Data-driven integral sliding mode control based on disturbance decoupling technology for electric multiple unit
Journal of the Franklin Institute(2023)
摘要
The controller with high reliability and excellent tracking effect is essential for electric multiple unit (EMU) operation system. Nevertheless, similar to many complex systems, the EMU operation process is multivariable, strong coupled, nonlinear and time-varying. In particular, the coupling relationship between the variables in the system seriously affects the performance of the control system. Disturbance decoupling technology is an effective method to address the above problem, so this paper proposes a multi-input-multi-output (MIMO) data-driven integral sliding mode decoupling control (DISMDC) algorithm for the automatic train operation (ATO) system. Based on dynamic linearization (DL) technology, DISMDC equates the EMU operation process with a full format dynamic linearization (FFDL) data model, and uses FFDL data model to design a discrete integral sliding mode control (SMC) law. Meanwhile, the discrete extended state observer (DESO) is introduced to estimate the unmodeled dynamics, unknown disturbances and coupling between variables of the FFDL data model in real time, which further improves the equivalent description of the system and enhances the decoupling effect and control performance. The DISMDC method is tested on a semiphysical simulation platform of CRH380A EMU equipped in the laboratory, and compared with some traditional model-based and data-driven methods. The simulation results show that the control performance of DISMDC algorithm is better than some traditional methods. The velocity tracking error of each power unit of the EMU is within ±0.15 km/h, the control force and acceleration are within [−51 kN, 42 kN] and [−0.8532 m/s2, 0.7810 m/s2], respectively, and the variations are stable. The decoupling control of EMU under disturbance is realized.
更多查看译文
关键词
mode control,disturbance,data-driven
AI 理解论文
溯源树
样例
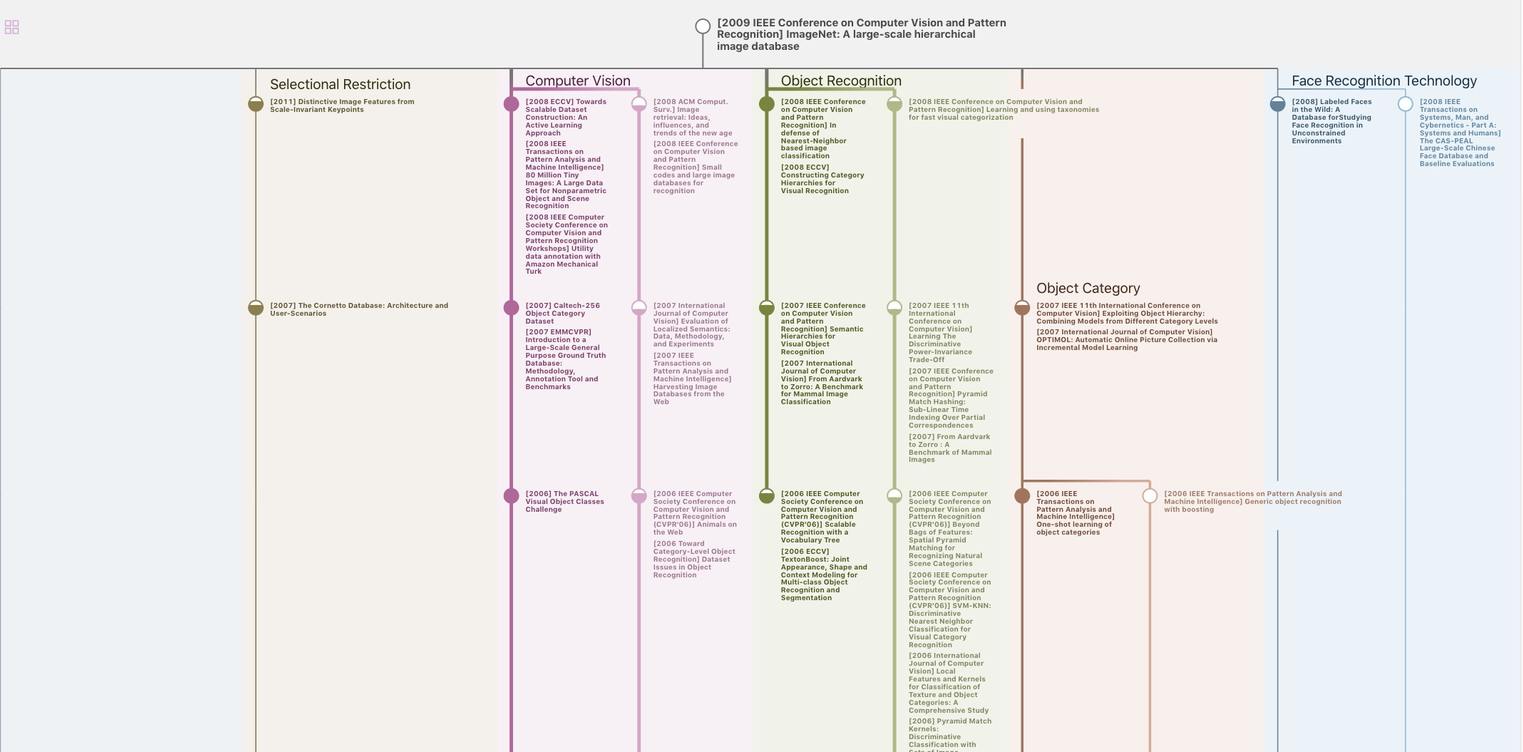
生成溯源树,研究论文发展脉络
Chat Paper
正在生成论文摘要