Learn global and optimize local: A data-driven methodology for last-mile routing
Computers & Operations Research(2023)
摘要
In last-mile routing, the task of finding a route is often framed as a Traveling Salesman Problem to minimize travel time and associated cost. However, solutions stemming from this approach do not match the realized paths as drivers deviate due to navigational considerations and preferences. To prescribe routes that incorporate this tacit knowledge, a data-driven model is proposed that aligns well with the hierarchical structure of delivery data wherein each stop belongs to a zone — a geographical area. First, on the global level, a zone sequence is established as a result of a minimization over a cost matrix which is a weighted combination of historical information and distances (travel times) between zones. Subsequently, within zones, sequences of stops are determined, such that, integrated with the predetermined zone sequence, a full solution is obtained.
更多查看译文
关键词
Last-mile logistics, Route prediction, Navigation, Traveling salesman problem, Data-driven optimization
AI 理解论文
溯源树
样例
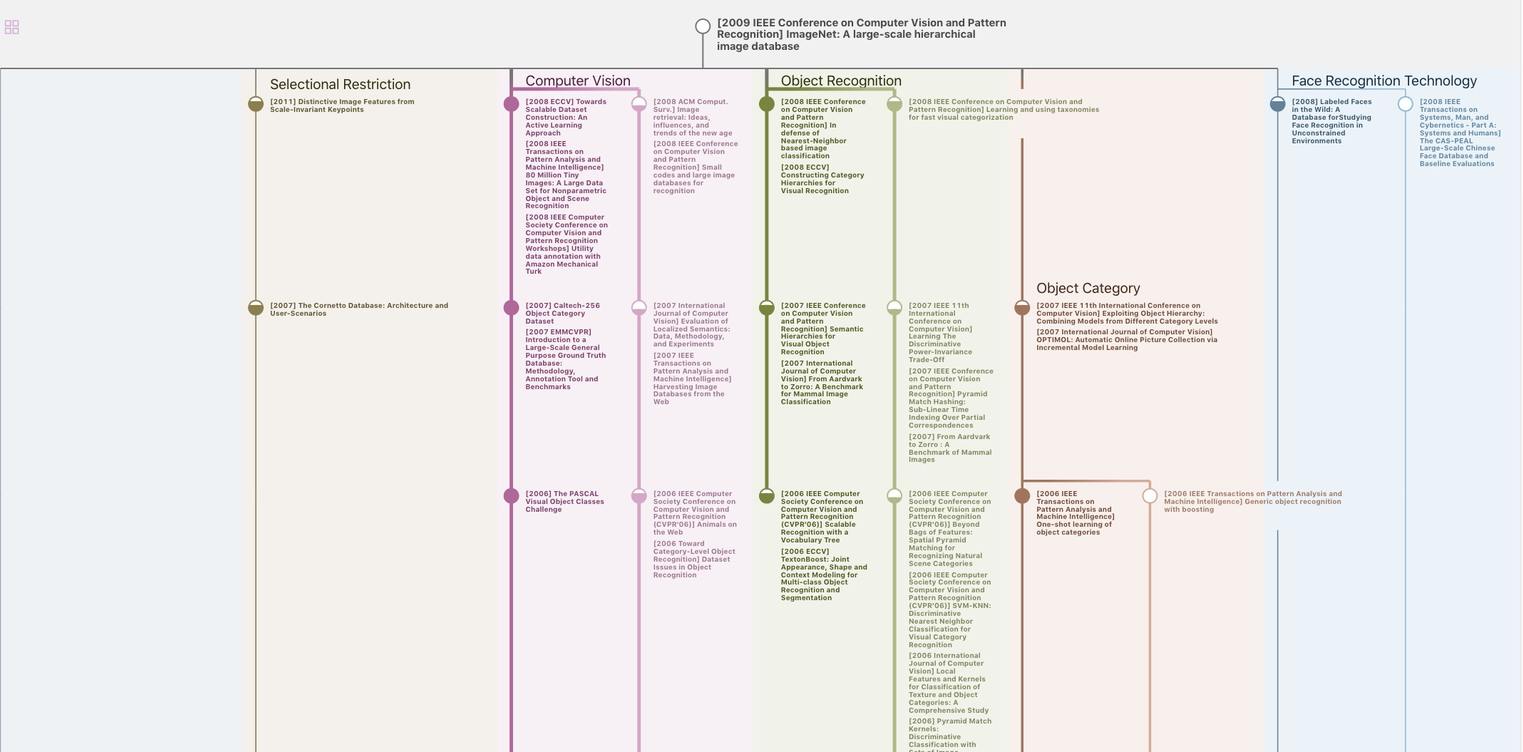
生成溯源树,研究论文发展脉络
Chat Paper
正在生成论文摘要