Unbiased Image Synthesis via Manifold Guidance in Diffusion Models
arxiv(2023)
摘要
Diffusion Models are a potent class of generative models capable of producing
high-quality images. However, they often inadvertently favor certain data
attributes, undermining the diversity of generated images. This issue is
starkly apparent in skewed datasets like CelebA, where the initial dataset
disproportionately favors females over males by 57.9
generated data where female representation outstrips males by 148
response, we propose a plug-and-play method named Manifold Guidance Sampling,
which is also the first unsupervised method to mitigate bias issue in DDPMs.
Leveraging the inherent structure of the data manifold, this method steers the
sampling process towards a more uniform distribution, effectively dispersing
the clustering of biased data. Without the need for modifying the existing
model or additional training, it significantly mitigates data bias and enhances
the quality and unbiasedness of the generated images.
更多查看译文
AI 理解论文
溯源树
样例
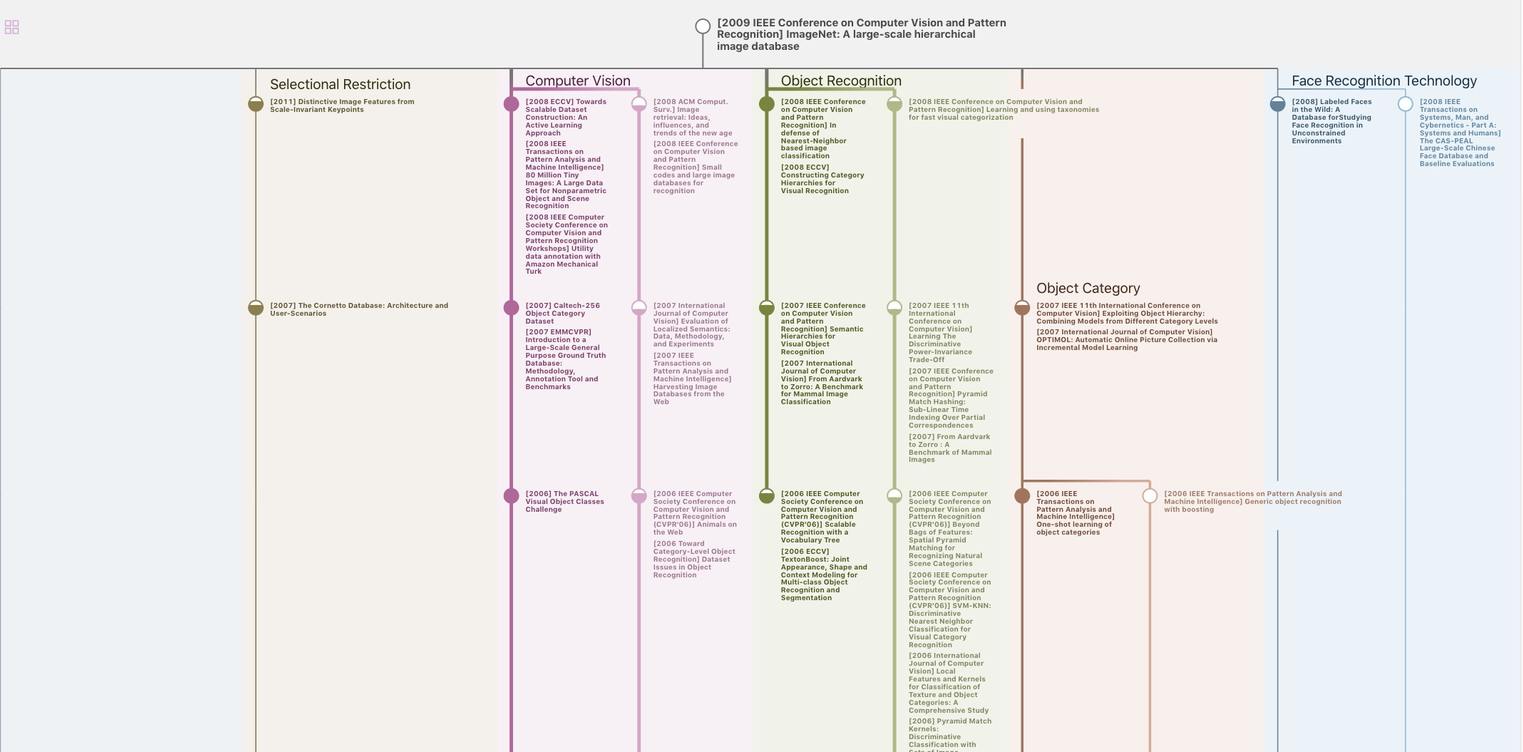
生成溯源树,研究论文发展脉络
Chat Paper
正在生成论文摘要