Information visualization and machine learning driven methods for impedimetric biosensing
TrAC Trends in Analytical Chemistry(2023)
摘要
This review addresses the convergence of impedimetric biosensing technologies and computational methods facilitating data information visualization. The literature brings various methodologies and analytical techniques associated with impedance measurements for biosensing, ranging from versatile testing platforms to management decisions according to the reported detection level. To this end, there has been a growing need for multivariate methods in data analysis, with a steady increase in machine learning methods to determine biosensing parameters. It has been expanded to calibration, analysis, classification, regression procedures, and more recently, calibration space and data inspection rules to optimize the device performance. Consequently, there has been a significant improvement in the automation and accuracy of data, with immediate impacts on diagnostics and protocols in recent years. We focus here on impedimetric biosensing and how multivariate methods combined with machine learning tools (artificial neural network, random forest, decision three, support vector machine, etc.) contribute to the outstanding performance of these devices.
更多查看译文
关键词
Impedance, Information visualization, Machine learning, Biosensor
AI 理解论文
溯源树
样例
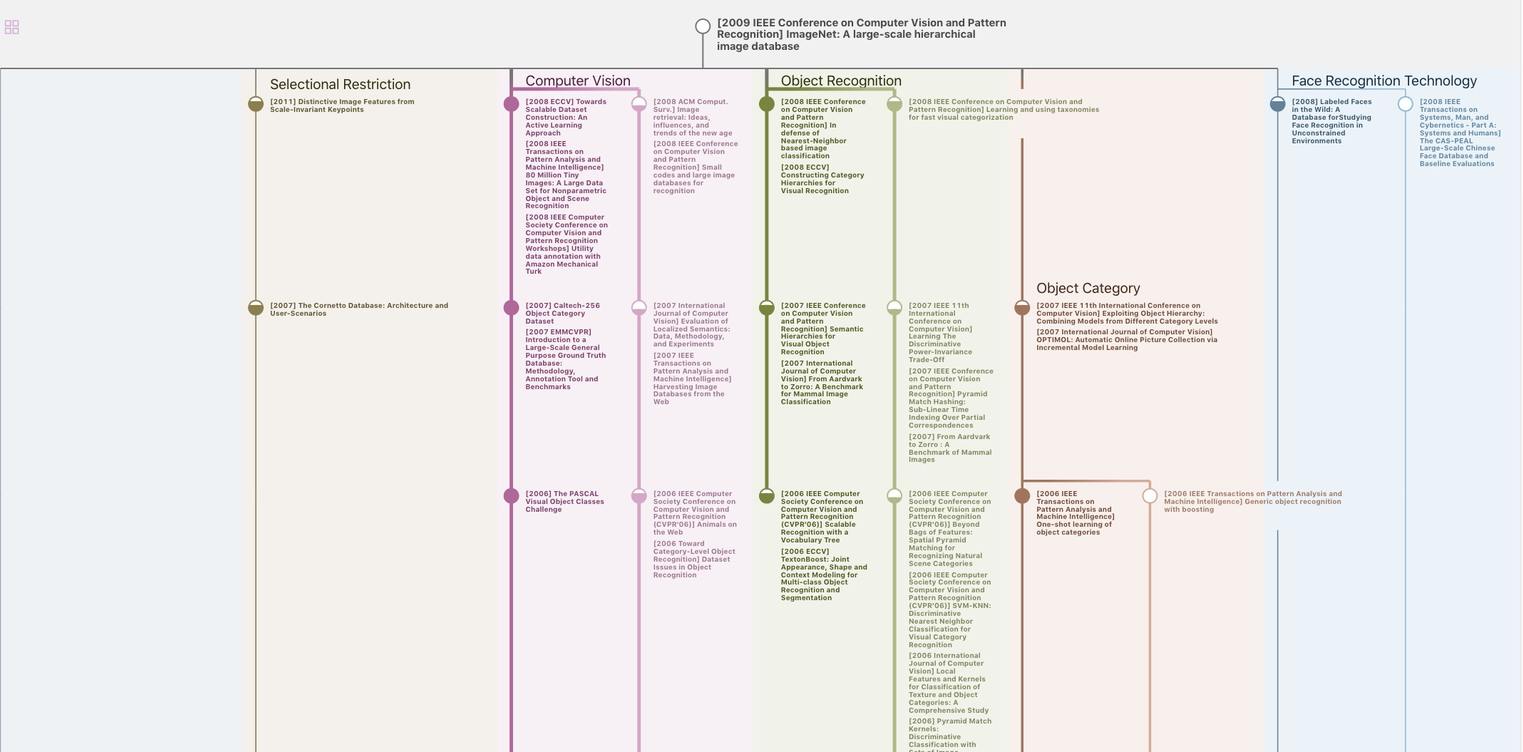
生成溯源树,研究论文发展脉络
Chat Paper
正在生成论文摘要