Applying Different Morphometric Intestinal Mucosa Methods and the Correlation with Broilers Performance under Eimeria Challenge.
Poultry science(2023)
摘要
The intestinal wall has on its surface, protrusions called villi that are responsible for the absorption of nutrients. Commonly, these structures have their dimensions measured to related more area surface with better absorption. However, the measurement of these villi neglects the inflammation and the presence of immature cells that increase the surface area but affect negatively the absorption and compromise the animal performance. The measurements of villi/crypt are traditional tools in animal research; however, they may overlook alterations that impact the mucosal functionality. This study aimed to compare the morphometry of the intestinal villi/crypt with the I See Inside (ISI) scoring methodology, exploring their correlation with zootechnical performance. Therefore, broilers were grouped as nonchallenged (NC) and challenged with Eimeria (CH) and jejunum samples were collected at 22 d for histological analysis. The same villi were submitted to the ISI methodology, which is based on the scoring of 8 parameters related to the inflammatory process, and the measurements of villus height (VH), villus width (VW), crypt depth (CD), crypt width (CW), VH:CD ratio and villi absorptive surface (VAS). The CH group presented higher ISI total score, VW, CD, CW and lower VH, VH:CD, and VAS in comparison to the NC group. While the villi/crypt morphometry did not exhibit correlations with performance, the presence of Eimeria oocysts and the ISI total score was positively correlated (P < 0.05) with the feed conversion ratio (FCR), demonstrating a statistical interaction between high ISI scores and worse performance. In conclusion, a larger villus is not related to better intestinal functionality when this enlargement is unleashed by the immune processes occurring inside. The scoring system that evaluates the type of alteration observed has a direct impact on the animal's zootechnical performance which is not observed with the single metric surface evaluation.
更多查看译文
关键词
histology,gut health,index,coccidiosis,intestinal lesion
AI 理解论文
溯源树
样例
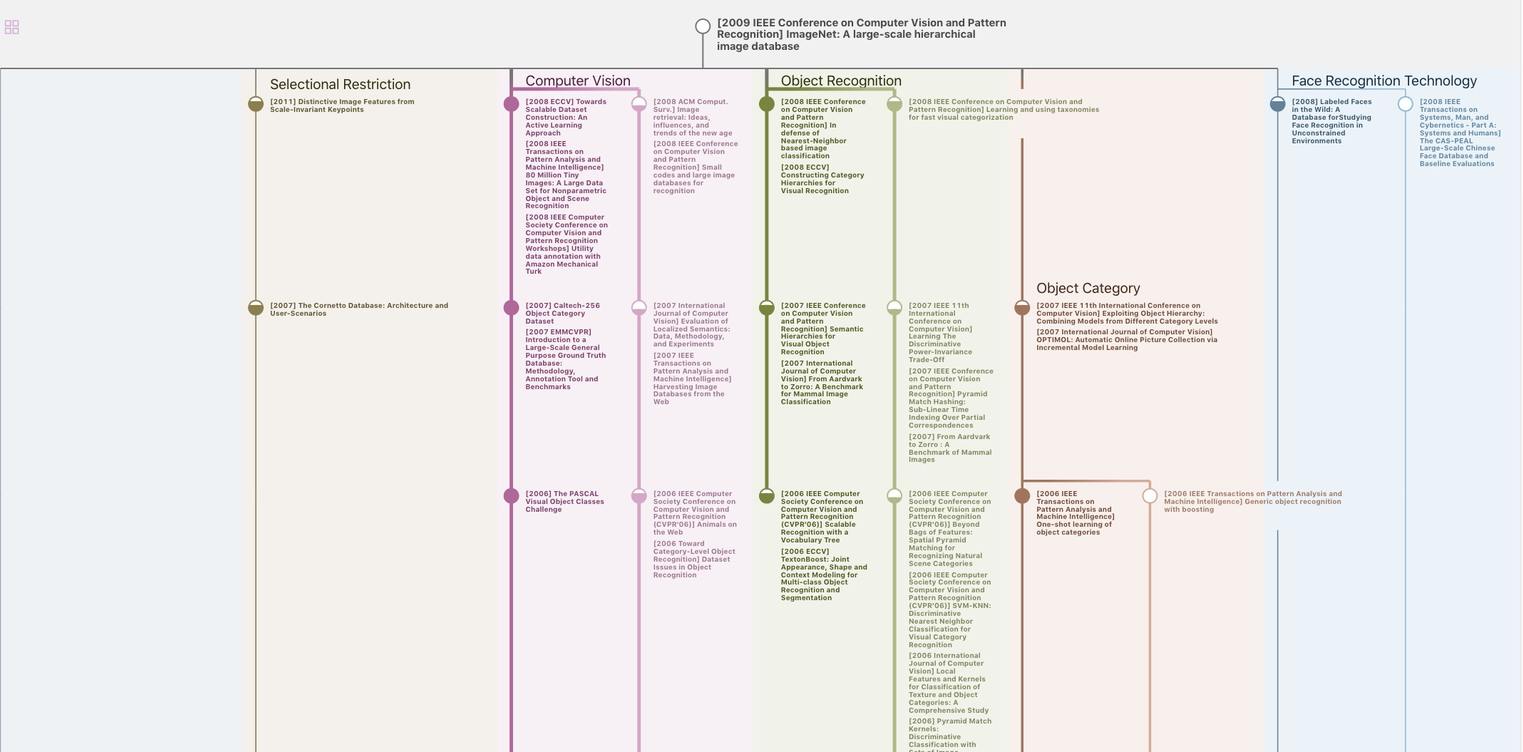
生成溯源树,研究论文发展脉络
Chat Paper
正在生成论文摘要