Model-free daily inversion of NOx emissions using TROPOMI (MCMFE-NOx) and its uncertainty: Declining regulated emissions and growth of new sources
Remote Sensing of Environment(2023)
摘要
Current approaches to estimate NOx emissions fail to account for new and small sources, biomass burning, and sources which change rapidly in time, generally don't account for measurement error, and are either based on models, or do not consider wind, chemistry, and dynamical effects. This work introduces a new, model-free analytical environment that assimilates daily TROPOMI NO2 measurements in a mass-conserving manner, to invert daily NOx emissions. This is applied over a rapidly developing and energy-consuming region of Northwest China, specifically chosen due to substantial economic and population changes, new environmental policies, large use of coal, and access to independent emissions measurements for validation, making this region representative of many rapidly developing regions found across the Global South. This technique computes a net NOx emissions gain of 118% distributed in a see-saw manner: a more than doubling of emissions in cleaner regions, chemical plants, and regions thought to be emissions-free, combined with a more than halving of emissions in city centers and at well-regulated steel and powerplants. The results allow attribution of sources, with major contributing factors computed to be increased combustion temperature, atmospheric transport, and in-situ chemical processing. Furthermore, sensitivity runs that represent the range of uncertainties in the TROPOMI NO2 retrievals themselves are performed, and it is determined that the differences between the errors in TROPOMI NO2 lead to smaller errors in the emissions estimates, due to non-linear buffering built in from the physical bounds on the underlying physical and chemical driving factors. It is also observed that errors in the priori dataset contribute more than the uncertainties in the TROPOMI retrievals, opening a new door to recursive iteration between this approach and new emissions dataset development. It is hoped that these findings will drive a new look at emissions estimation and how it is related to remotely sensed measurements and associated uncertainties, especially applied to rapidly developing regions. This is especially important for understanding the loadings and impacts of short-lived climate forcers, and provides a bridge between remotely sensed data, measurement error, and models, while allowing for further improvement of identification of new, small, and rapidly changing sources.
更多查看译文
关键词
nox emissions,model-free,mcmfe-nox
AI 理解论文
溯源树
样例
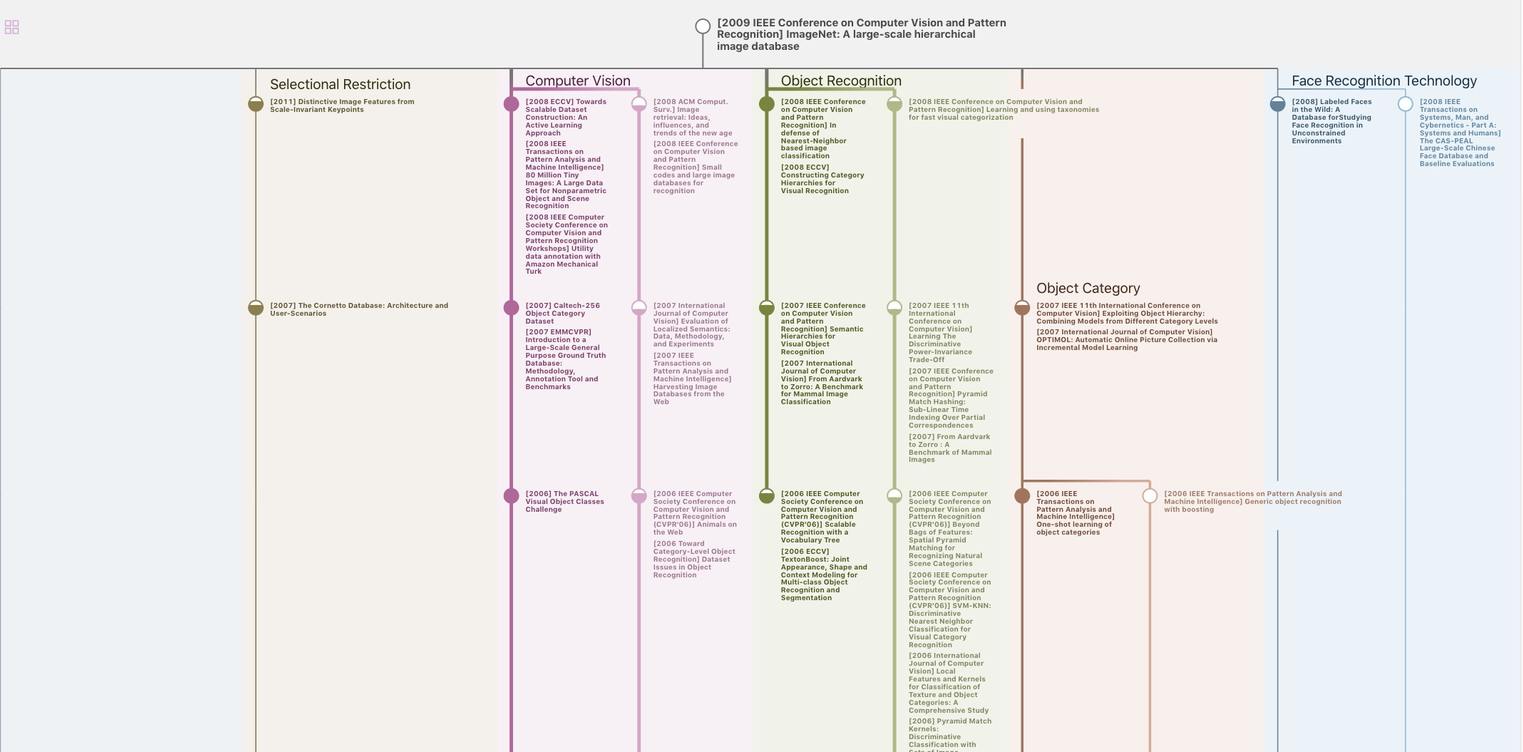
生成溯源树,研究论文发展脉络
Chat Paper
正在生成论文摘要