Human activity recognition in an end-of-life consumer electronics disassembly task
Applied Ergonomics(2023)
摘要
The production of electronic waste, also known as e-waste, has risen with the growing reliance on electronic products. To reduce negative environmental impact and achieve sustainable industrial processes, recovering and reusing products is crucial. Advances in AI and robotics can help in this effort by reducing workload for human workers and allowing them to stay away from hazardous materials. However, autonomous human motion/ intention perception is a primary barrier in e-waste remanufacturing. To address the research gap, this study combined experimental data collection with deep learning models for accurate disassembly task recognition. Over 570,000 frames of motion data were collected from inertial measurement units (IMU) worn by 22 participants. A novel sequence-based correction (SBC) algorithm was also proposed to further improve the accuracy of the overall pipeline. Results showed that models (CNN, LSTM, and GoogLeNet) had an overall accuracy of 88-92%. The proposed SBC algorithm improved accuracy to 95%.
更多查看译文
关键词
e -waste disassembly,Task recognition,Deep learning methods
AI 理解论文
溯源树
样例
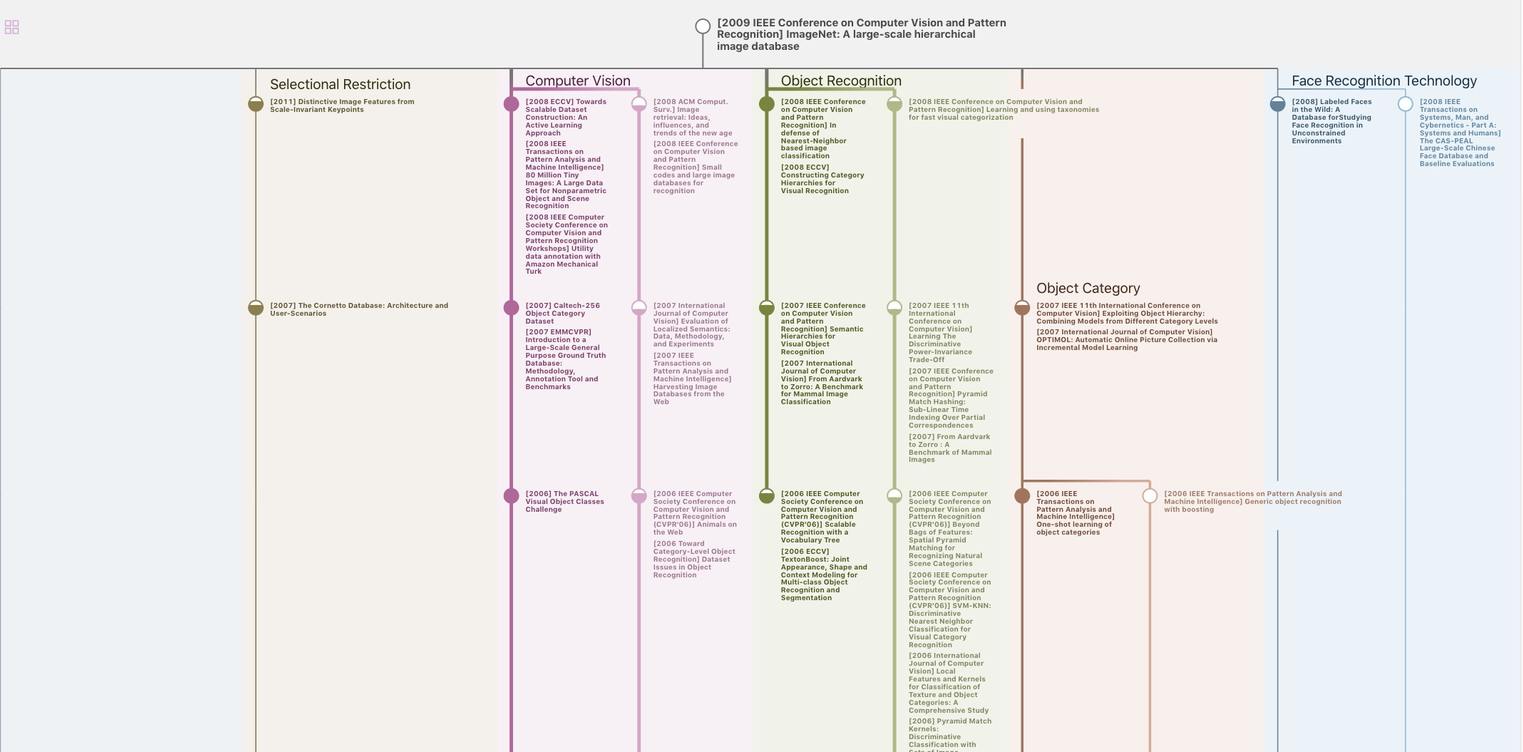
生成溯源树,研究论文发展脉络
Chat Paper
正在生成论文摘要