Joint representation and classifier learning for long-tailed image classification
Image and Vision Computing(2023)
摘要
Long-tailed classification with fine-grained appearance, e.g., in chest X-ray images, is very challenging due to the very similar appearance and imbalanced distribution between normal and abnormal samples, which extremely limits the ability of deep networks to learn powerful representations and discriminative classifiers. In this paper, we propose a novel Joint Representation and Classifier Learning (JRCL) framework to achieve the above purposes, simultaneously. In terms of representation learning, we propose a One-to-All supervised contrastive learning strategy to avoid the medium or tail classes mixing in the head classes. For the classifier cleaning, we propose a novel Binary Distribution Consistency (BDC) loss to learn a discriminative classifier that could separate the normal and abnormal samples.The BDC loss measures the binary distribution consistency between the designed multi-class classifier and an auxiliary binary classifier. Consequently, the JRCL framework is optimized with a supervised contrastive learning loss, a binary distribution consistency loss, and a multi-classification loss. We conduct experiments on large-scale, long-tail image datasets, NIH-CXR-LT, MIMIC-CXR-LT, iNaturalist 2018, and Places-LT. Experimental results demonstrate JRCL could improve the discriminate ability of the imbalanced data and thus obtain better classification performance. Compared with the state-of-the-art methods, our proposed JRCL achieves comparable or even better performance. The source codes are available at https://github. com/guanqj932/JRCL.
更多查看译文
关键词
Long-tailed image classification,Representation learning,Classifier learning,Supervised contrastive learning
AI 理解论文
溯源树
样例
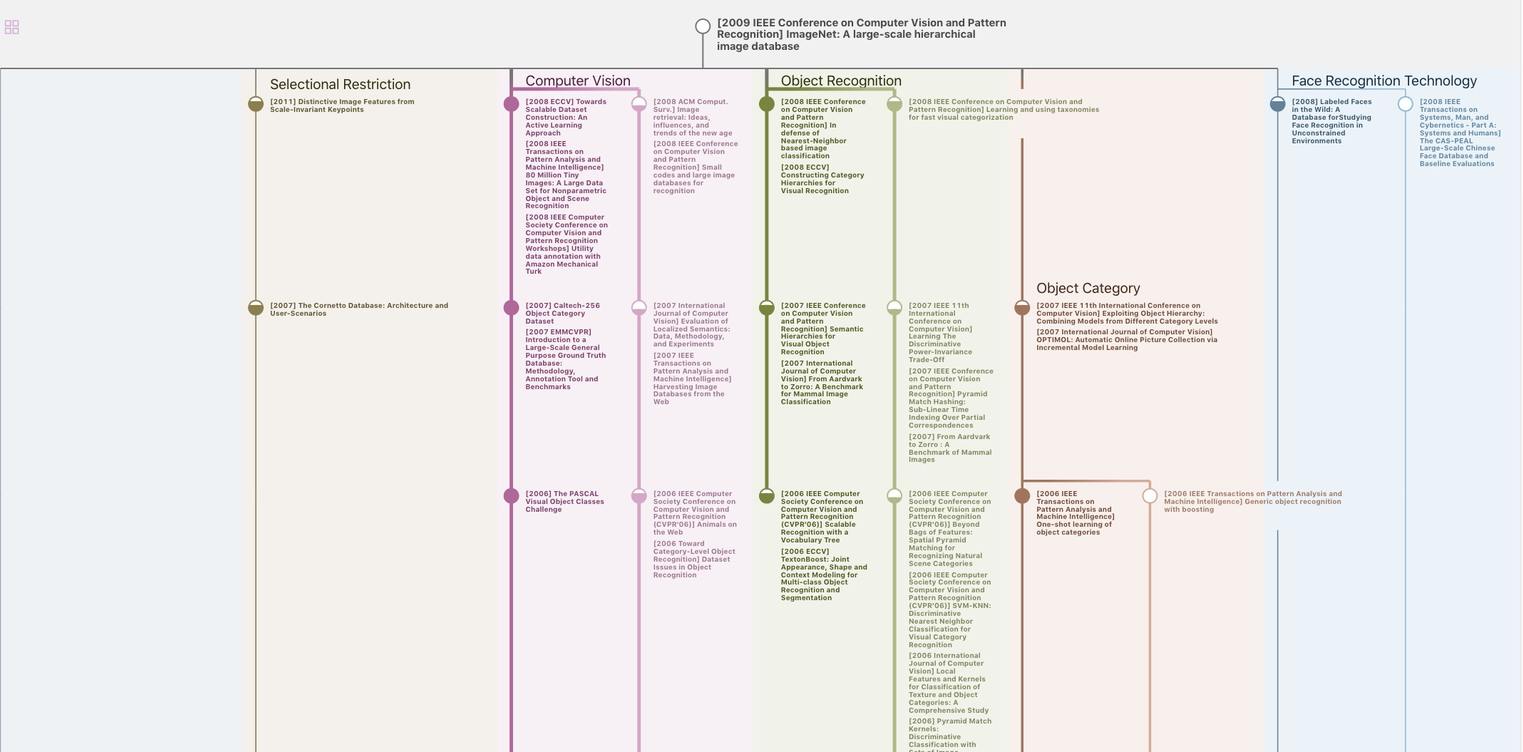
生成溯源树,研究论文发展脉络
Chat Paper
正在生成论文摘要