Complexity-based drift detection for nonstationary data streams
Neurocomputing(2023)
摘要
This publication presents the Complexity Drift Detector (C2D) – the method for detecting a concept shift in the data stream based on the classification task complexity measures. The method belongs to the group of detectors agnostic to the recognition quality of the base classifier. The possibility of selecting a set of difficulty measures taken into account during the data stream processing allows applying the method to many tasks in which the detection of a classification task complexity change is expected. The publication includes experiments analyzing the hyperparameters’ influence on the operation of the method and a broad comparative experiment comparing the proposed algorithm with state-of-the-art solutions. The experiments were carried out on synthetic data streams of different dimensions and with different concept drift characteristics, also presenting the effects of processing real-world data streams. The results of the conducted research confirm the high efficiency of the method in detecting concept changes, sensitive not only to the fact of drift occurrence but also to its dynamics.
更多查看译文
关键词
drift detection,complexity-based
AI 理解论文
溯源树
样例
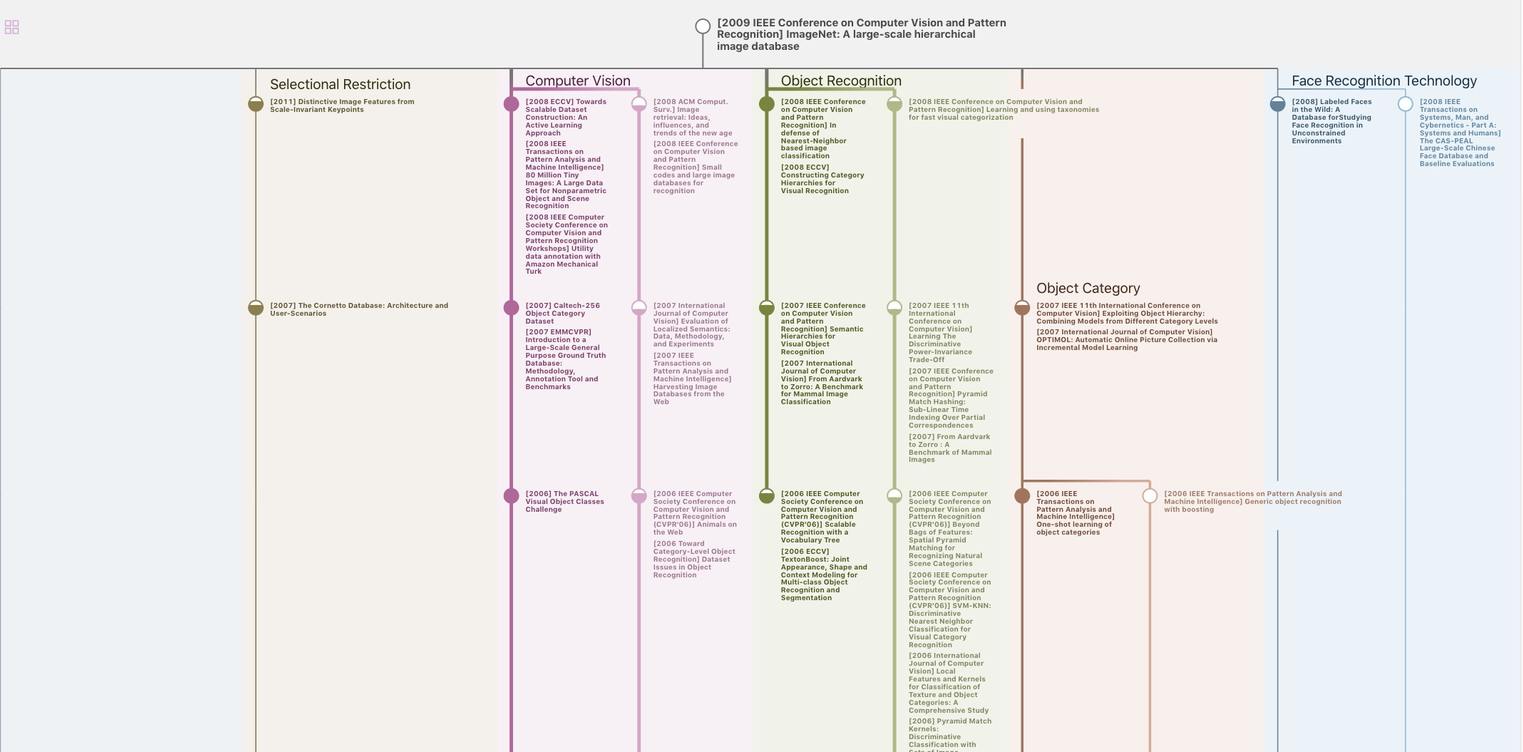
生成溯源树,研究论文发展脉络
Chat Paper
正在生成论文摘要