A cumulative descriptor enhanced ensemble deep neural networks method for remaining useful life prediction of cutting tools
Advanced Engineering Informatics(2023)
摘要
Prognostics and health management (PHM) of turbine cutting tools, particularly the remaining useful life (RUL) prediction is a Gordian technique to maintain the reliability and availability of the turbine, which is also a method to reduce unexpected downtime and total machined costs. Nowadays, most of the recent works in RUL prediction neglect the quality of data used for model training, assuming that the collected sensor data and extracted features can both reflect the degradation process well, which is not always true. This paper firstly introduces a novel cumulative descriptor to better represent the degradation process of cutting tools and further proposes a well-designed stacking-based deep neural network (S-DNN) that contains several different neuron architectures and an ensemble model to construct deep learning layer and ensemble learning layer. Cumulative descriptors are constructed only using directly measured values extracted from raw sensor data, and then the constructed novel features will be fed into S-DNN to extract abstract features from sensor data in multiple ways and automatically learn the relationship between the outputs of deep learning layer and the ground truth by training the ensemble model iteratively. Case studies on experimental data set and NASA Ames milling data set indicate that the proposed method has great effectiveness and generalization in RUL prediction tasks.
更多查看译文
关键词
Remaining useful life (RUL), Cumulative descriptors, Stacking-based deep neural network (S-DNN), Turbine cutting tools, Ensemble learning
AI 理解论文
溯源树
样例
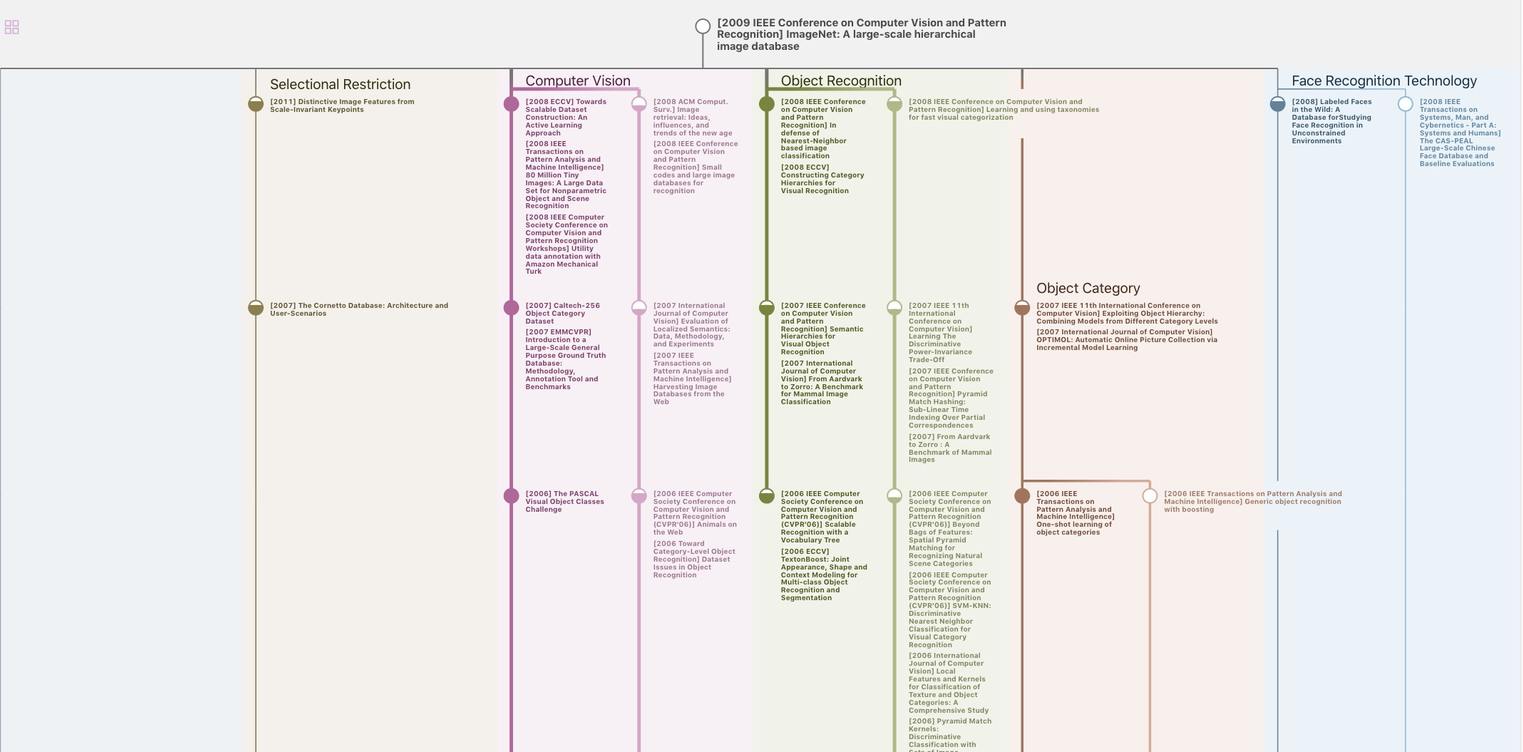
生成溯源树,研究论文发展脉络
Chat Paper
正在生成论文摘要