Multi-graph multi-label learning with novel and missing labels
Knowledge-Based Systems(2023)
摘要
Real-life objects typically contain complex structures, and the graph is a prevalent presentation for describing such objects. Multi-graph multi-label (MGML) learning is a supervised learning framework for learning complicated objects associated with multiple labels, where each object is described as a bag-of-graphs representation. However, existing methods are dependent on transforming graphs into instances, which prevents the structure information from being fully utilized; in addition, the adaptability of MGML is insufficient for the assumptions that the label set is in a closed environment and complete. In real applications, some novel labels may exist outside the fixed set, and some values of labels are missing. Existing MGML approaches are unable to deal with these novel and missing labels. In this paper, we propose a new multi-graph multi-label learning with novel and missing labels (MGMLNM) algorithm, which is the first attempt to learn novel and missing labels in the MGML problem. To preserve the structure information, we exploit the specific graph kernels to generate an efficient graph representation. To learn multi-graph data with novel and missing labels, we propose a unified objective function, which has a two-perspective regularization term, a projection-similarity regularization term, and a bag-dependent regularization term, enabling novel and missing labels to be modeled simultaneously and exploring the complex relationship between bag and graph labels. Two first-order proximity optimization methods are then developed to solve the unified problem efficiently. A theoretical analysis of the generalization bound is provided for the unified objective. Experimental results demonstrate the effectiveness of the proposed algorithm.& COPY; 2023 Elsevier B.V. All rights reserved.
更多查看译文
关键词
Multi-graph learning,Multi-label learning,Novel labels,Missing labels
AI 理解论文
溯源树
样例
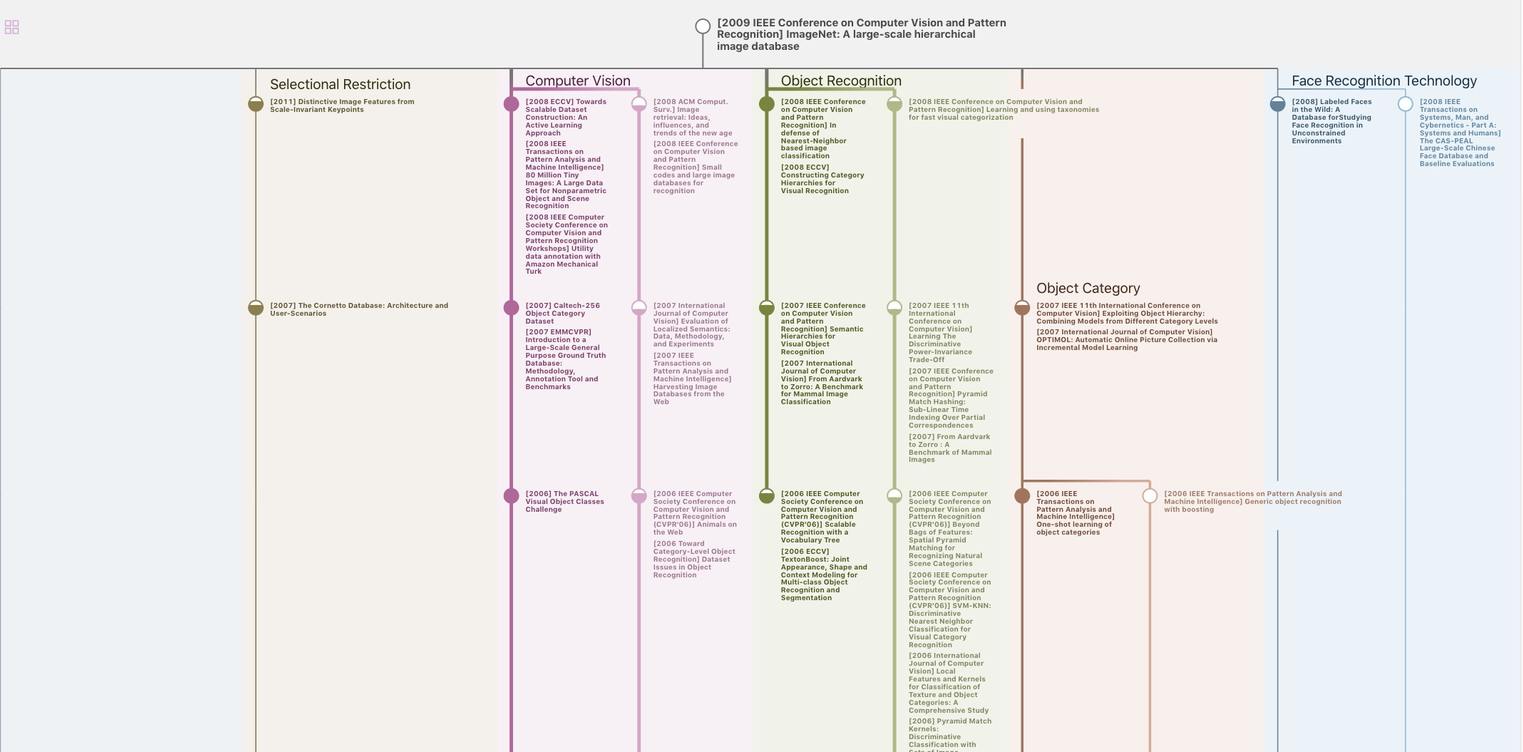
生成溯源树,研究论文发展脉络
Chat Paper
正在生成论文摘要