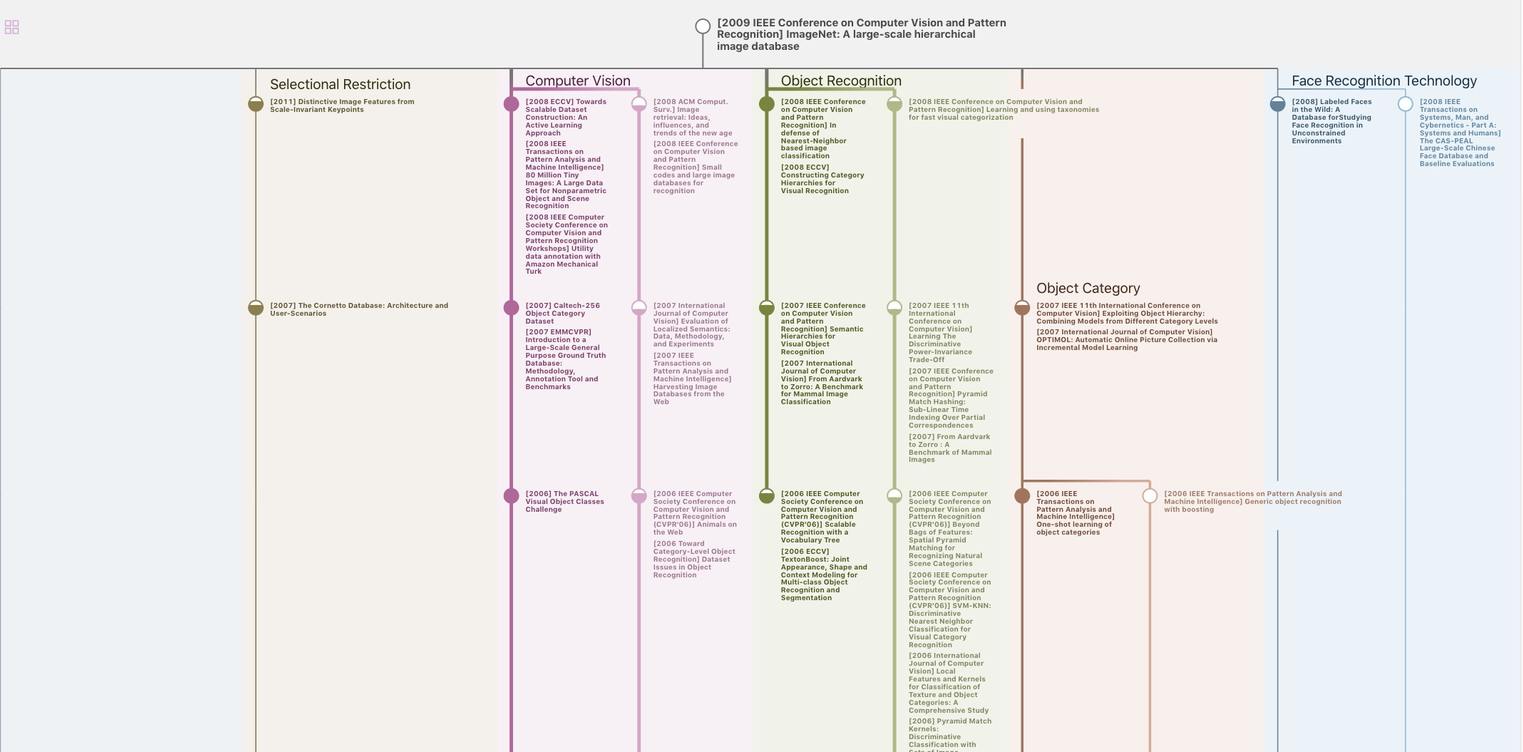
生成溯源树,研究论文发展脉络
Chat Paper
正在生成论文摘要