Improvement of a deterministic fuel management code using artificial neural network for liquid-fueled molten salt reactor
Annals of Nuclear Energy(2023)
摘要
The fuel management code of liquid-fueled molten salt reactor (MSR) relies on the coupling of the neutronics code with depletion code. The deterministic method can improve the efficiency of neutronics calculation. However, updating the microscopic cross-sections in depletion calculation is still time-consuming using Monte Carlo code. A surrogate model based on artificial neural network (ANN) is developed to update the cross-sections online. Then a new fuel management code named ThorNEMFM-ANN was developed by integrating the surrogate model into the deterministic fuel management code. The surrogate model and ThorNEMFM-ANN code were validated with the molten salt breeder reactor (MSBR) and molten salt fast reactor (MSFR) benchmarks. Furthermore, the ThorNEMFM-ANN was applied to the fuel cycle analysis of a molten chloride salt fast reactor (MCSFR) for the demonstration of the applicability. The numerical results indicate that the surrogate model is feasible and effective in the fuel cycle simulation of liquid-fueled MSR.
更多查看译文
关键词
Molten salt reactor,Cross-section,Surrogate model,Artificial neural network,Fuel management
AI 理解论文
溯源树
样例
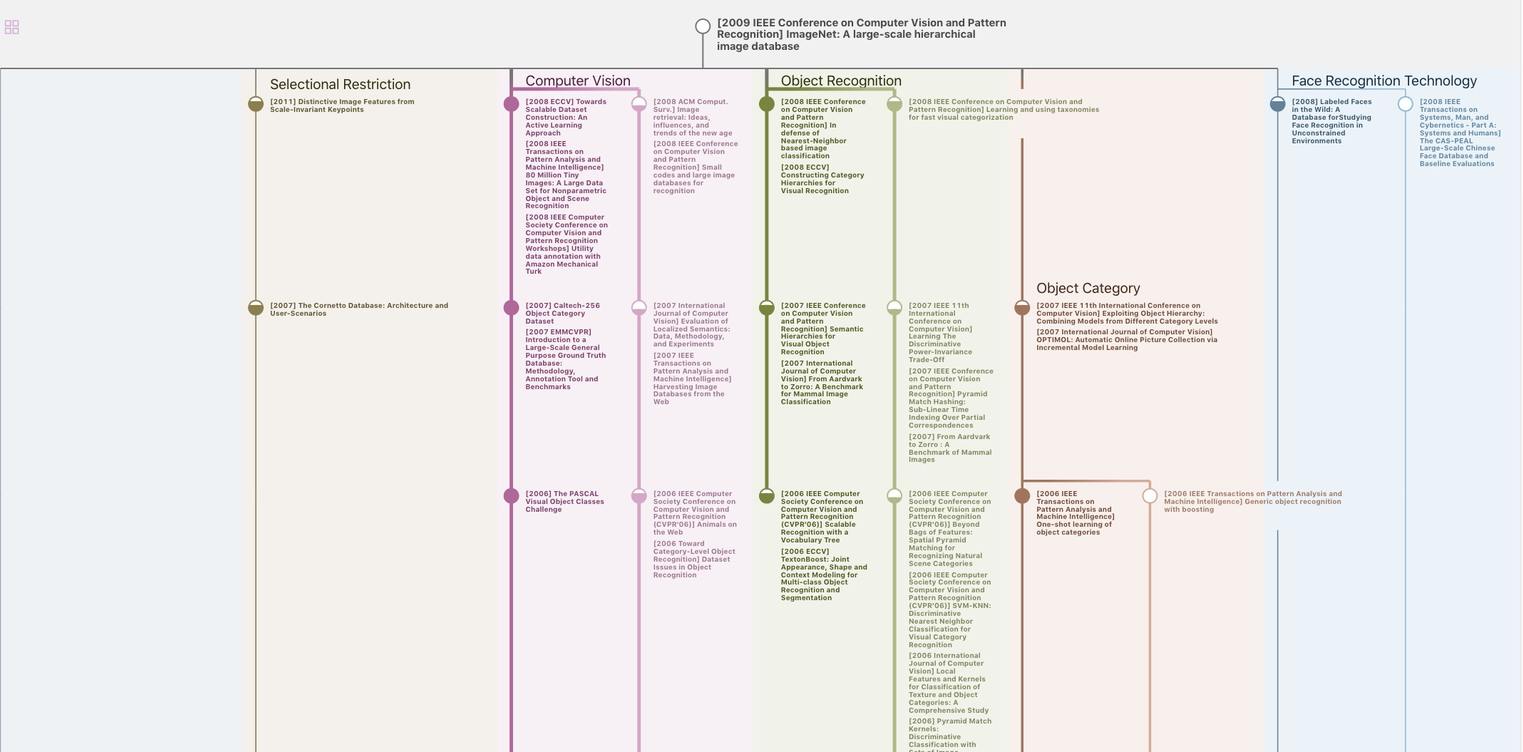
生成溯源树,研究论文发展脉络
Chat Paper
正在生成论文摘要