Implicit multi-sensor reconstruction based on neural signed distance functions for reverse engineering
Procedia CIRP(2023)
摘要
Reverse engineering has been gaining considerable interest in the product design process, in which the obtaining of measurement data from digitizing sensors are important beginning procedures. Considering the requirements of both accuracy and efficiency, multi-sensor measurement and reconstruction emerge as promising techniques to take advantage of the complementary capabilities of the different sensors. However, most exiting multi-sensor reconstruction methods are only applicable to explicit surfaces, which are defined by parametric equations with two parameters. The limitation of explicit representation significantly restricts the further application of multi-sensors reconstruction for more complex products. This paper proposes an implicit multi-sensor reconstruction method, in which the surface is represented implicitly by a deep neural network controlled by the signed distance function. Therefore, the multi-sensor reconstruction problem is then transferred to a multi-sources neural network training problem, which can be addressed by parameters frozen and finetuning techniques from the transfer learning field. The reconstruction experiments on two real workpieces show the effectiveness of the proposed method, and the limitations of neural network representation are also discussed.
更多查看译文
关键词
distance functions,reconstruction,multi-sensor
AI 理解论文
溯源树
样例
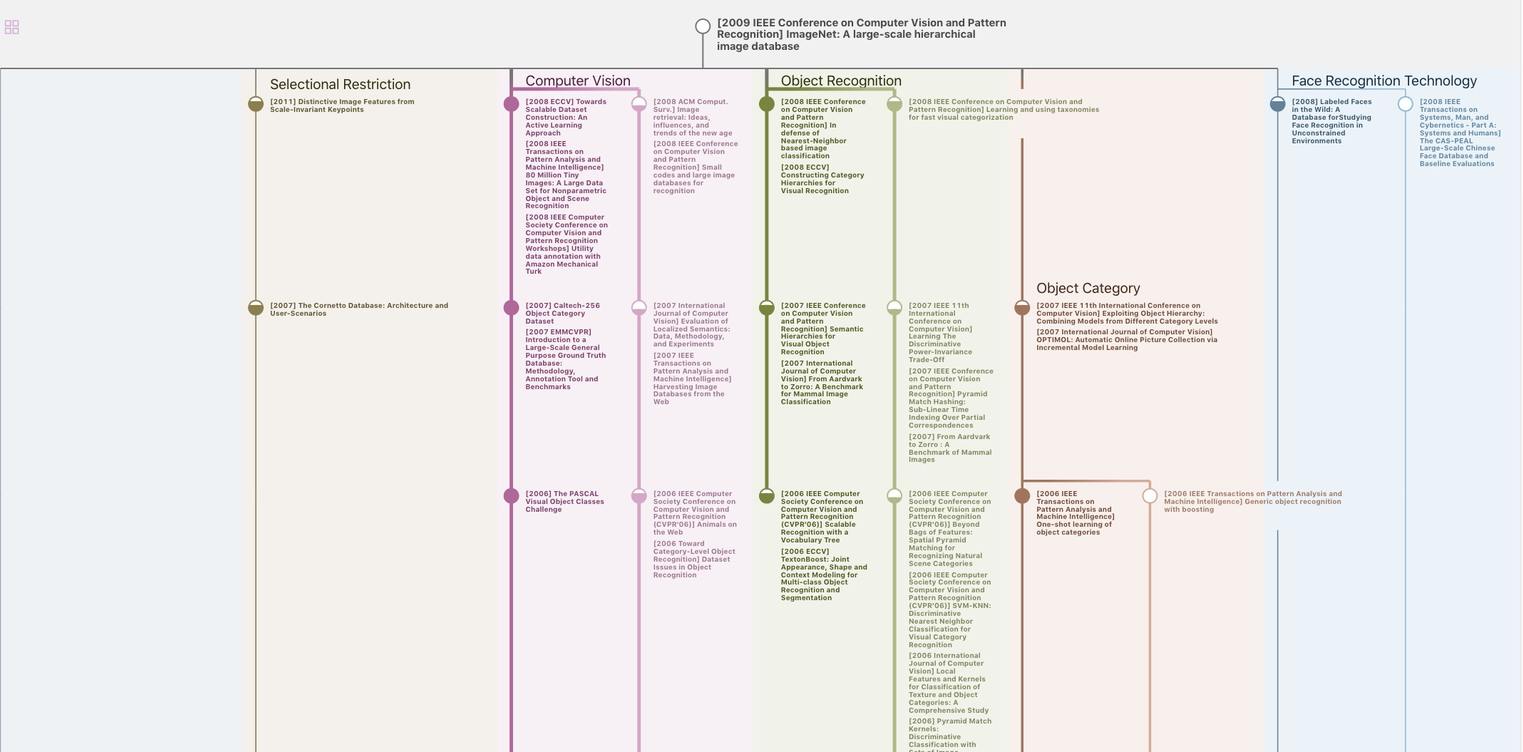
生成溯源树,研究论文发展脉络
Chat Paper
正在生成论文摘要