Strength evaluation sustainable concrete with waste ingredients at elevated temperature by employing interpretable algorithms: Optimization and hyper tuning
Materials Today Communications(2023)
摘要
Mix proportions of the component materials employed significantly affects the concrete's compressive strength (CS) at elevated temperatures. Predicting the CS of concrete under elevated temperatures is complicated and requires the application of reliable and effective algorithms. In the present study, machine learning methods using distinct learners and combined learners, such as bagging and boosting, are used to estimate the CS of concrete at elevated temperatures. This is achieved by the utilization of Anaconda software using Python. The combined learner boosting (Adaboost and Xgboost), bagging, and modified bagging algorithms are utilized in constructing a robust ensemble learner by integrating an individual learner. These ensemble algorithms were incorporated with decision tree (DT), support vector regression (SVR), and multilayer perception neural network (MLPNN) techniques to enhance the robustness of the models. The database used to develop the models comprised 207 data points with nine input parameters: water, temperature, silica fume, cement, fly ash, superplasticizer, sand, Nano silica, and gravels and CS as output. The optimization was performed to achieve the maximum R2 by training the data in 20 sub-models for each boosting and bagging technique. The data is verified by using a K-fold cross-validation approach. In addition, various statistical measures such as MAE, RMSE, NSE, and MSE are employed for cross-validation of the data. Based on the findings, it appears that making use of bagging and boosting learners results in improved particular model performance. In more general terms, RF and DT with bagging produce the most accurate results among the developed models, with a higher R2 value of 0.94 and lesser error values. When it comes to machine learning, having an ensemble model would often improve the model's overall accuracy.
更多查看译文
关键词
sustainable concrete,optimization,interpretable algorithms,waste ingredients
AI 理解论文
溯源树
样例
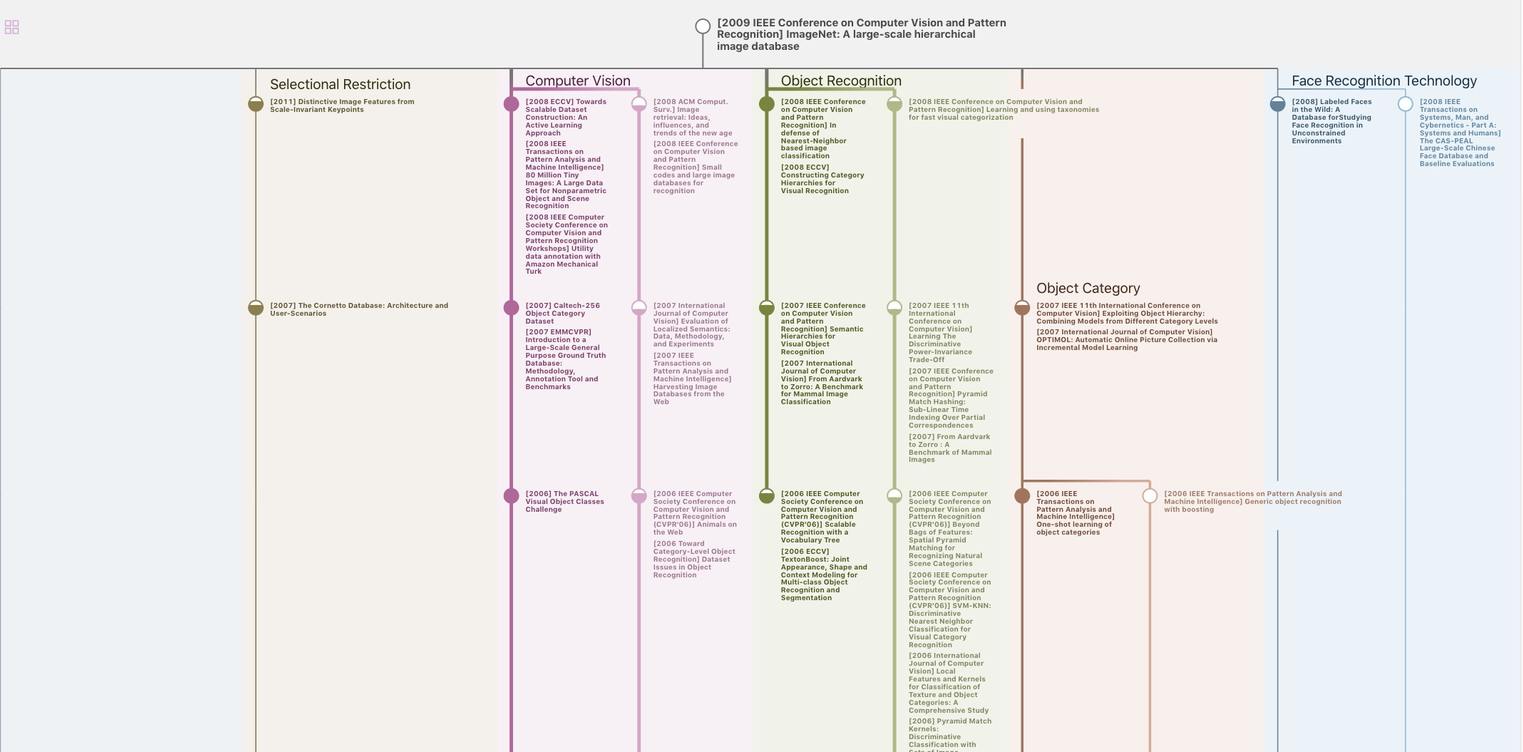
生成溯源树,研究论文发展脉络
Chat Paper
正在生成论文摘要