Learning from single-defect wafer maps to classify mixed-defect wafer maps
Expert Systems with Applications(2023)
摘要
Automation of wafer map pattern classification has been an active research topic for identifying the root causes of semiconductor manufacturing processes. Building a classification model is a primary challenge owing to the high cost of labeling wafer maps with their defect categories to create a training dataset. Single-defect wafer maps are relatively easy to label because their patterns are simple and clear. However, labeling mixed-defect wafer maps is difficult and time-consuming because they often have complex and ambiguous patterns. This study aims to address the problem of data insufficiency in which only normal and single-defect wafer maps are present in the training dataset, whereas mixed-defect wafer maps are also present in the test dataset. We propose a method for training a convolutional neural network (CNN) by using a training dataset of normal and single-defect wafer maps to accurately classify mixed-defect wafer maps. The proposed method utilizes three components to generate synthetic wafer maps from the training dataset: mixup, random rotation, and noise filtering. Mixup combines single-defect wafer maps to create synthetic mixed-defect wafer maps. Random rotation increases the diversity of the synthetic wafer maps. Noise filtering prevents the accumulation of random noise in synthetic wafer maps. The CNN is trained with the synthetic wafer maps generated through the proposed method, which include normal, single-defect, and mixed-defect variations. Through experimental investigation using the MixedWM38 dataset, we demonstrate that the proposed method significantly outperforms the existing methods.
更多查看译文
关键词
Wafer map pattern classification,Mixed-defect patterns,Multi-label classification,Data synthesis
AI 理解论文
溯源树
样例
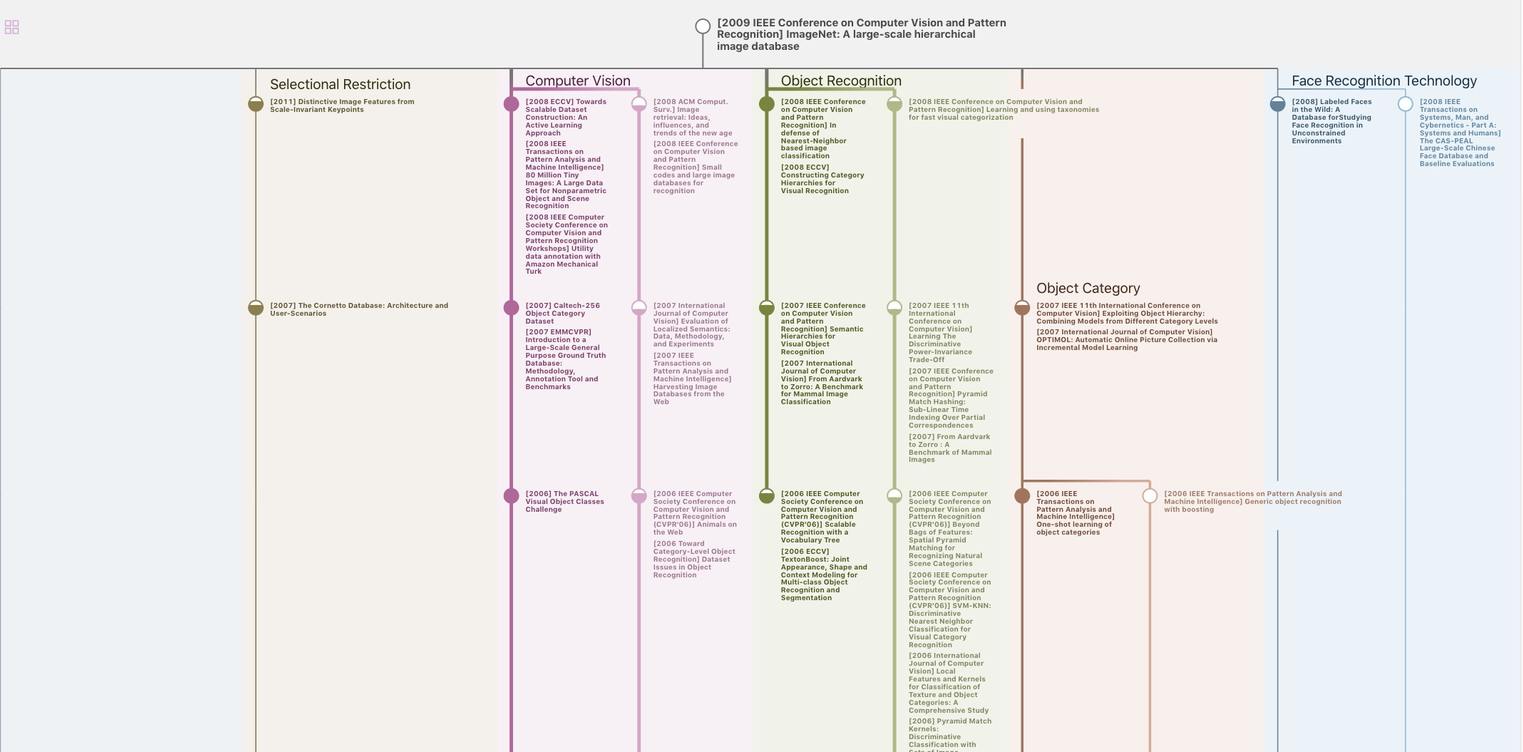
生成溯源树,研究论文发展脉络
Chat Paper
正在生成论文摘要