DARTS: Double Attention Reference-based Transformer for Super-resolution
CoRR(2023)
摘要
We present DARTS, a transformer model for reference-based image super-resolution. DARTS learns joint representations of two image distributions to enhance the content of low-resolution input images through matching correspondences learned from high-resolution reference images. Current state-of-the-art techniques in reference-based image super-resolution are based on a multi-network, multi-stage architecture. In this work, we adapt the double attention block from the GAN literature, processing the two visual streams separately and combining self-attention and cross-attention blocks through a gating attention strategy. Our work demonstrates how the attention mechanism can be adapted for the particular requirements of reference-based image super-resolution, significantly simplifying the architecture and training pipeline. We show that our transformer-based model performs competitively with state-of-the-art models, while maintaining a simpler overall architecture and training process. In particular, we obtain state-of-the-art on the SUN80 dataset, with a PSNR/SSIM of 29.83 / .809. These results show that attention alone is sufficient for the RSR task, without multiple purpose-built subnetworks, knowledge distillation, or multi-stage training.
更多查看译文
关键词
transformer,attention,reference-based,super-resolution
AI 理解论文
溯源树
样例
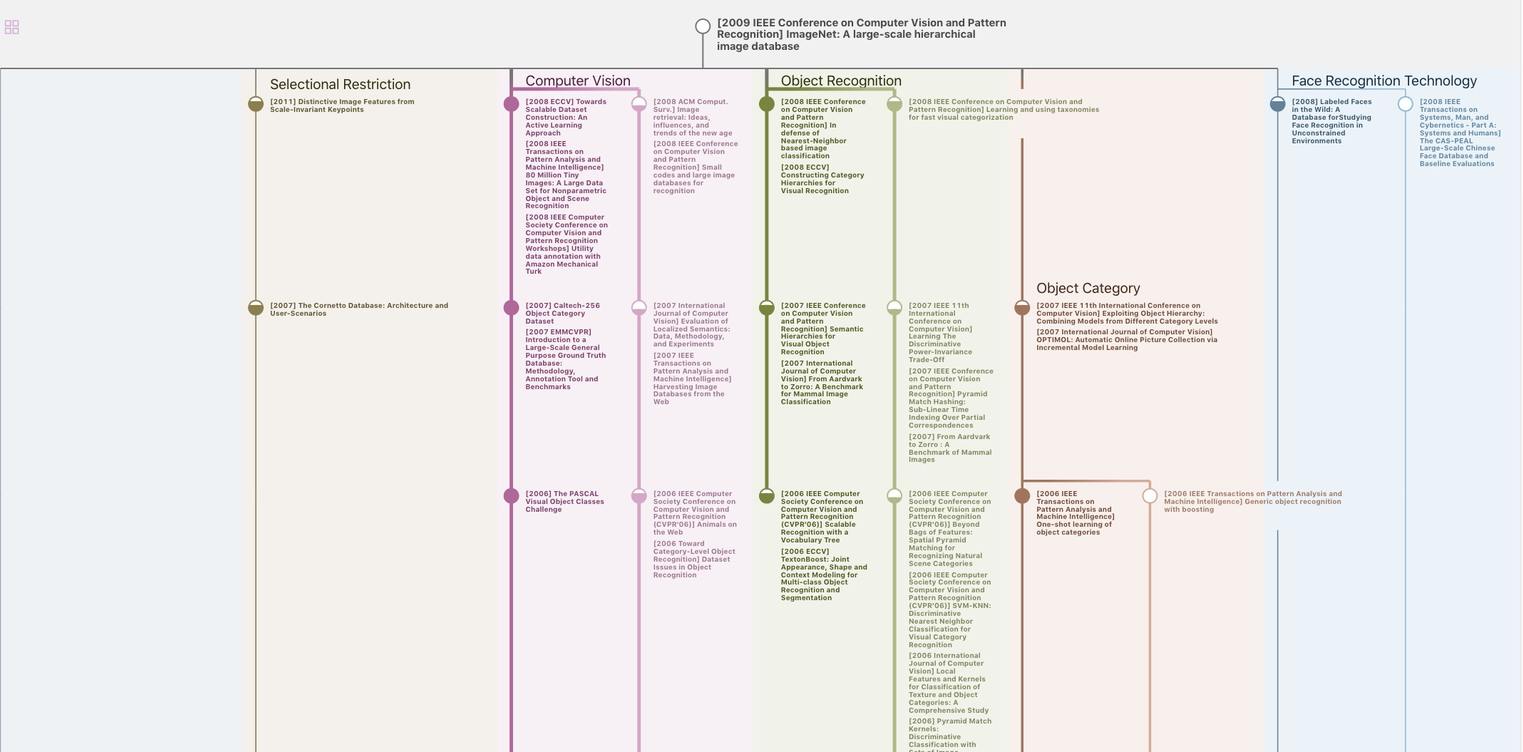
生成溯源树,研究论文发展脉络
Chat Paper
正在生成论文摘要