An explainable machine learning for geographical origin traceability of mussels Mytilus edulis based on stable isotope ratio and compositions of C, N, O and H
Journal of Food Composition and Analysis(2023)
摘要
Geographical traceability of marine bivalves has been explored continually, however the traceability results have not been recognized owing to the absence of a reasonable explanation for a certain model prediction. To address this problem, we employed an explainable machine learning to identify the origin of mussels based on stable isotope ratio and compositions of carbon (C), nitrogen (N), oxygen (O), and hydrogen (H). Our findings proved that the Extreme gradient boosting (XGBoost) model may be the best model based upon its high accuracy (93.75 %), precision (93.75 %), recall (94.51 %), F1 score (93.72 %) and AUC (0.990). The feature impact for model out of XGBoost was interpreted by SHapley Additive exPlanations (SHAP) on global and local perspective. This study demonstrated the potential of explainable machine learning to elucidate the complex relationships between desirable geographical information of mussels and the selected features.
更多查看译文
关键词
mussels mytilus edulis,geographical origin traceability,stable isotope ratio
AI 理解论文
溯源树
样例
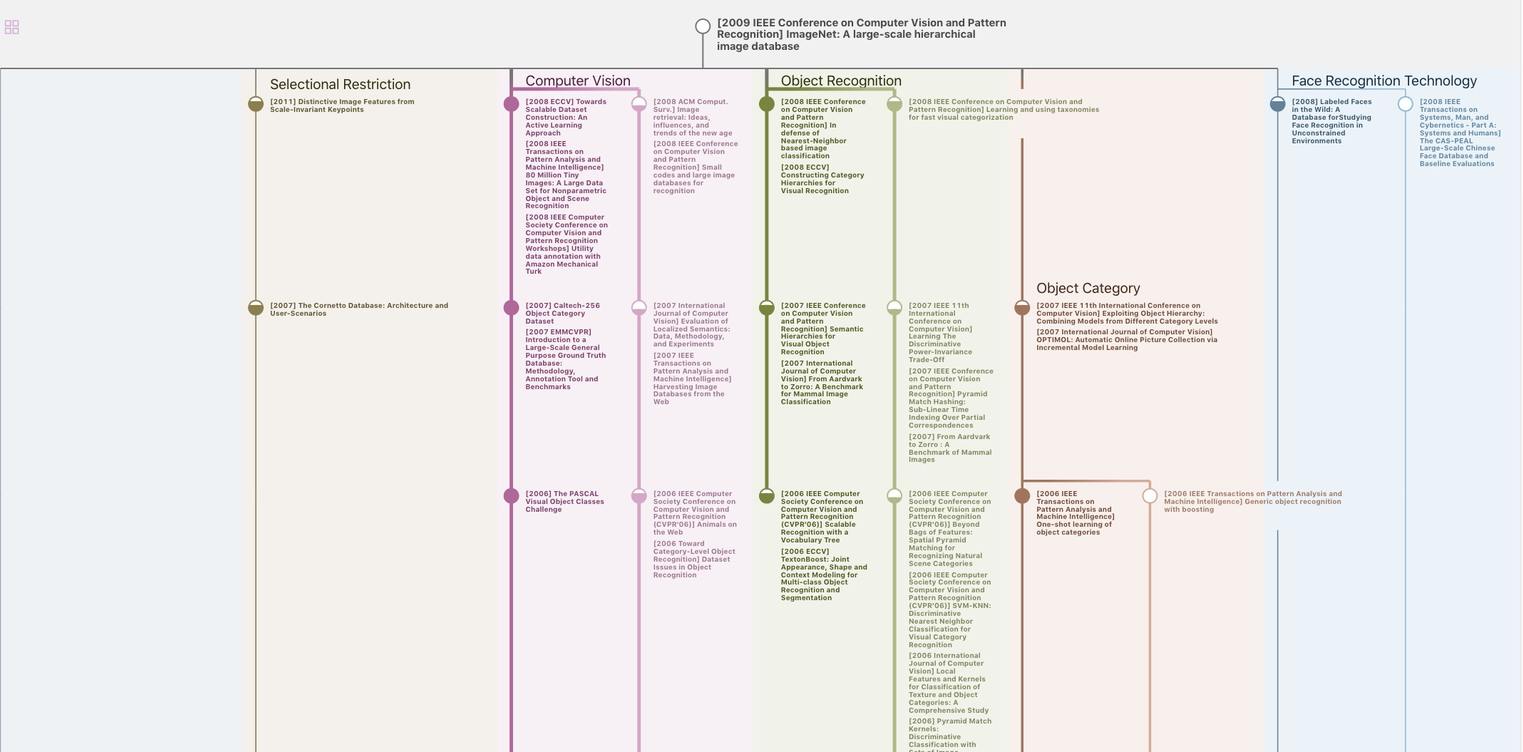
生成溯源树,研究论文发展脉络
Chat Paper
正在生成论文摘要