Automated Polynomial Filter Learning for Graph Neural Networks
CoRR(2023)
摘要
Polynomial graph filters have been widely used as guiding principles in the design of Graph Neural Networks (GNNs). Recently, the adaptive learning of the polynomial graph filters has demonstrated promising performance for modeling graph signals on both homophilic and heterophilic graphs, owning to their flexibility and expressiveness. In this work, we conduct a novel preliminary study to explore the potential and limitations of polynomial graph filter learning approaches, revealing a severe overfitting issue. To improve the effectiveness of polynomial graph filters, we propose Auto-Polynomial, a novel and general automated polynomial graph filter learning framework that efficiently learns better filters capable of adapting to various complex graph signals. Comprehensive experiments and ablation studies demonstrate significant and consistent performance improvements on both homophilic and heterophilic graphs across multiple learning settings considering various labeling ratios, which unleashes the potential of polynomial filter learning.
更多查看译文
关键词
neural networks,learning
AI 理解论文
溯源树
样例
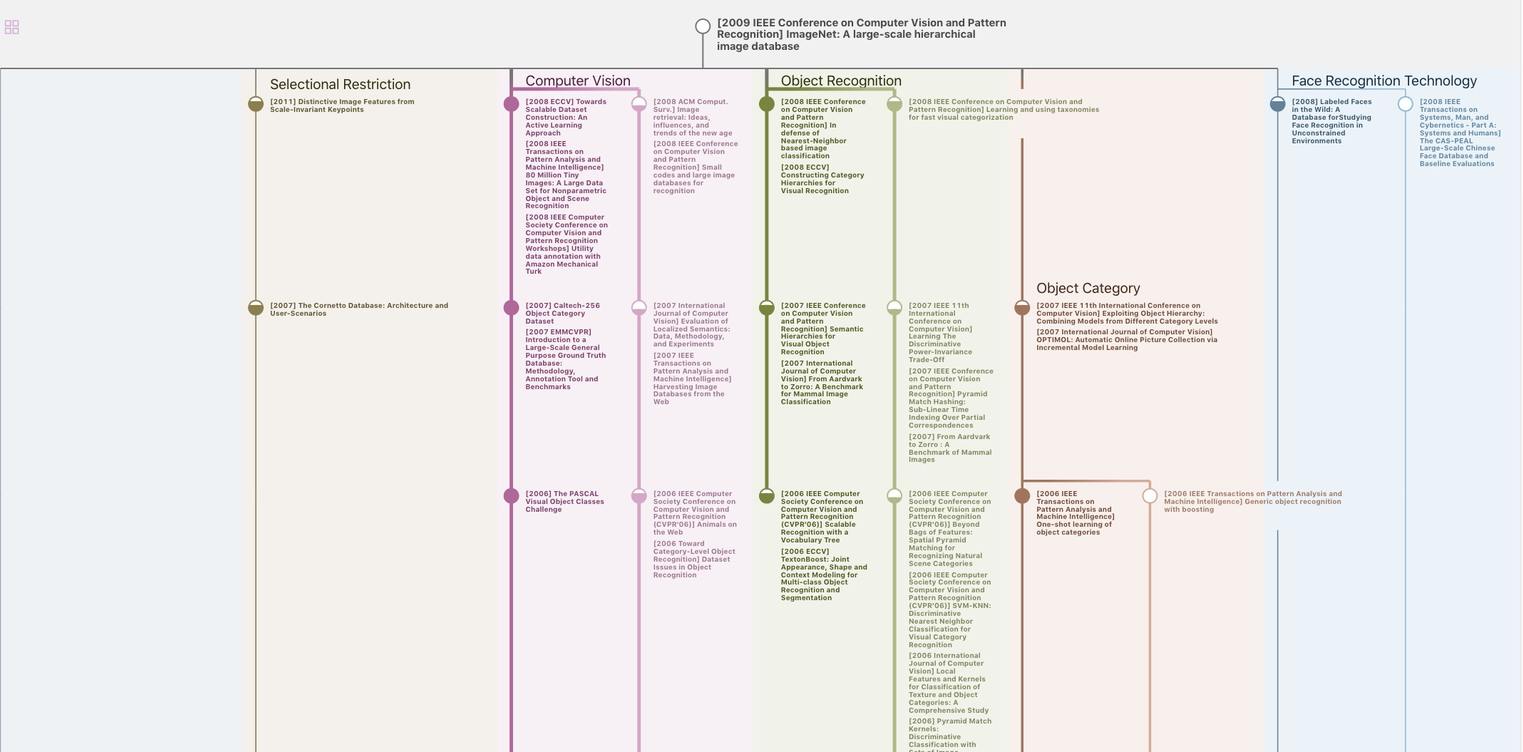
生成溯源树,研究论文发展脉络
Chat Paper
正在生成论文摘要