Scalable and Upgradable AI for Detected Beat-By-Beat ECG Signals in Smart Health
2023 IEEE World AI IoT Congress (AIIoT)(2023)
摘要
The accurate and efficient classification of electrocardiogram (ECG) signals is crucial in identifying cardiac conditions for effective remote heart monitoring and telemedicine systems. However, the large-scale data from various ECG databases presents a challenge to traditional artificial intelligence (AI) algorithms. To address this issue, we use multi-stage classification as a promising method in sHealth. In this study, we aim to evaluate the performance and power consumption of various machine learning and deep learning algorithms in single-stage and multi-stage classification. Specifically, we analyzed beats combined from three different ECG databases and trained decision tree (DT), artificial neural network (ANN), support vector machine (SVM), Naïve Bayes, K-nearest neighbors (KNN), bagged tree, recurrent neural network (RNN), convolutional neural network (CNN), and long short-term memory (LSTM) algorithms using time series feature extraction library (TSFEL). By dividing the data into training and testing sets, we were able to obtain the best accuracy for each algorithm and evaluate their memory usage, CPU usage, and running time. Our study highlights the advantages of multi-stage classification with DT and ANN in accurately detecting cardiovascular diseases while also consuming less power, making it a scalable and upgradable approach. The algorithm developed through this research can be implemented in wearable devices as a pre-trained model to efficiently monitor heart health and detect potential cardiac issues. The use of such IoT devices for remote heart monitoring can significantly improve access to healthcare for patients in remote or underserved areas, allowing for early detection and treatment of cardiovascular diseases.
更多查看译文
关键词
Multi-stage classification,Single-stage classification,Cardiac episodes,Unsupervised monitoring,Machine learning
AI 理解论文
溯源树
样例
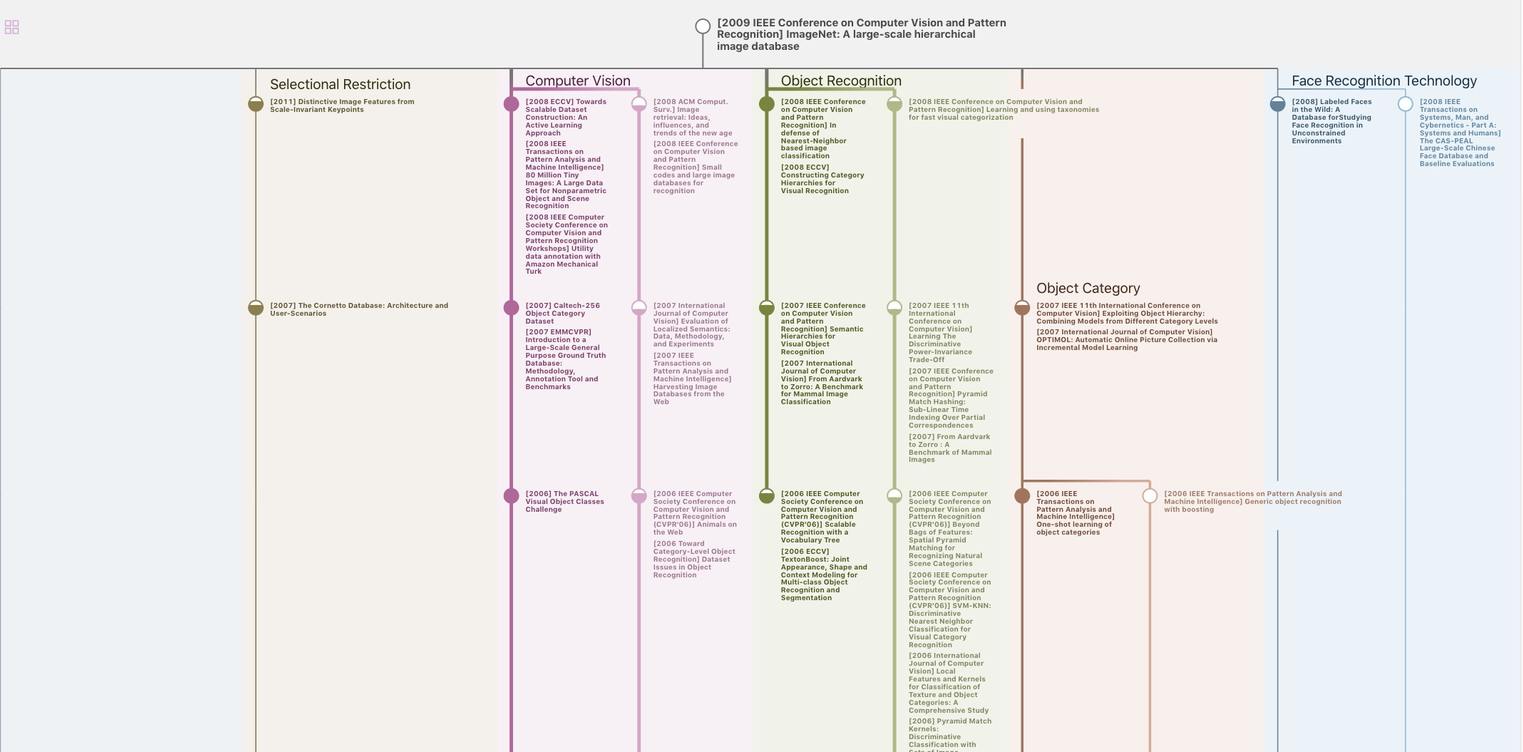
生成溯源树,研究论文发展脉络
Chat Paper
正在生成论文摘要