Predicting Electric Vehicle Charging Demand Using a Heterogeneous Spatio-Temporal Graph Convolutional Network
Transportation research Part C, Emerging technologies(2023)
摘要
Short-term Electric Vehicle (EV) charging demand prediction is an essential task in the fields of smart grid and intelligent transportation systems, as understanding the spatiotemporal distribu-tion of charging demand over the next few hours could help operators of charging stations and the grid to take measures (e.g., dynamic pricing) in response to varying charging demand. This study proposed a heterogeneous spatial-temporal graph convolutional network to predict the EV charging demand at different spatial and temporal resolutions. Specifically, we first learned the spatial correlations between charging regions by constructing heterogeneous graphs, i.e., a geographic graph and a demand graph. Then, we used graph convolutional layers and gated recurrent units to extract spatio-temporal features in the observations. Further, we designed a region-specific prediction module that grouped regions based on graph embedding and point of interest (POI) data for prediction. We used a large real-world GPS dataset which contained over 76,000 private EVs in Beijing for model training and validation. The results showed that, compared with recently popular spatio-temporal prediction methods, the proposed model had superior prediction accuracy and steady performance at different scales of regions. In addition, we conducted ablation studies and hyperparameter sensitivity tests. The results suggested that incorporating the demand graph and geographic graph could help improve model performance.
更多查看译文
关键词
Electric Vehicle,Charging Demand Prediction,Heterogeneous Graph,Spatio-temporal Data Mining,Graph Convolutional Network
AI 理解论文
溯源树
样例
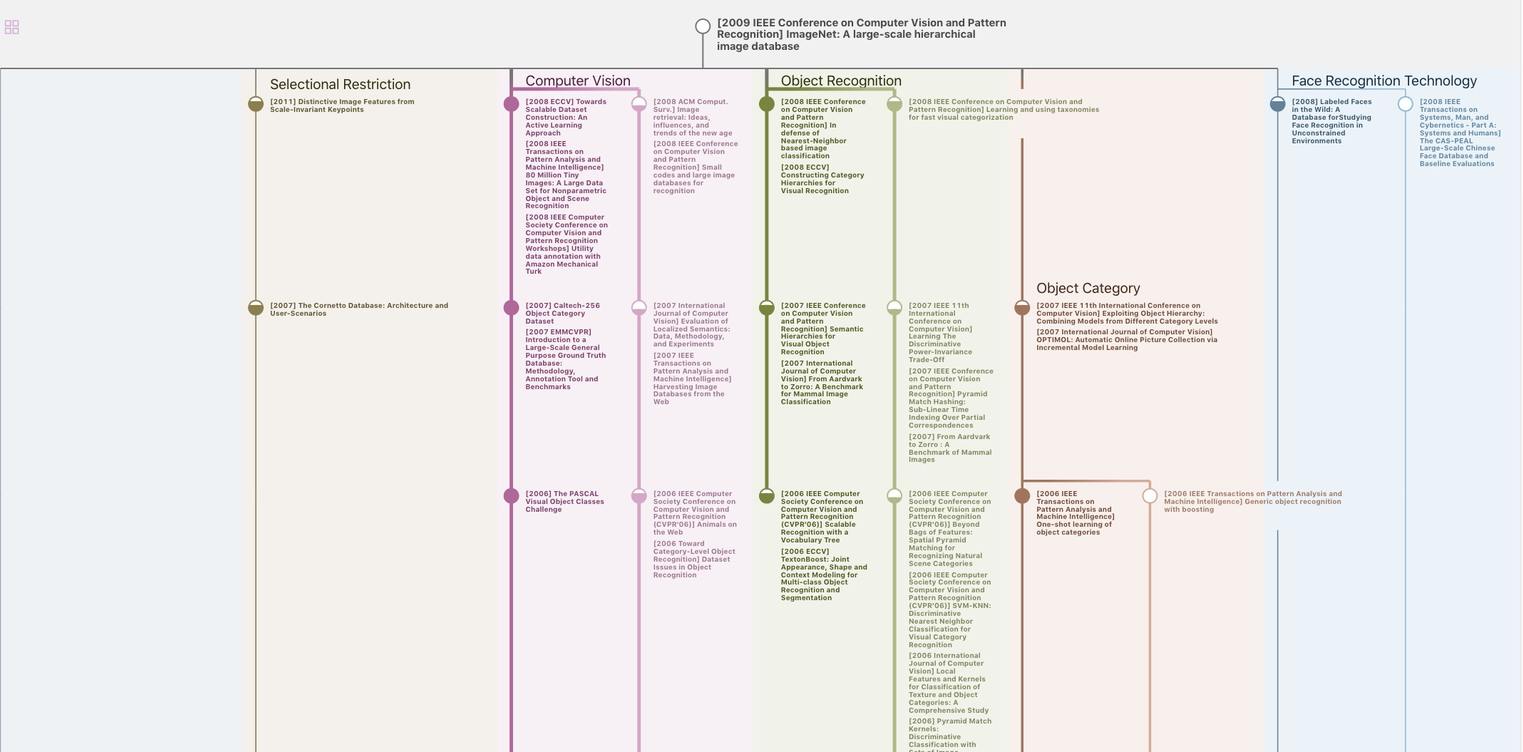
生成溯源树,研究论文发展脉络
Chat Paper
正在生成论文摘要