Letter: Deep Neural Networks Can Accurately Detect Blood Loss and Hemorrhage Control Task Success from Video.
Neurosurgery(2023)
摘要
To the Editor: We read with great interest the article by Kugener et al.1 We would like to congratulate the authors for successfully developing a novel pipeline using deep neural network methodologies to predict blood loss and other clinical outcomes of hemorrhage control from 123 training videos of iatrogenic internal carotid artery injury repair with great accuracy. Of great interest to us is that Kugener et al used a Long Short-Term Memory (LSTM) Recurrent Neural Network (RNN) as a component of their deep neural network pipeline to predict task success after feature generation and outcome prediction from individual video frames. The roles of RNN and LSTM models as deep learning architectures optimized for time series analyses have been well-established.2-4 Nevertheless, they have received limited attention in neurosurgery. Although Kugener et al have explored RNN in deep learning–based video analysis pipelines,1 these architectures may be applicable to other fields of neurosurgery, including sequential data for time series analyses.5 In this letter, we expound on the current state and future potential of time series–based model architectures for machine learning analyses in the field of neurosurgery. AN OVERVIEW OF TIME SERIES MACHINE LEARNING Time series analyses are important in prediction algorithms where earlier disease states will contribute greatly to prognostication of future disease states. Specific to neurosurgery, a hidden Markov model predicted partial brain tissue oxygenation crises 30 minutes in advance6 and multivariate regression and Gaussian processes predicted episodes of raised intracranial pressure.7 However, these classical regression methods may not capture the complexity of temporal data comprehensively. By contrast, contemporary machine learning algorithms are robust techniques capable of accounting for the prognostic significance of previous input states, hence establishing themselves as competitive alternatives to classical regression methods.8 Techniques in machine learning literature for dynamic forecasting encompass RNNs with LSTM or gated recurrent units (GRUs). These algorithms can learn dependencies from past “long-term” inputs and recent “short-term” inputs in RNNs. However, they cannot effectively manage irregular time series data and missing data, which are common occurrences in medical data sets. Novel state-of-the-art deep learning architectures have thus emerged to tackle this problem, including GRU-D,9 a GRU model with trainable decays for missing values, and phased LSTM,10 an LSTM model that adds a new time gate to the existing RNN. These have been successful at handling missing parameters and addressing sparse and asynchronous data. Recently, there has also been a growing interest in using ordinary differential equations for time series predictions, which has since yielded excellent results. In 2018, Chen et al11 published a landmark article documenting their success in handling irregularly spaced observations by using a continuous-time modeling design and modeling temporal states using Neural Ordinary Differential Equations (ODEs). Neural ODE models may be used on data containing irregular time series intervals, such as healthcare data, because they can decode the underlying differential equations with great performance and accuracy. A GRU-ODE-Bayes model has been used successfully to project multiple sclerosis disability progression within a 2-year horizon.12 As this field develops, latest research has expanded to solve a wider variety of differential equations using the Neural Laplace framework, which uses the Laplace domain to represent solutions.13 These models can capture differential equations containing historical states, which is advantageous when processing long-range discontinuities frequently observed in clinical and laboratory features.13 APPLICATION OF TIME SERIES MODEL ARCHITECTURES IN NEUROSURGERY In neurosurgery, time series models may be applicable for intracranial pressure monitoring and prediction, a research question recently explored by Schweingruber et al.5 Despite the diversity of time series machine learning methods with varying applications, their use in neurosurgery has remained limited. This may be attributable to the black box nature of machine learning models, which limits their interpretability and, thus, applicability. While Schweingruber et al were able to assign an importance weight to each feature by calculating gradient-based feature importance using SmoothGrad and Integrated Gradients,5,14,15 it has been shown that the magnitude of feature importance weights may not necessarily represent a feature's influence over the model's output.16 Hence, the use of feature importance weights to interpret data-driven decisions made by black box models may be inadequate. To this end, there has been increasing interest to incorporate explainable AI into time series models, such as Temporal Fusion Transformers,17 which accounts for the time-based order of features and identifies persistent time patterns. By inheriting both quantitative and qualitative approaches to model evaluation,18 the growing field of time series explainable AI has promising potential to catalyze novel and practical applications of time series machine learning in neurosurgery. In conclusion, time series analyses and predictions offer additional clinical insights and may play an important role in the future of clinical practice. The efforts of Kugener et al in developing a video-based LSTM model highlight one possible application of time series machine learning in neurosurgery. As these models become more reliable and interpretable, their role as clinician decision support systems may expand. Having increasingly precise models will extend the utility of time series predictions to fields ranging from neurosurgical critical care monitoring, trending biomarkers in neurological disease,14,19 to identifying surgery outcome through video prediction. We hope that this letter will be complementary to Kugener et al and the field of machine learning and time series analysis in neurosurgery.
更多查看译文
关键词
hemorrhage control task success,blood loss,deep neural networks,neural networks
AI 理解论文
溯源树
样例
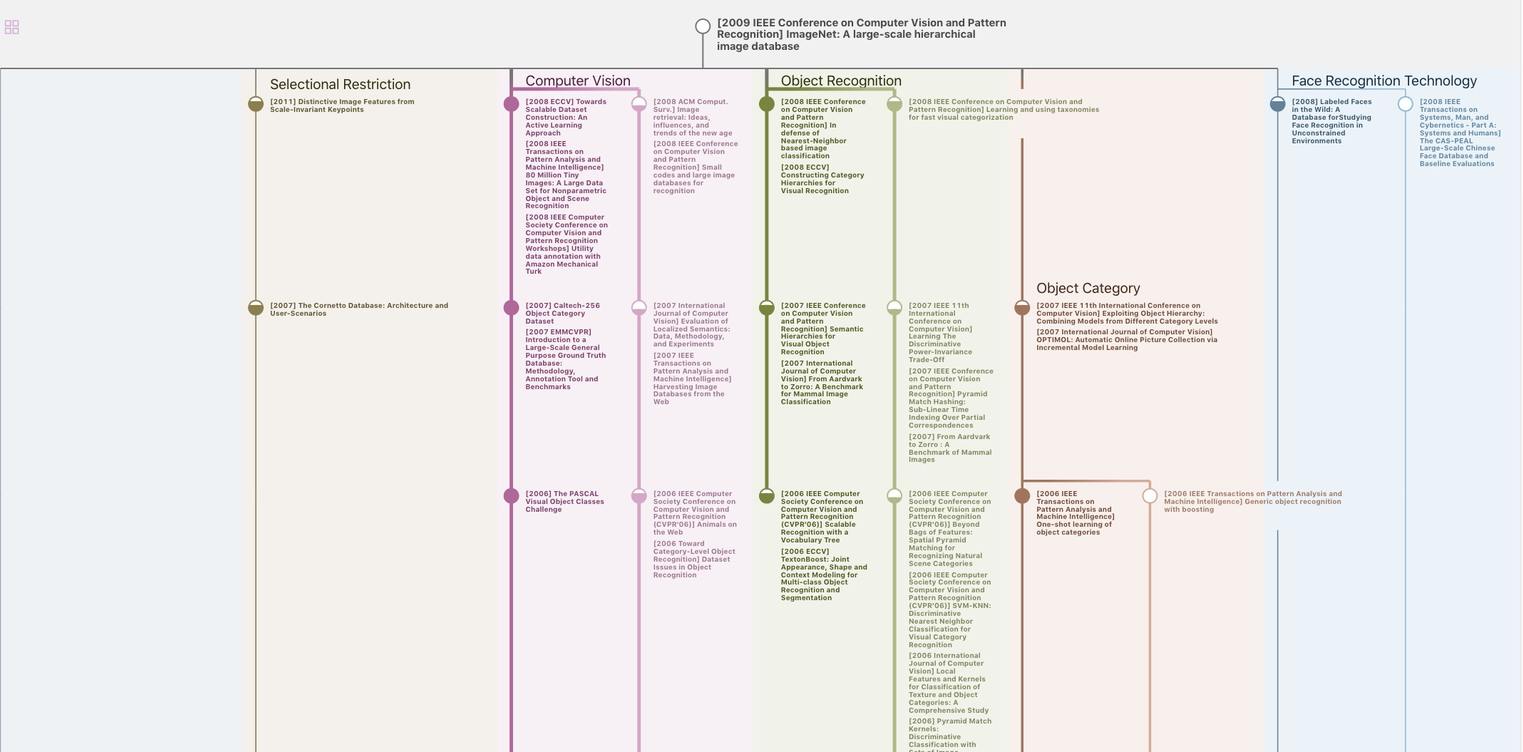
生成溯源树,研究论文发展脉络
Chat Paper
正在生成论文摘要