All SMILES Variational Autoencoder for Molecular Property Prediction and Optimization
Challenges and advances in computational chemistry and physics(2023)
摘要
Variational autoencoders (VAEs)Variational autoencoders defined over SMILES stringSMILES strings and graph-based representations of molecules promise to improve the optimization of molecular properties, thereby revolutionizing the pharmaceuticals and materials industries. However, these VAEs are hindered by the non-unique nature of SMILES stringsSMILES strings and the computational cost of graph convolutions. To efficiently pass messages along all paths through the molecular graphMolecular graph, we encode multiple SMILES stringsSMILES strings of a single molecule using a set of stacked recurrent neural networksRecurrent neural networks (RNNs), harmonizing hidden representations of each atom between SMILES representations, and use attentional pooling to build a final fixed-length latent representation. By then decoding to a disjoint set of SMILES stringsSMILES strings of the molecule, our All SMILES VAE learns an almost bijective mapping between molecules and latent representations near the high probability mass subspace of the prior. Our SMILES-derived but molecule-based latent representations significantly surpass the state of the art in a variety of fully and semi-supervised property regression and molecular property optimization tasks.
更多查看译文
关键词
smiles variational autoencoder,molecular property prediction
AI 理解论文
溯源树
样例
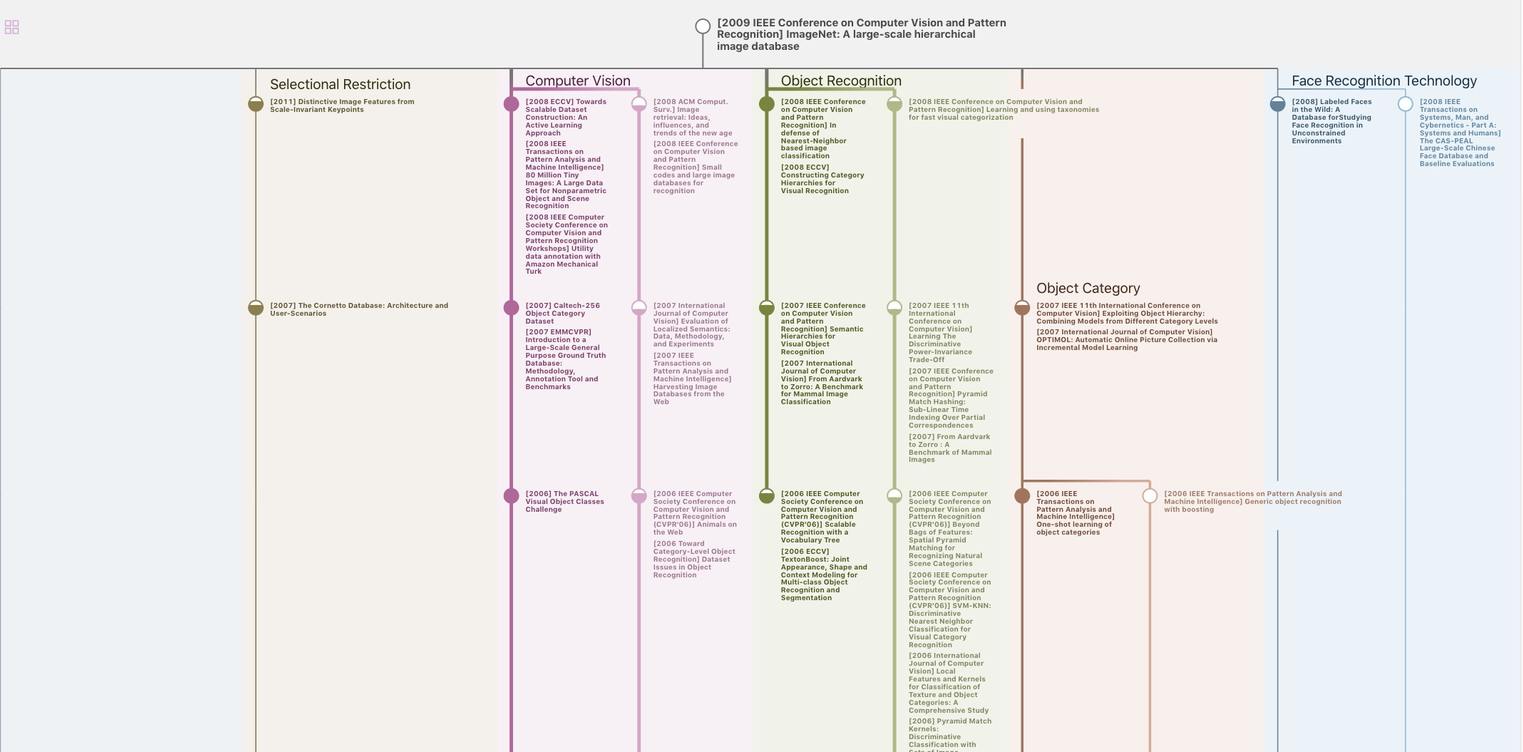
生成溯源树,研究论文发展脉络
Chat Paper
正在生成论文摘要