Semi-supervised multi-channel speaker diarization with cross-channel attention
2023 IEEE Automatic Speech Recognition and Understanding Workshop (ASRU)(2023)
摘要
Most neural speaker diarization systems rely on sufficient manual training data labels, which are hard to collect under real-world scenarios. This paper proposes a semi-supervised speaker diarization system to utilize large-scale multi-channel training data by generating pseudo-labels for unlabeled data. Furthermore, we introduce cross-channel attention into the Neural Speaker Diarization Using Memory-Aware Multi-Speaker Embedding (NSD-MA-MSE) to learn channel contextual information of speaker embeddings better. Experimental results on the CHiME-7 Mixer6 dataset which only contains partial speakers' labels of the training set, show that our system achieved 57.01% relative DER reduction compared to the clustering-based model on the development set. We further conducted experiments on the CHiME-6 dataset to simulate the scenario of missing partial training set labels. When using 80% and 50% labeled training data, our system performs comparably to the results obtained using 100% labeled data for training.
更多查看译文
关键词
Speaker diarization,semi-supervise,pseudolabel,multi-channel,cross-channel attention
AI 理解论文
溯源树
样例
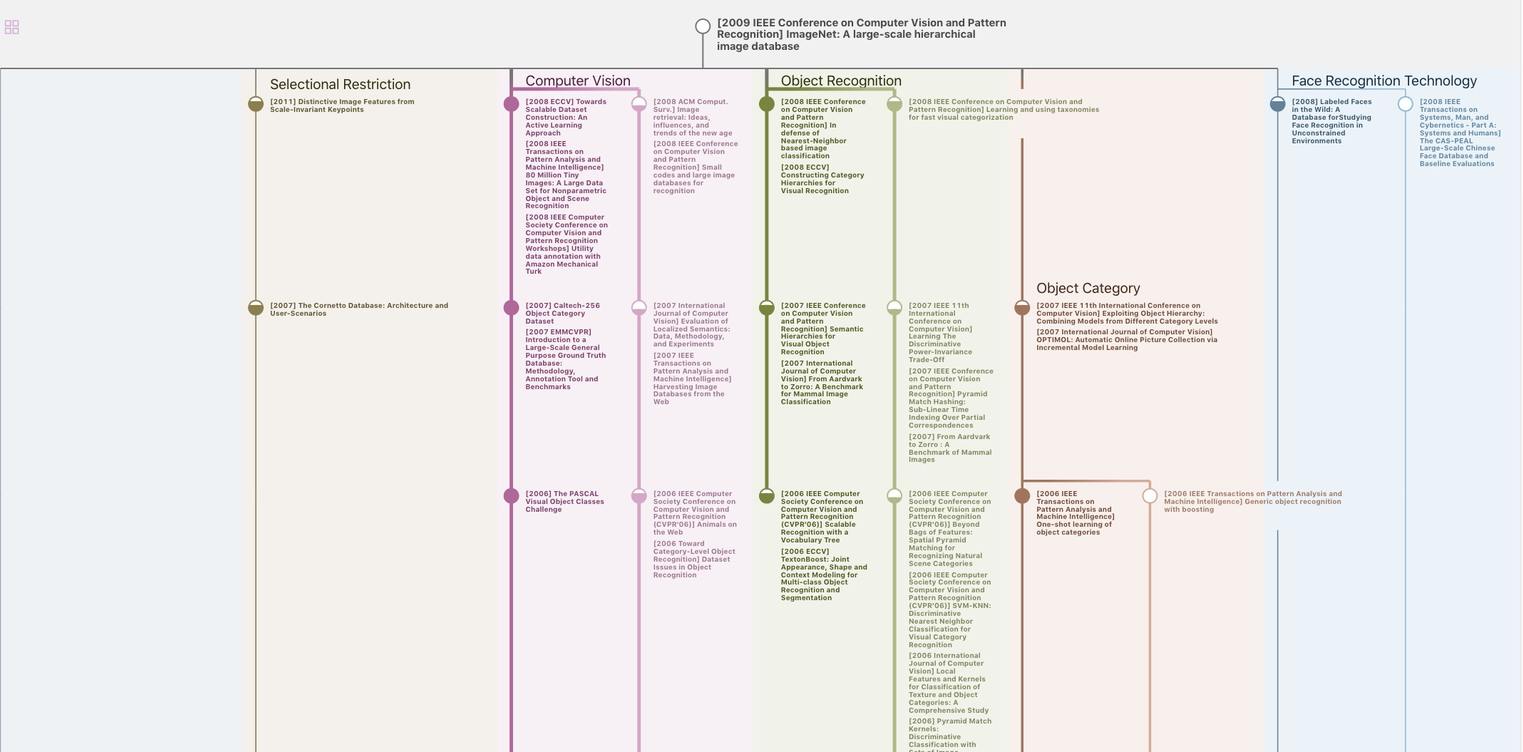
生成溯源树,研究论文发展脉络
Chat Paper
正在生成论文摘要