Deep Learning System for Distinguishing Optic Neuritis from Non-Arteritic Anterior Ischemic Optic Neuropathy at Acute Phase Based on Fundus Photographs
FRONTIERS IN MEDICINE(2023)
摘要
Purpose To develop a deep learning system to differentiate demyelinating optic neuritis (ON) and non-arteritic anterior ischemic optic neuropathy (NAION) with overlapping clinical profiles at the acute phase. Methods We developed a deep learning system (ONION) to distinguish ON from NAION at the acute phase. Color fundus photographs (CFPs) from 871 eyes of 547 patients were included, including 396 ON from 232 patients and 475 NAION from 315 patients. Efficientnet-B0 was used to train the model, and the performance was measured by calculating the sensitivity, specificity, and area under the receiver operating characteristic curve (AUC). Also, Cohen’s kappa coefficients were obtained to compare the system’s performance to that of different ophthalmologists. Results In the validation data set, the ONION system distinguished between acute ON and NAION achieved the following mean performance: time-consuming (23 s), AUC 0.903 (95% CI 0.827–0.947), sensitivity 0.796 (95% CI 0.704–0.864), and specificity 0.865 (95% CI 0.783–0.920). Testing data set: time-consuming (17 s), AUC 0.902 (95% CI 0.832–0.944), sensitivity 0.814 (95% CI 0.732–0.875), and specificity 0.841 (95% CI 0.762–0.897). The performance (κ = 0.805) was comparable to that of a retinal expert (κ = 0.749) and was better than the other four ophthalmologists (κ = 0.309–0.609). Conclusion The ONION system performed satisfactorily distinguishing ON from NAION at the acute phase. It might greatly benefit the challenging differentiation between ON and NAION.
更多查看译文
关键词
Optical Coherence Tomography,Ischemic Optic Neuropathy,Neurophysiological Monitoring,Ocular Findings,Neurodegeneration
AI 理解论文
溯源树
样例
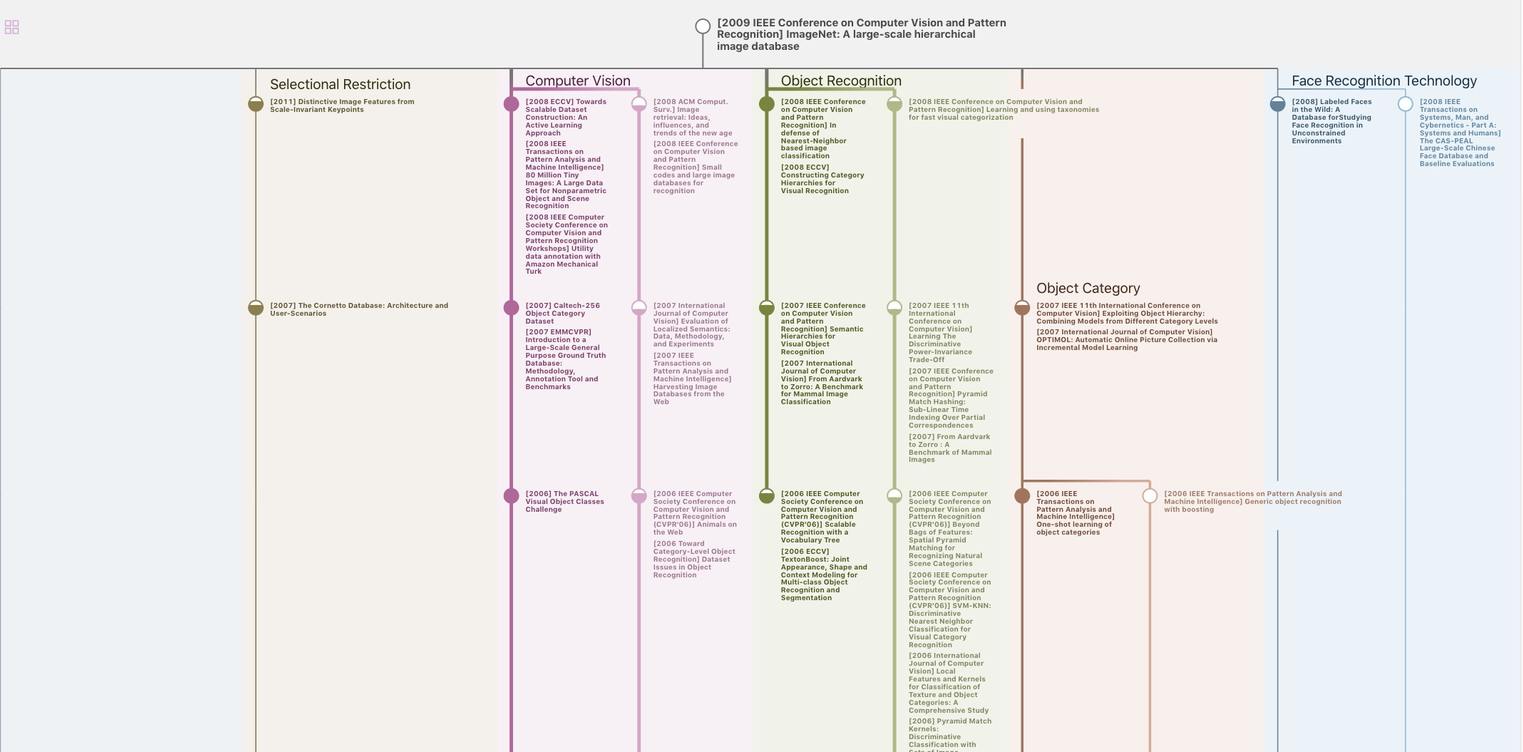
生成溯源树,研究论文发展脉络
Chat Paper
正在生成论文摘要