Infrared Spectroscopic Evaluation for Catalytic Hydrogenation of Biomass and Coal Using Unsupervised and Supervised Algorithms
FUEL(2023)
Abstract
Three biomass (rice straw, rice husk and wheat straw) and three coal samples (lignite, subbituminous coal and bituminous coal) were extracted via a thermal dissolution (TD) process, and the TD extracts were treated by catalytic hydrogenation to obtain reaction products. To reveal the similarity in molecular information among the samples and elucidate their chemical reactivity, four machine learning algorithms were applied to analyze the Fourier transform infrared spectra of both TD extracts and catalytic hydrogenation products. Functional groups were used as variables and the difference in peak area can be treated as the basis for sample classification. Aromatic CH, COC, aliphatic CH2 or CH3 and aromatic CO or CC bonds were the main characteristic variables in principal component analysis algorithm to classify biomass- and coal-derivated samples. These samples were also grouped into four clusters by hierarchical clustering analysis algorithm according to the similarity and difference in the distribution of functional groups. For artificial neural network algorithm, aliphatic CH and OH bonds are the most important variables to classify these samples into four groups, and aromatic CH, OH, and COC groups are the main variables contributed to the classification trees in random forest algorithm. Machine learning algorithms will provide methodological guidance for the data mining of the spectra of complex organic systems.
MoreTranslated text
Key words
Biomass,Coal,Catalytic hydrogenation,Unsupervised algorithm,Supervised algorithm
AI Read Science
Must-Reading Tree
Example
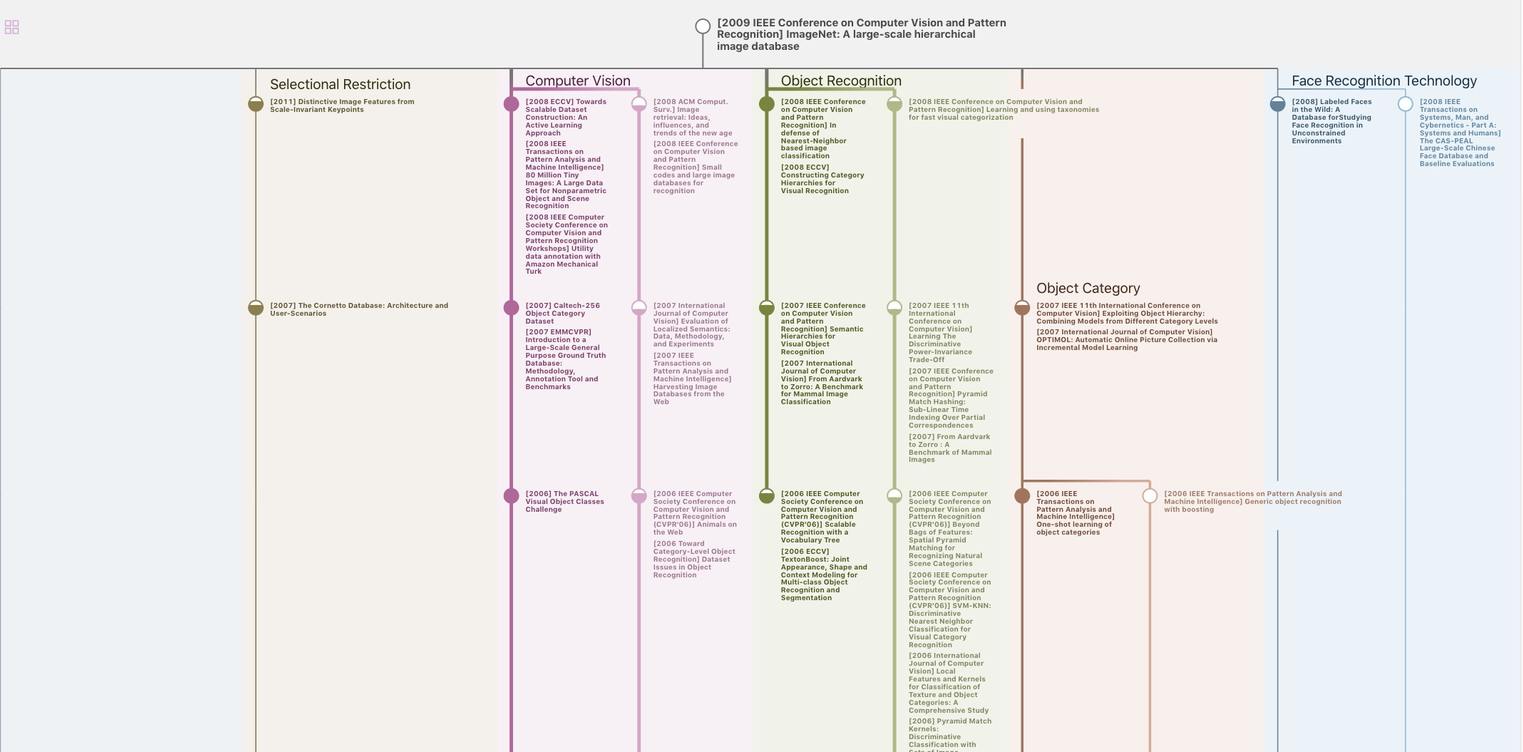
Generate MRT to find the research sequence of this paper
Chat Paper
Summary is being generated by the instructions you defined