Likelihood Analysis of Imperfect Data
IEEE Transactions on Systems, Man, and Cybernetics: Systems(2023)
摘要
This article investigates how to make use of imperfect data gathered from different sources for inference and decision making. Based on Bayesian inference and the principle of likelihood, a likelihood analysis method is proposed for acquisition of evidence from imperfect data to enable likelihood inference within the framework of the evidential reasoning (ER). The nature of this inference process is underpinned by the new necessary and sufficient conditions that when a piece of evidence is acquired from a data source it should be represented as a normalized likelihood distribution to capture the essential evidential meanings of data. While the explanation of sufficiency of the conditions is straightforward based on the principle of likelihood, their necessity needs to be established by following the principle of Bayesian inference. It is also revealed that the inference process enabled by the ER rule under the new conditions constitutes a likelihood inference process, which becomes equivalent to Bayesian inference when there is no ambiguity in data and a prior distribution can be obtained as a piece of independent evidence. Two examples in decision analysis under uncertainty and a case study about fault diagnosis for railway track maintenance management are examined to demonstrate the steps of implementation and potential applications of the likelihood inference process.
更多查看译文
关键词
Bayesian inference, decision making under uncertainty, evidential reasoning (ER), likelihood analysis of data, likelihood principle
AI 理解论文
溯源树
样例
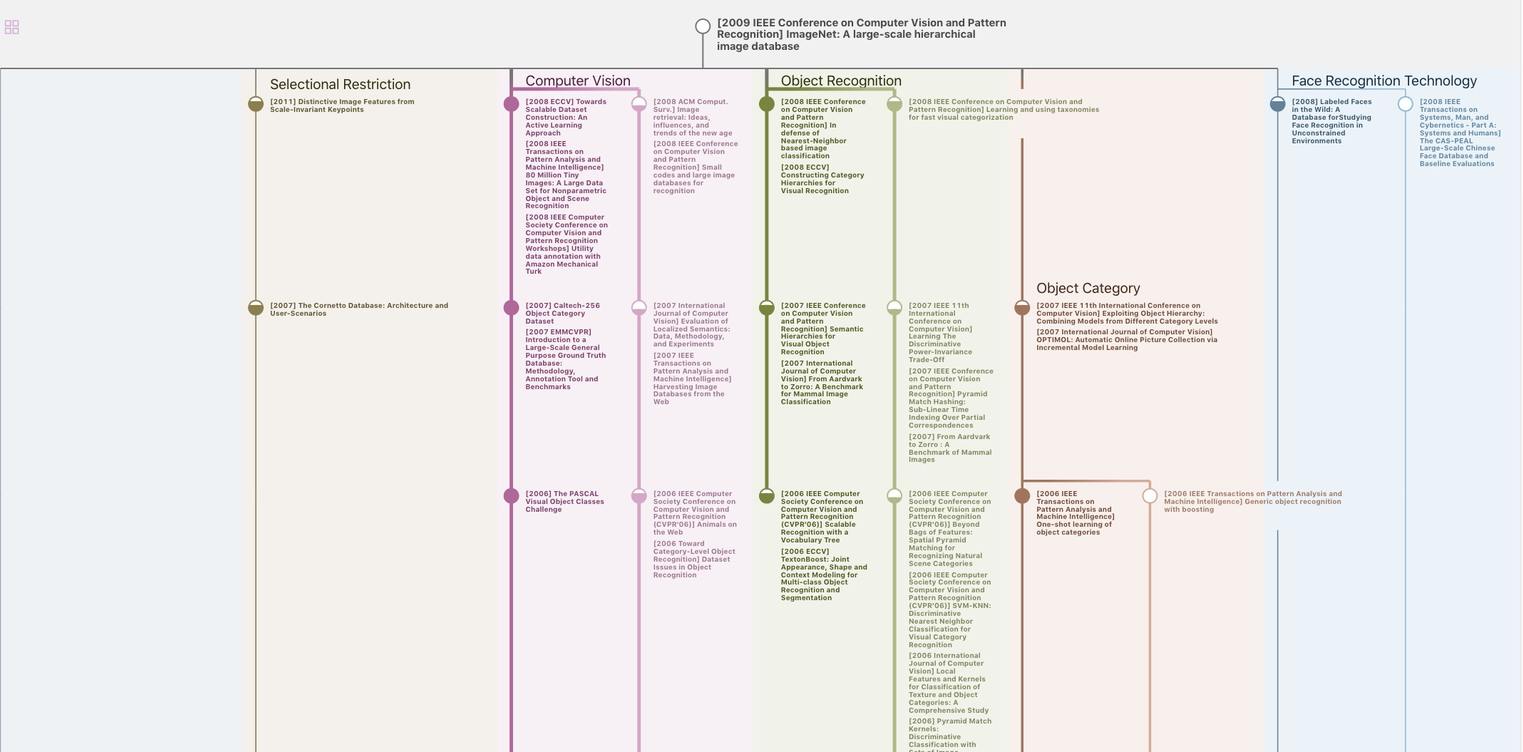
生成溯源树,研究论文发展脉络
Chat Paper
正在生成论文摘要