Wildland fire mid-story: A generative modeling approach for representative fuels
ENVIRONMENTAL MODELLING & SOFTWARE(2024)
摘要
Computational models for understanding and predicting fire in wildland and managed lands are increasing in impact. Data characterizing the fuels and environment is needed to continue improvement in the fidelity and reliability of fire outcomes. This paper addresses a gap in the characterization and population of mid -story fuels, which are not easily observable either through traditional survey, where data collection is time consuming, or with remote sensing, where the mid-story is typically obscured by forest canopy. We present a methodology to address populating a mid-story using a generative model for fuel placement that captures key concepts of spatial density and heterogeneity that varies by regional or local environmental conditions. The advantage of using a parameterized generative model is the ability to calibrate (or 'tune') the generated fuels based on comparison to limited observation datasets or with expert guidance, and we show how this generative model can balance information from these sources to capture the essential characteristics of the wildland fuels environment. In this paper we emphasize the connection of terrestrial LiDAR (TLS) as the observations used to calibrate of the generative model, as TLS is a promising method for supporting forest fuels assessment. Code for the methods in this paper is available.
更多查看译文
关键词
Wildfire modeling,Cox process,Bayesian model calibration,Gaussian process,Prescribed fire,Environmental assessment,Spatial generative model
AI 理解论文
溯源树
样例
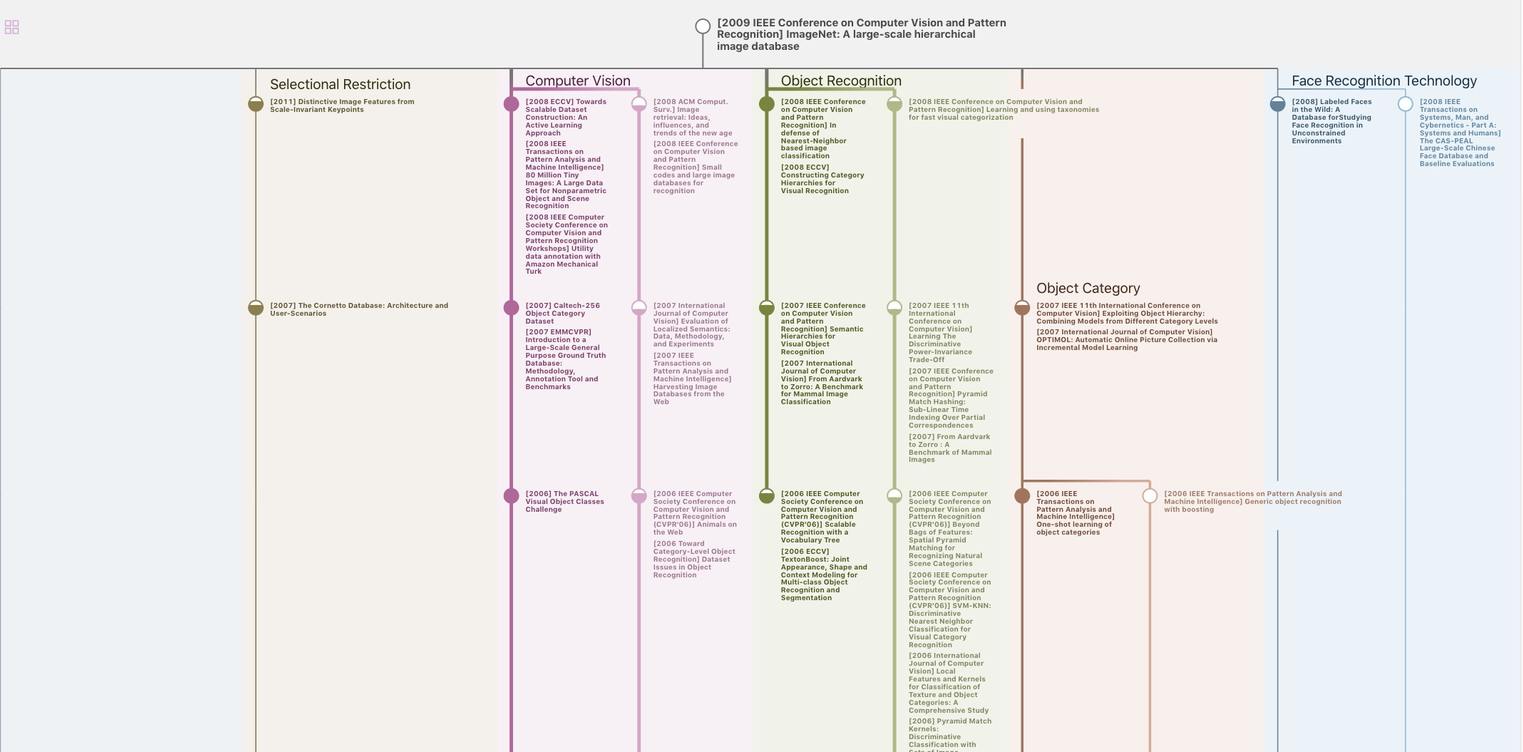
生成溯源树,研究论文发展脉络
Chat Paper
正在生成论文摘要