Prediction of SIF range for plain API 5L Grade X65 steel under corrosion using AI & ML models
Materials Today Communications(2023)
摘要
Corrosion is one of the significant aspects of science & engineering and the effects of corrosion on various infrastructures due to fatigue loading are paramount. Experimental investigations to evaluate fracture parameters and fatigue crack growth life take a considerable amount of time and effort. Recently, the computational boon in the form of Artificial Intelligence (AI) and Machine Learning (ML) has paved the way for predicting the required fracture parameters through training with the available limited experimental data. In this paper, the fracture parameter, Stress Intensity Factor (SIF) of plain API 5L X65 grade steel is predicted using the experimental data of crack growth studies performed on the specimen with single edge notch, eccentrically loaded, subjected to inert and corrosive environments (2.0% and 3.5% NaCl). From the experimental data, the fracture parameter, SIF range is determined for various crack lengths corresponding to certain loading cycles. Multivariate Adaptive regression splines (MARS), Artificial Neural Network (ANN), and relevance vector machine (RVM) based models are developed by using the experimental data to predict the SIF range. The efficiencies of the developed models are verified through several statistical parameters. The studies show that the proposed models are capable of predicting the SIF range for plain API 5L X65 grade steel effectively with the available experimental data. The predicted SIF range is highly useful for the prediction of crack growth life and the evaluation of the residual strength of structural components.
更多查看译文
关键词
sif range,corrosion,steel
AI 理解论文
溯源树
样例
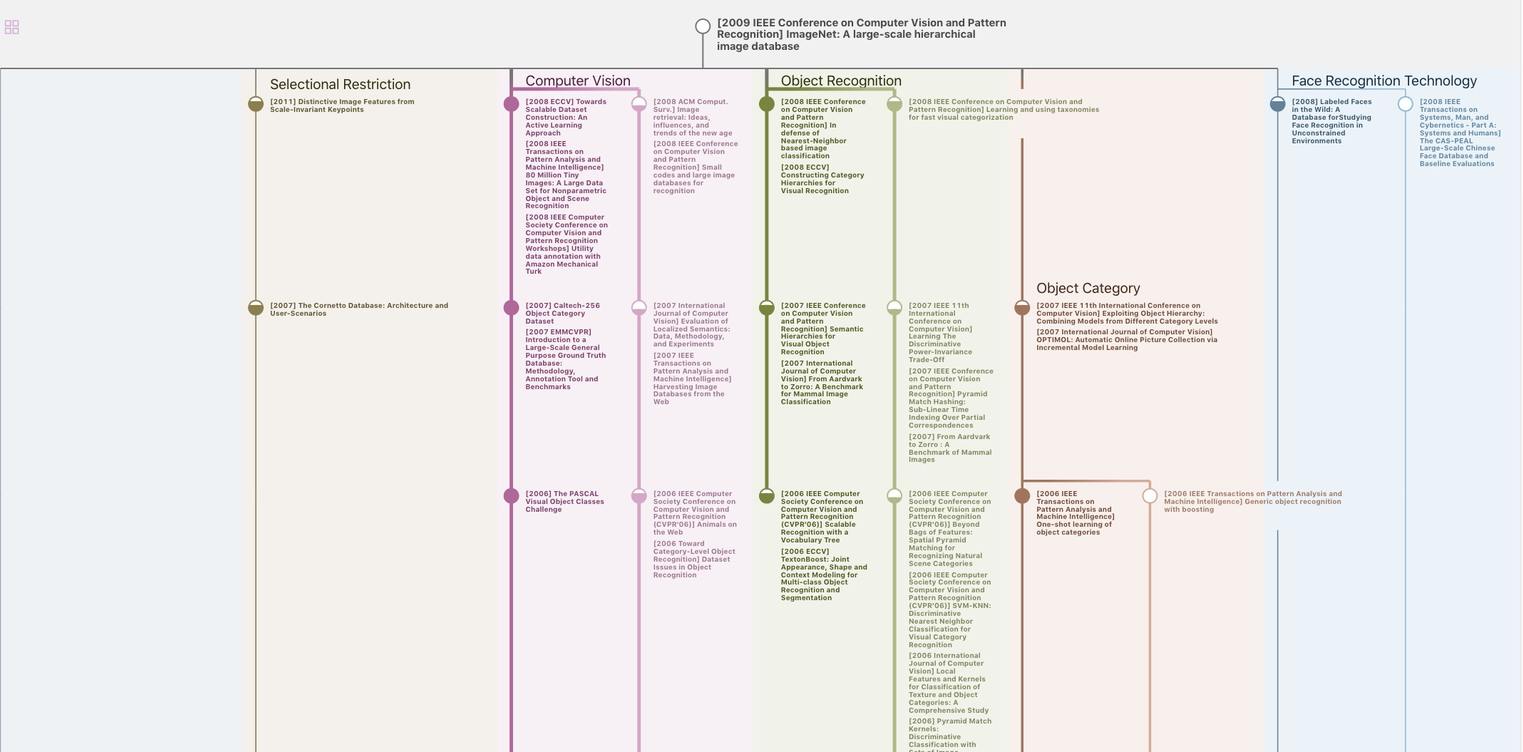
生成溯源树,研究论文发展脉络
Chat Paper
正在生成论文摘要