Deep learning-based asteroid surface temperature evaluation from disk-resolved near-infrared spectra for thermal excess correction
Planetary and Space Science(2023)
摘要
Near-Earth asteroids can become warm enough to emit radiation at near-infrared wavelengths, close to 2.5 μm. Thermal radiation can interfere with reflectance measurements in these wavelengths, and should be evaluated and corrected for. Current methods for correcting disk-resolved measurements either rely on previous Earth-based observations or perform heavy computations to find the thermally emitted spectral radiance. Using results based on disk-integrated observations may lead to errors for some cases where the target asteroid surface is not homogeneous. Computational efficiency is desirable for those future missions where data processing is to be performed on-board the spacecraft due to a limited downlink budget, such as missions employing small spacecraft. We propose to predict the temperature of an asteroid surface element from its observed spectral radiance using a convolutional neural network. The thermal spectral radiance emitted by the asteroid surface can be approximated using the temperature, and subsequently subtracted from the original spectral radiance. The model was tested using OSIRIS-REx measurements of asteroid (101955) Bennu with promising results. The performance of the model should be validated further in the future as asteroid missions produce suitable data. Both accuracy and speed of the method could likely be increased significantly with further development.
更多查看译文
关键词
asteroid surface temperature evaluation,thermal,learning-based,disk-resolved,near-infrared
AI 理解论文
溯源树
样例
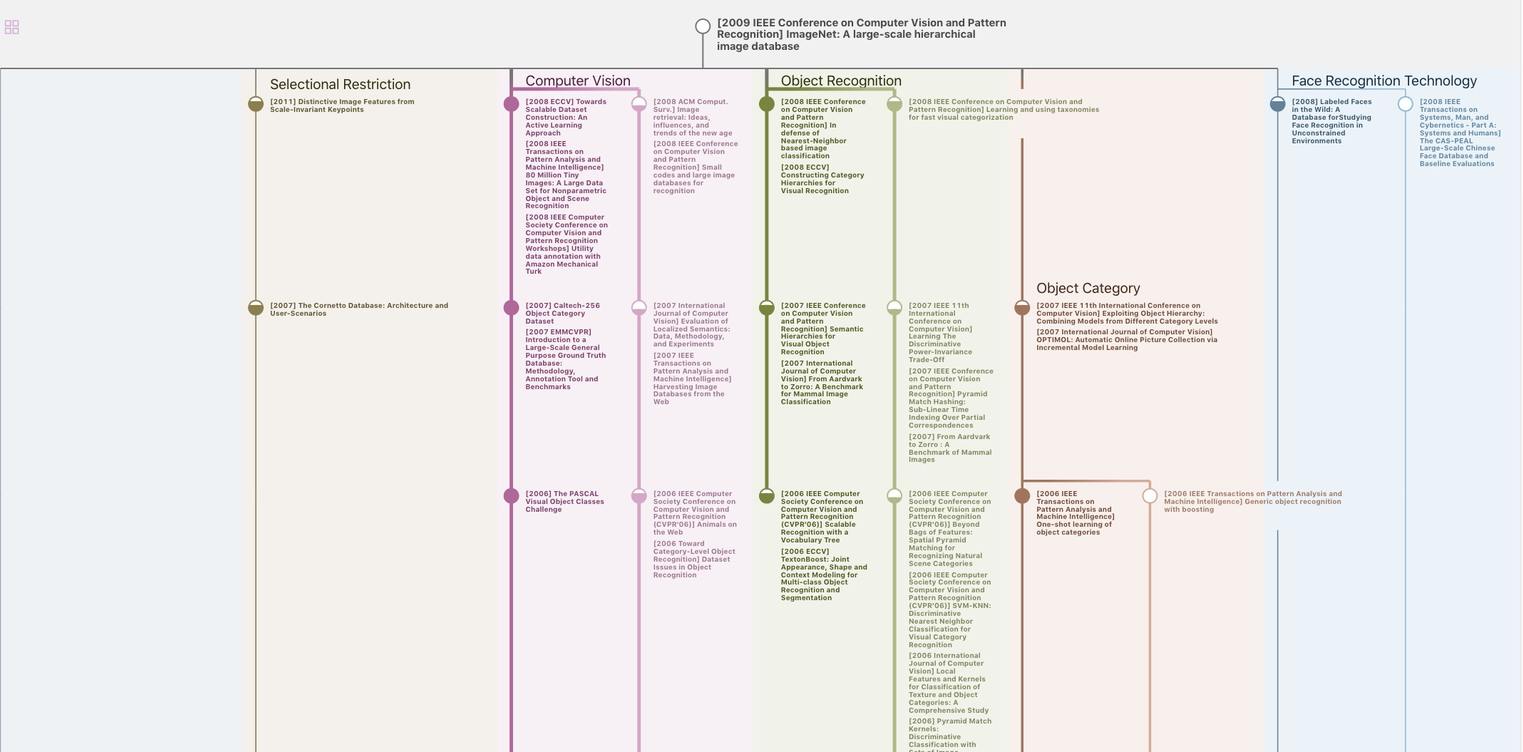
生成溯源树,研究论文发展脉络
Chat Paper
正在生成论文摘要