ROFusion: Efficient Object Detection Using Hybrid Point-Wise Radar-Optical Fusion
ARTIFICIAL NEURAL NETWORKS AND MACHINE LEARNING, ICANN 2023, PT VII(2023)
摘要
Radars, due to their robustness to adverse weather conditions and ability to measure object motions, have served in autonomous driving and intelligent agents for years. However, Radar-based perception suffers from its unintuitive sensing data, which lack of semantic and structural information of scenes. To tackle this problem, camera and Radar sensor fusion has been investigated as a trending strategy with low cost, high reliability and strong maintenance. While most recent works explore how to explore Radar point clouds and images, rich contextual information within Radar observation are discarded. In this paper, we propose a hybrid point-wise Radar-Optical fusion approach for object detection in autonomous driving scenarios. The framework benefits from dense contextual information from both the range-doppler spectrum and images which are integrated to learn a multi-modal feature representation. Furthermore, we propose a novel local coordinate formulation, tackling the object detection task in an object-centric coordinate. Extensive results show that with the information gained from optical images, we could achieve leading performance in object detection (97.69% recall) compared to recent state-of-the-art methods FFT-RadNet [17] (82.86% recall). Ablation studies verify the key design choices and practicability of our approach given machine generated imperfect detections. The code will be available at https://github.com/LiuLiu-55/ROFusion.
更多查看译文
关键词
Radar-Optical Fusion,Object Detection,Deep Learning
AI 理解论文
溯源树
样例
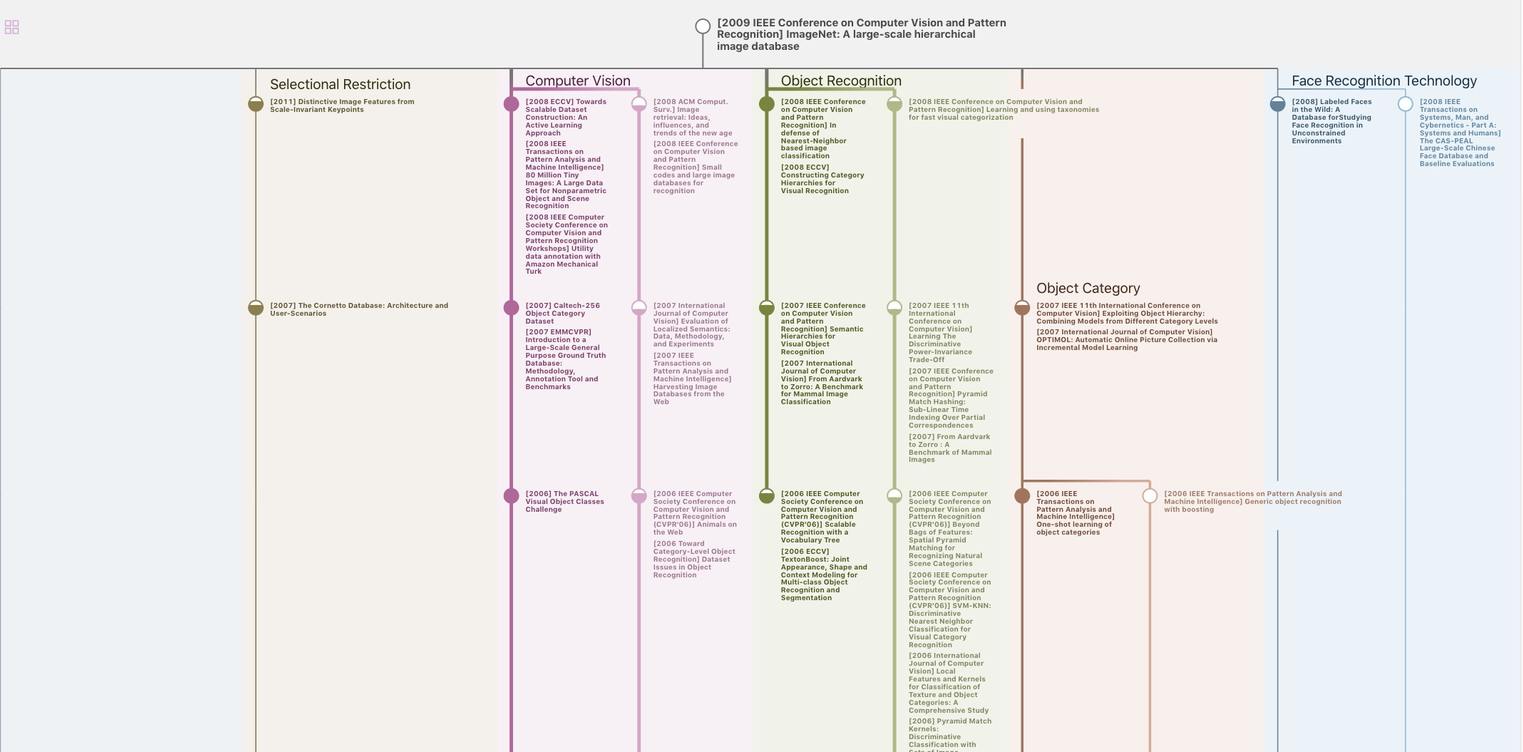
生成溯源树,研究论文发展脉络
Chat Paper
正在生成论文摘要