Asphalt pavement water film thickness detection and prediction model: A review
Journal of Traffic and Transportation Engineering (English Edition)(2023)
摘要
Over the course of storm or rainfall event, water thickness builds up on road surface resulting in a loss of contact between vehicle tires and road surface and puts drivers into immediate danger especially at high speeds. Therefore this is a considerably dangerous condition of the road and the realistic measurements and prediction model of water film thickness (WFT) on pavement surface is crucial for determining the road friction coefficient and evaluating the impact of rainfall on traffic safety. A review of the principle as well as critical evaluation of current detection methods of pavement WFT were compared for consistency and accuracy in this paper. The method selection guidelines are given for different road surface water film thickness detection requirements. This paper also introduces the latest development of WFT detection and prediction models for asphalt pavement, and gives the calculation elements and conditions of different WFT prediction models from different modeling ideas, which provides a basis for the selection and optimization of WFT models for future researchers. This article also suggests a few insights as further research directions on this topic. (1) The research can consider the influencing factors of WFT to conduct research on the delineation standard of pavement WFT. (2) In order to meet the future traffic safety dynamic early warning needs, road factors of different material types, disease conditions and linear conditions should be studied, as well as a comprehensive and accurate real-time water film thickness detection and evaluation method considering meteorological factors of rainfall timing, scale and intensity. (3) The prediction model of WFT should be further studied by the analytical method to clarify the influence of the pavement WFT on the driving safety.
更多查看译文
关键词
Asphalt pavement,Water film thickness,Detection method,Prediction model
AI 理解论文
溯源树
样例
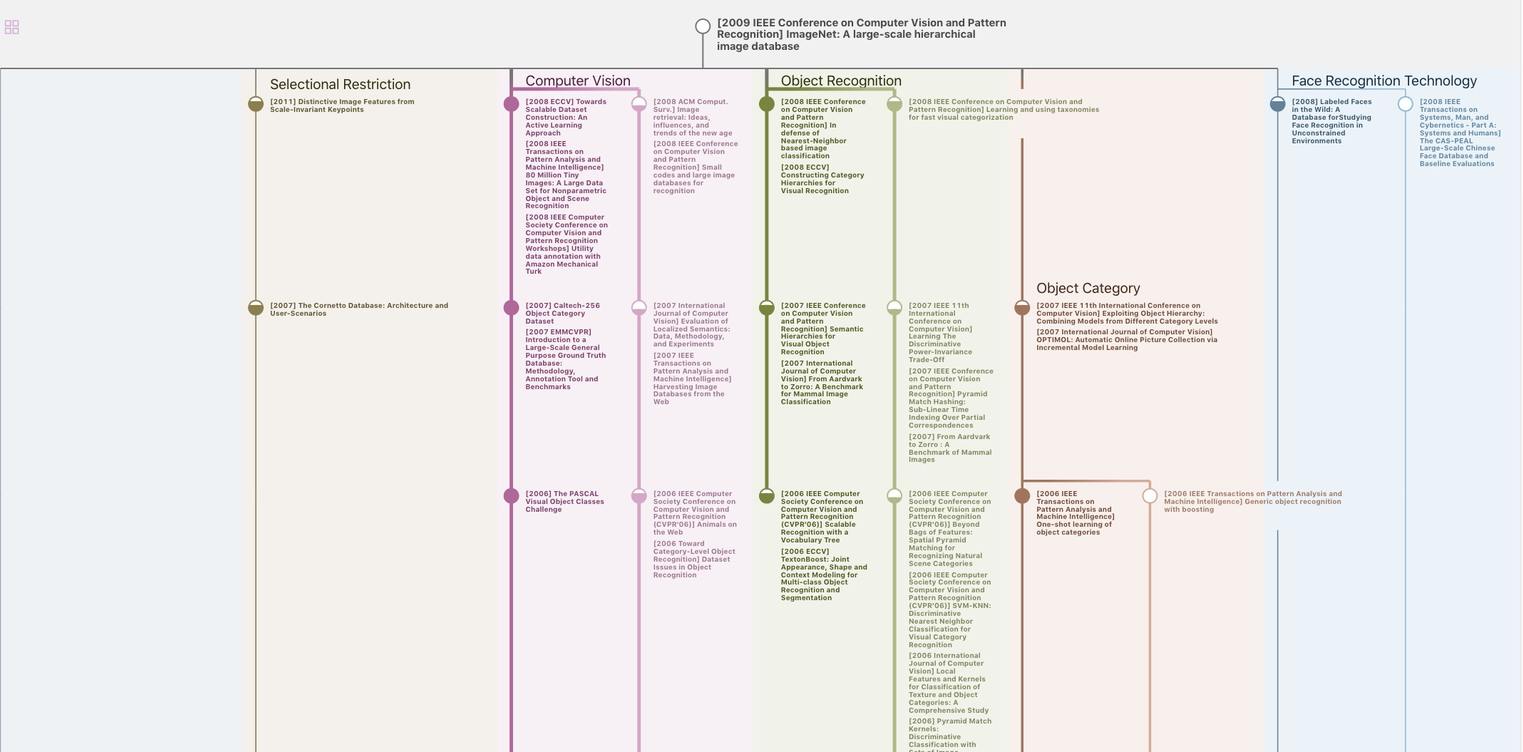
生成溯源树,研究论文发展脉络
Chat Paper
正在生成论文摘要